کد مقاله | کد نشریه | سال انتشار | مقاله انگلیسی | نسخه تمام متن |
---|---|---|---|---|
379104 | 659264 | 2010 | 21 صفحه PDF | دانلود رایگان |
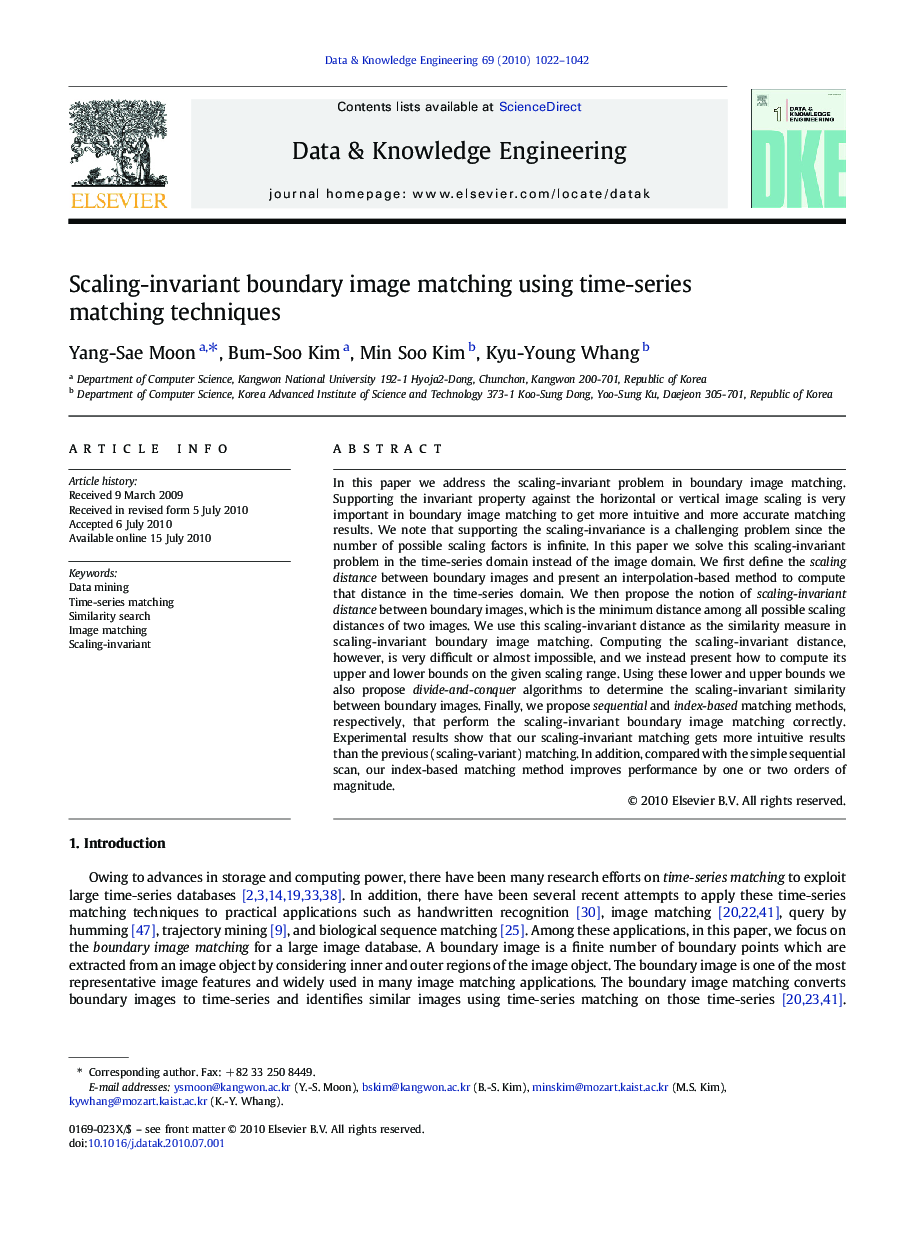
In this paper we address the scaling-invariant problem in boundary image matching. Supporting the invariant property against the horizontal or vertical image scaling is very important in boundary image matching to get more intuitive and more accurate matching results. We note that supporting the scaling-invariance is a challenging problem since the number of possible scaling factors is infinite. In this paper we solve this scaling-invariant problem in the time-series domain instead of the image domain. We first define the scaling distance between boundary images and present an interpolation-based method to compute that distance in the time-series domain. We then propose the notion of scaling-invariant distance between boundary images, which is the minimum distance among all possible scaling distances of two images. We use this scaling-invariant distance as the similarity measure in scaling-invariant boundary image matching. Computing the scaling-invariant distance, however, is very difficult or almost impossible, and we instead present how to compute its upper and lower bounds on the given scaling range. Using these lower and upper bounds we also propose divide-and-conquer algorithms to determine the scaling-invariant similarity between boundary images. Finally, we propose sequential and index-based matching methods, respectively, that perform the scaling-invariant boundary image matching correctly. Experimental results show that our scaling-invariant matching gets more intuitive results than the previous (scaling-variant) matching. In addition, compared with the simple sequential scan, our index-based matching method improves performance by one or two orders of magnitude.
Journal: Data & Knowledge Engineering - Volume 69, Issue 10, October 2010, Pages 1022–1042