کد مقاله | کد نشریه | سال انتشار | مقاله انگلیسی | نسخه تمام متن |
---|---|---|---|---|
237413 | 465704 | 2012 | 7 صفحه PDF | دانلود رایگان |
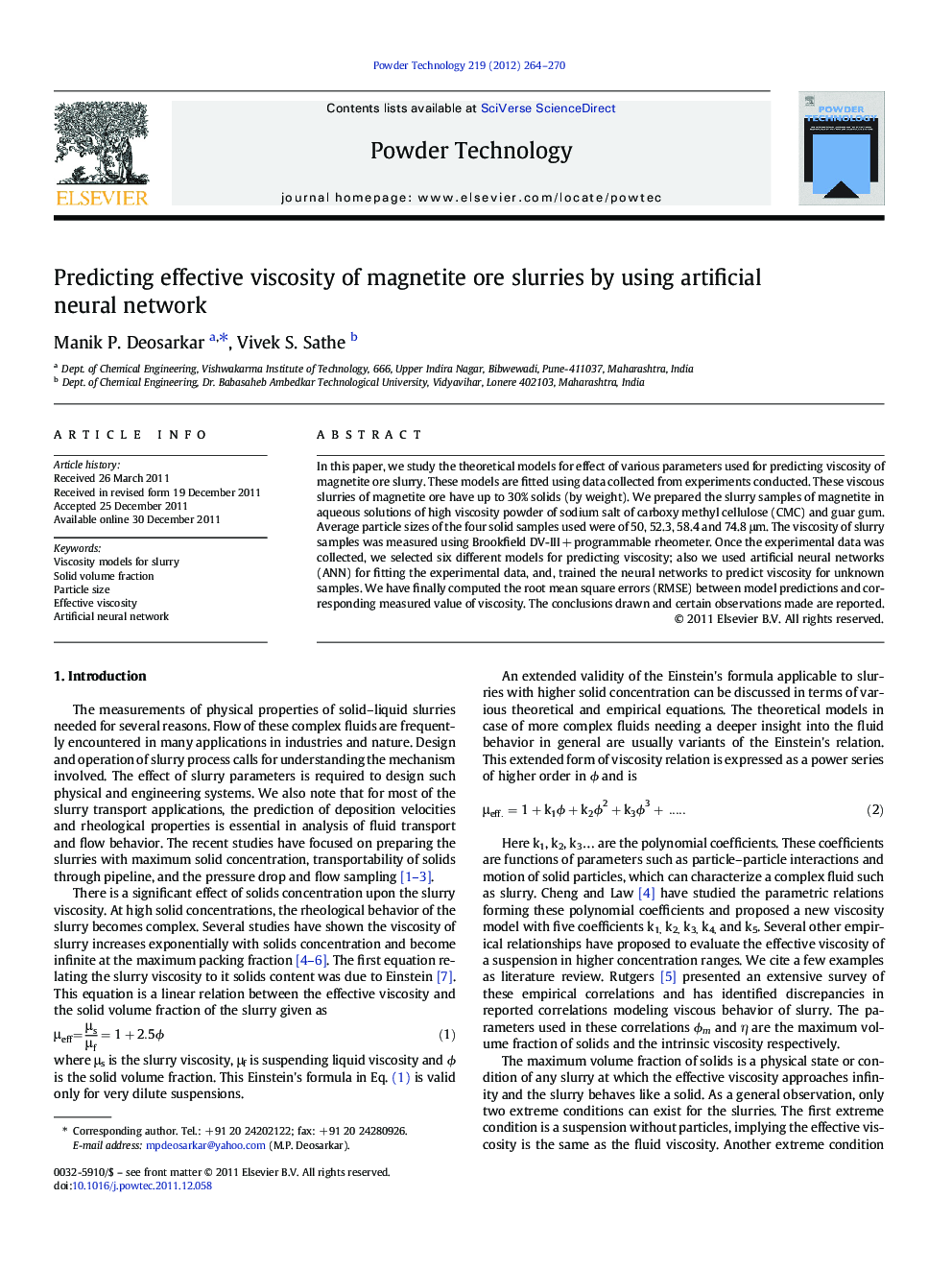
In this paper, we study the theoretical models for effect of various parameters used for predicting viscosity of magnetite ore slurry. These models are fitted using data collected from experiments conducted. These viscous slurries of magnetite ore have up to 30% solids (by weight). We prepared the slurry samples of magnetite in aqueous solutions of high viscosity powder of sodium salt of carboxy methyl cellulose (CMC) and guar gum. Average particle sizes of the four solid samples used were of 50, 52.3, 58.4 and 74.8 μm. The viscosity of slurry samples was measured using Brookfield DV-III + programmable rheometer. Once the experimental data was collected, we selected six different models for predicting viscosity; also we used artificial neural networks (ANN) for fitting the experimental data, and, trained the neural networks to predict viscosity for unknown samples. We have finally computed the root mean square errors (RMSE) between model predictions and corresponding measured value of viscosity. The conclusions drawn and certain observations made are reported.
Comparison between effective viscosity predictions by different models for the slurries prepared in aqueous guar gum solutions.Figure optionsDownload as PowerPoint slideHighlights
► Viscosity of magnetite ore slurries with up to 30% solids (by wt.) was measured.
► Six models are presented for predicting viscosity of magnetite ore slurries.
► Liu's model is better fitting among the six models presented.
► ANN model is developed for predicting viscosity of magnetite ore slurries.
► Results of Liu's model and ANN model are compared.
Journal: Powder Technology - Volume 219, March 2012, Pages 264–270