کد مقاله | کد نشریه | سال انتشار | مقاله انگلیسی | نسخه تمام متن |
---|---|---|---|---|
155326 | 456891 | 2013 | 14 صفحه PDF | دانلود رایگان |
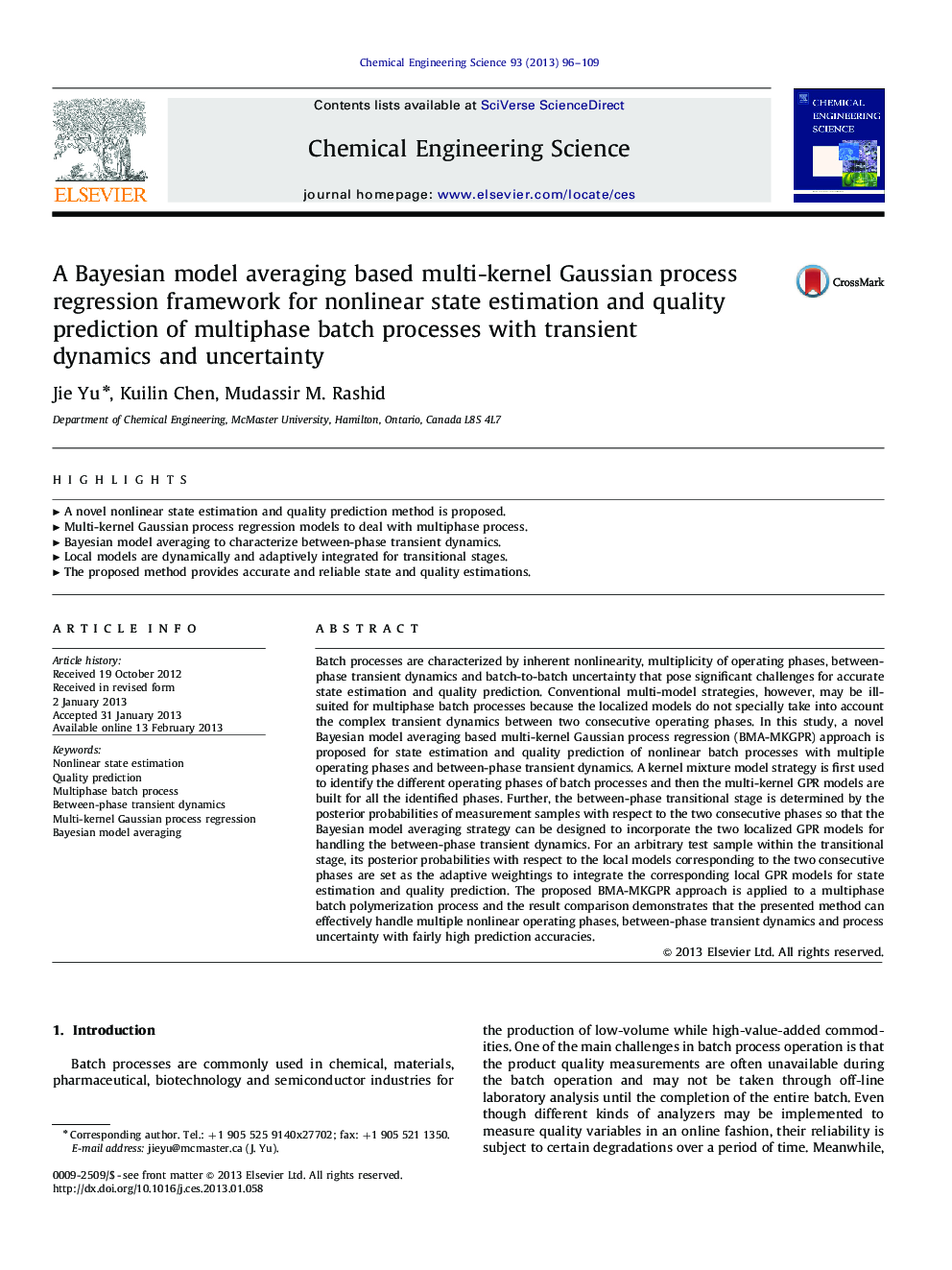
Batch processes are characterized by inherent nonlinearity, multiplicity of operating phases, between-phase transient dynamics and batch-to-batch uncertainty that pose significant challenges for accurate state estimation and quality prediction. Conventional multi-model strategies, however, may be ill-suited for multiphase batch processes because the localized models do not specially take into account the complex transient dynamics between two consecutive operating phases. In this study, a novel Bayesian model averaging based multi-kernel Gaussian process regression (BMA-MKGPR) approach is proposed for state estimation and quality prediction of nonlinear batch processes with multiple operating phases and between-phase transient dynamics. A kernel mixture model strategy is first used to identify the different operating phases of batch processes and then the multi-kernel GPR models are built for all the identified phases. Further, the between-phase transitional stage is determined by the posterior probabilities of measurement samples with respect to the two consecutive phases so that the Bayesian model averaging strategy can be designed to incorporate the two localized GPR models for handling the between-phase transient dynamics. For an arbitrary test sample within the transitional stage, its posterior probabilities with respect to the local models corresponding to the two consecutive phases are set as the adaptive weightings to integrate the corresponding local GPR models for state estimation and quality prediction. The proposed BMA-MKGPR approach is applied to a multiphase batch polymerization process and the result comparison demonstrates that the presented method can effectively handle multiple nonlinear operating phases, between-phase transient dynamics and process uncertainty with fairly high prediction accuracies.
► A novel nonlinear state estimation and quality prediction method is proposed.
► Multi-kernel Gaussian process regression models to deal with multiphase process.
► Bayesian model averaging to characterize between-phase transient dynamics.
► Local models are dynamically and adaptively integrated for transitional stages.
► The proposed method provides accurate and reliable state and quality estimations.
Journal: Chemical Engineering Science - Volume 93, 19 April 2013, Pages 96–109