کد مقاله | کد نشریه | سال انتشار | مقاله انگلیسی | نسخه تمام متن |
---|---|---|---|---|
402234 | 676880 | 2015 | 15 صفحه PDF | دانلود رایگان |
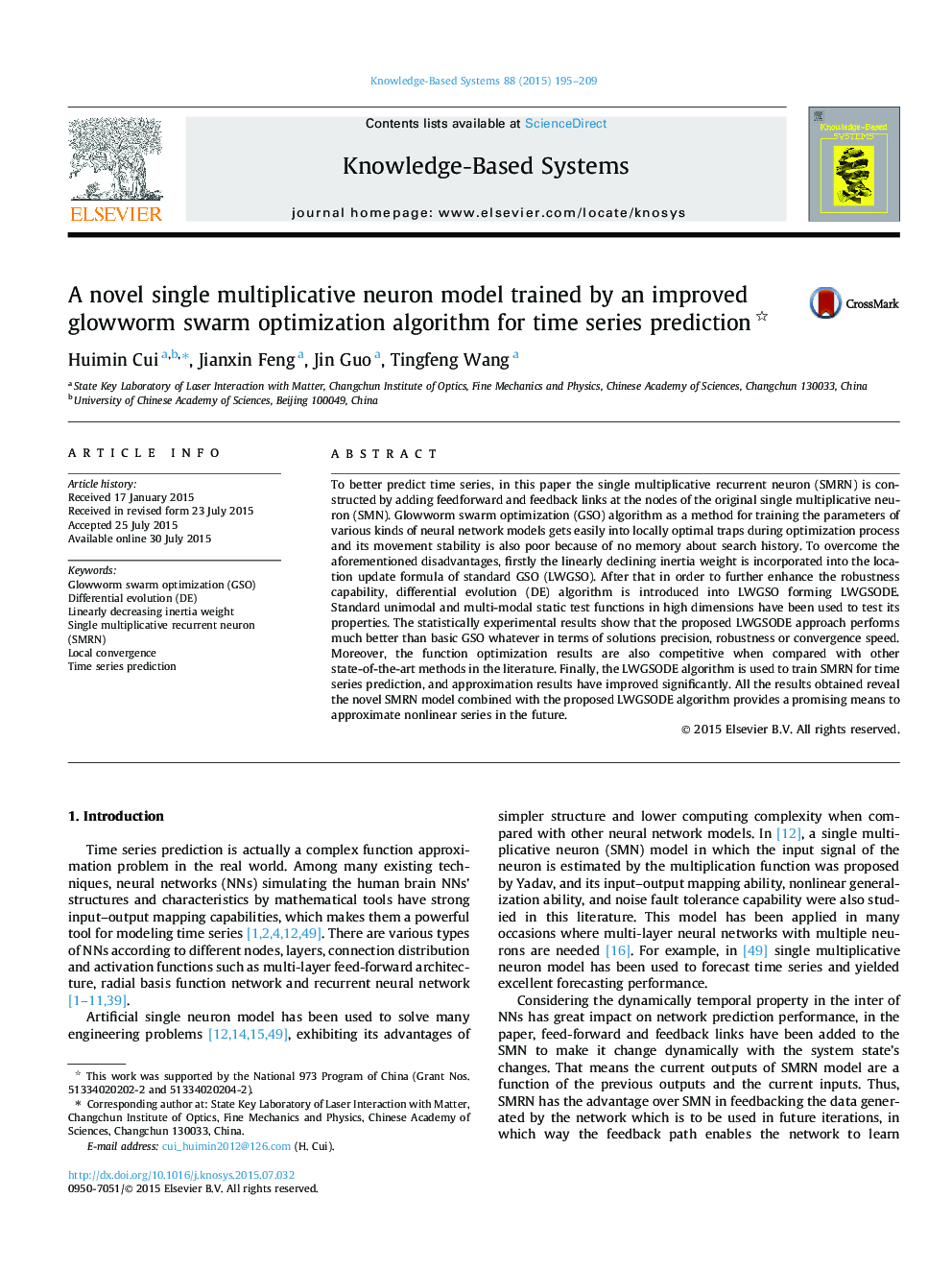
To better predict time series, in this paper the single multiplicative recurrent neuron (SMRN) is constructed by adding feedforward and feedback links at the nodes of the original single multiplicative neuron (SMN). Glowworm swarm optimization (GSO) algorithm as a method for training the parameters of various kinds of neural network models gets easily into locally optimal traps during optimization process and its movement stability is also poor because of no memory about search history. To overcome the aforementioned disadvantages, firstly the linearly declining inertia weight is incorporated into the location update formula of standard GSO (LWGSO). After that in order to further enhance the robustness capability, differential evolution (DE) algorithm is introduced into LWGSO forming LWGSODE. Standard unimodal and multi-modal static test functions in high dimensions have been used to test its properties. The statistically experimental results show that the proposed LWGSODE approach performs much better than basic GSO whatever in terms of solutions precision, robustness or convergence speed. Moreover, the function optimization results are also competitive when compared with other state-of-the-art methods in the literature. Finally, the LWGSODE algorithm is used to train SMRN for time series prediction, and approximation results have improved significantly. All the results obtained reveal the novel SMRN model combined with the proposed LWGSODE algorithm provides a promising means to approximate nonlinear series in the future.
Journal: Knowledge-Based Systems - Volume 88, November 2015, Pages 195–209