کد مقاله | کد نشریه | سال انتشار | مقاله انگلیسی | نسخه تمام متن |
---|---|---|---|---|
378661 | 659182 | 2006 | 18 صفحه PDF | دانلود رایگان |
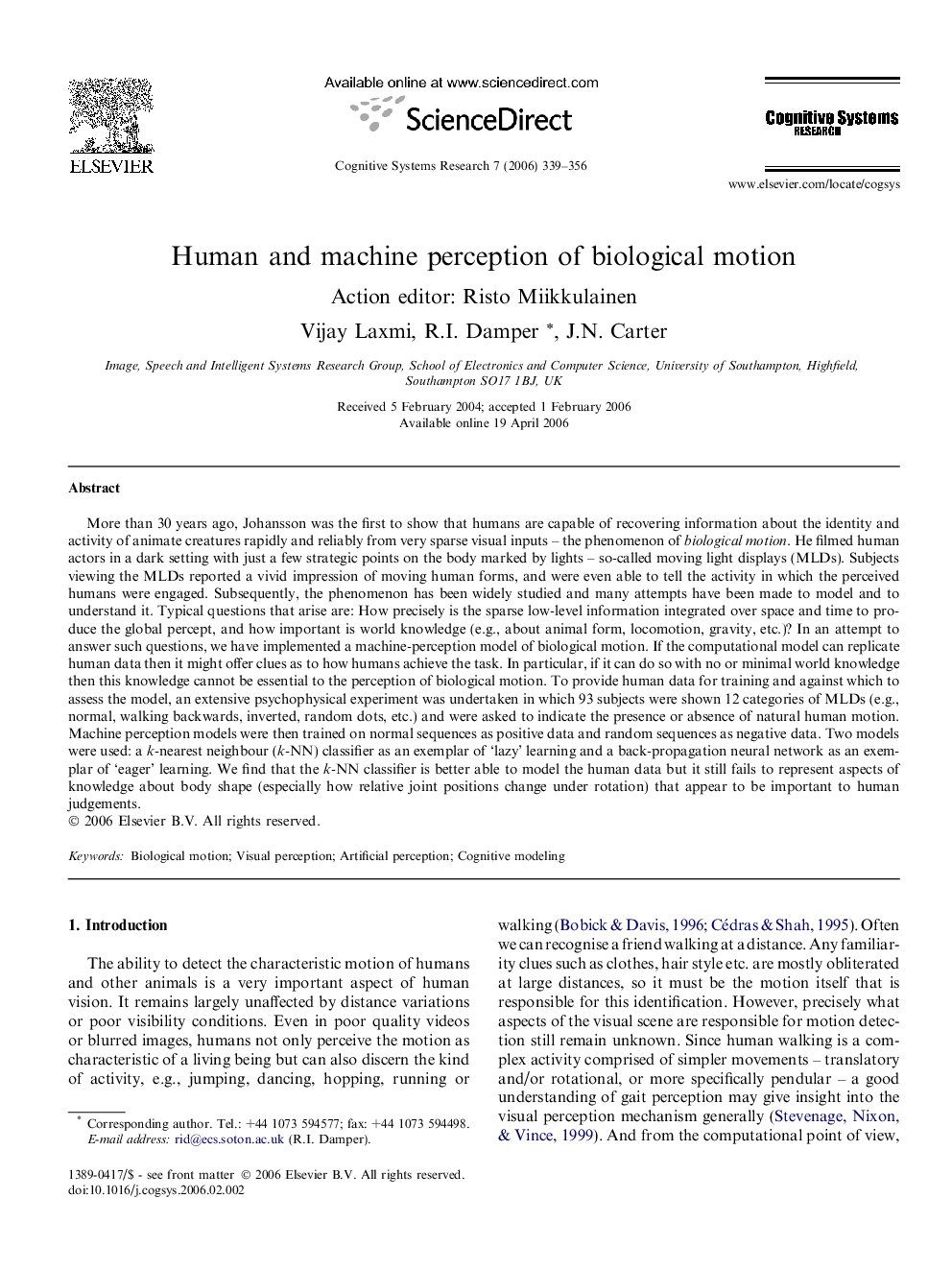
More than 30 years ago, Johansson was the first to show that humans are capable of recovering information about the identity and activity of animate creatures rapidly and reliably from very sparse visual inputs – the phenomenon of biological motion. He filmed human actors in a dark setting with just a few strategic points on the body marked by lights – so-called moving light displays (MLDs). Subjects viewing the MLDs reported a vivid impression of moving human forms, and were even able to tell the activity in which the perceived humans were engaged. Subsequently, the phenomenon has been widely studied and many attempts have been made to model and to understand it. Typical questions that arise are: How precisely is the sparse low-level information integrated over space and time to produce the global percept, and how important is world knowledge (e.g., about animal form, locomotion, gravity, etc.)? In an attempt to answer such questions, we have implemented a machine-perception model of biological motion. If the computational model can replicate human data then it might offer clues as to how humans achieve the task. In particular, if it can do so with no or minimal world knowledge then this knowledge cannot be essential to the perception of biological motion. To provide human data for training and against which to assess the model, an extensive psychophysical experiment was undertaken in which 93 subjects were shown 12 categories of MLDs (e.g., normal, walking backwards, inverted, random dots, etc.) and were asked to indicate the presence or absence of natural human motion. Machine perception models were then trained on normal sequences as positive data and random sequences as negative data. Two models were used: a k-nearest neighbour (k-NN) classifier as an exemplar of ‘lazy’ learning and a back-propagation neural network as an exemplar of ‘eager’ learning. We find that the k-NN classifier is better able to model the human data but it still fails to represent aspects of knowledge about body shape (especially how relative joint positions change under rotation) that appear to be important to human judgements.
Journal: Cognitive Systems Research - Volume 7, Issue 4, December 2006, Pages 339–356