کد مقاله | کد نشریه | سال انتشار | مقاله انگلیسی | نسخه تمام متن |
---|---|---|---|---|
303742 | 512753 | 2011 | 9 صفحه PDF | دانلود رایگان |
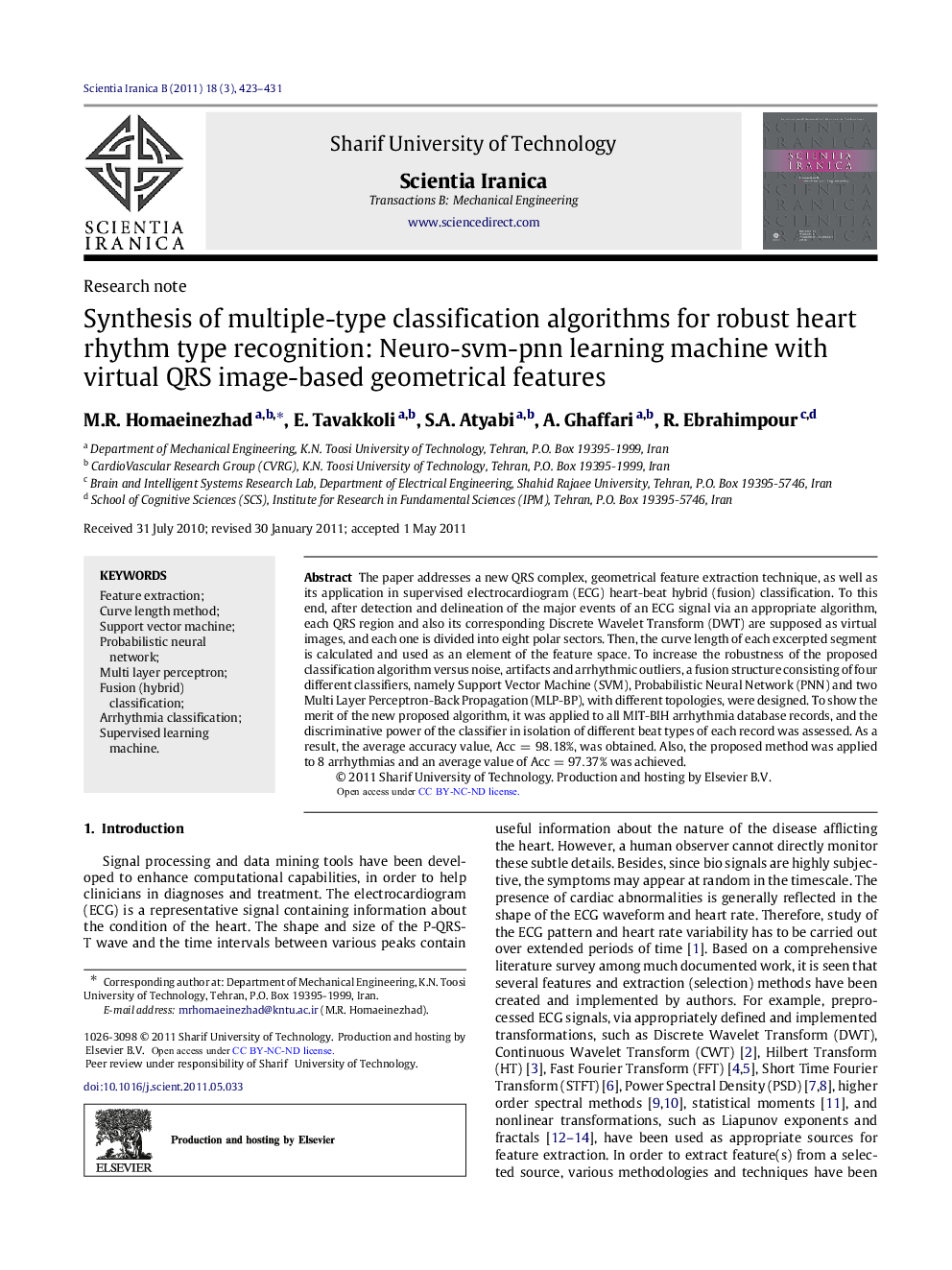
The paper addresses a new QRS complex, geometrical feature extraction technique, as well as its application in supervised electrocardiogram (ECG) heart-beat hybrid (fusion) classification. To this end, after detection and delineation of the major events of an ECG signal via an appropriate algorithm, each QRS region and also its corresponding Discrete Wavelet Transform (DWT) are supposed as virtual images, and each one is divided into eight polar sectors. Then, the curve length of each excerpted segment is calculated and used as an element of the feature space. To increase the robustness of the proposed classification algorithm versus noise, artifacts and arrhythmic outliers, a fusion structure consisting of four different classifiers, namely Support Vector Machine (SVM), Probabilistic Neural Network (PNN) and two Multi Layer Perceptron-Back Propagation (MLP-BP), with different topologies, were designed. To show the merit of the new proposed algorithm, it was applied to all MIT-BIH arrhythmia database records, and the discriminative power of the classifier in isolation of different beat types of each record was assessed. As a result, the average accuracy value, Acc=98.18%, was obtained. Also, the proposed method was applied to 8 arrhythmias and an average value of Acc=97.37% was achieved.
Journal: Scientia Iranica - Volume 18, Issue 3, June 2011, Pages 423–431