کد مقاله | کد نشریه | سال انتشار | مقاله انگلیسی | نسخه تمام متن |
---|---|---|---|---|
404223 | 677400 | 2012 | 11 صفحه PDF | دانلود رایگان |
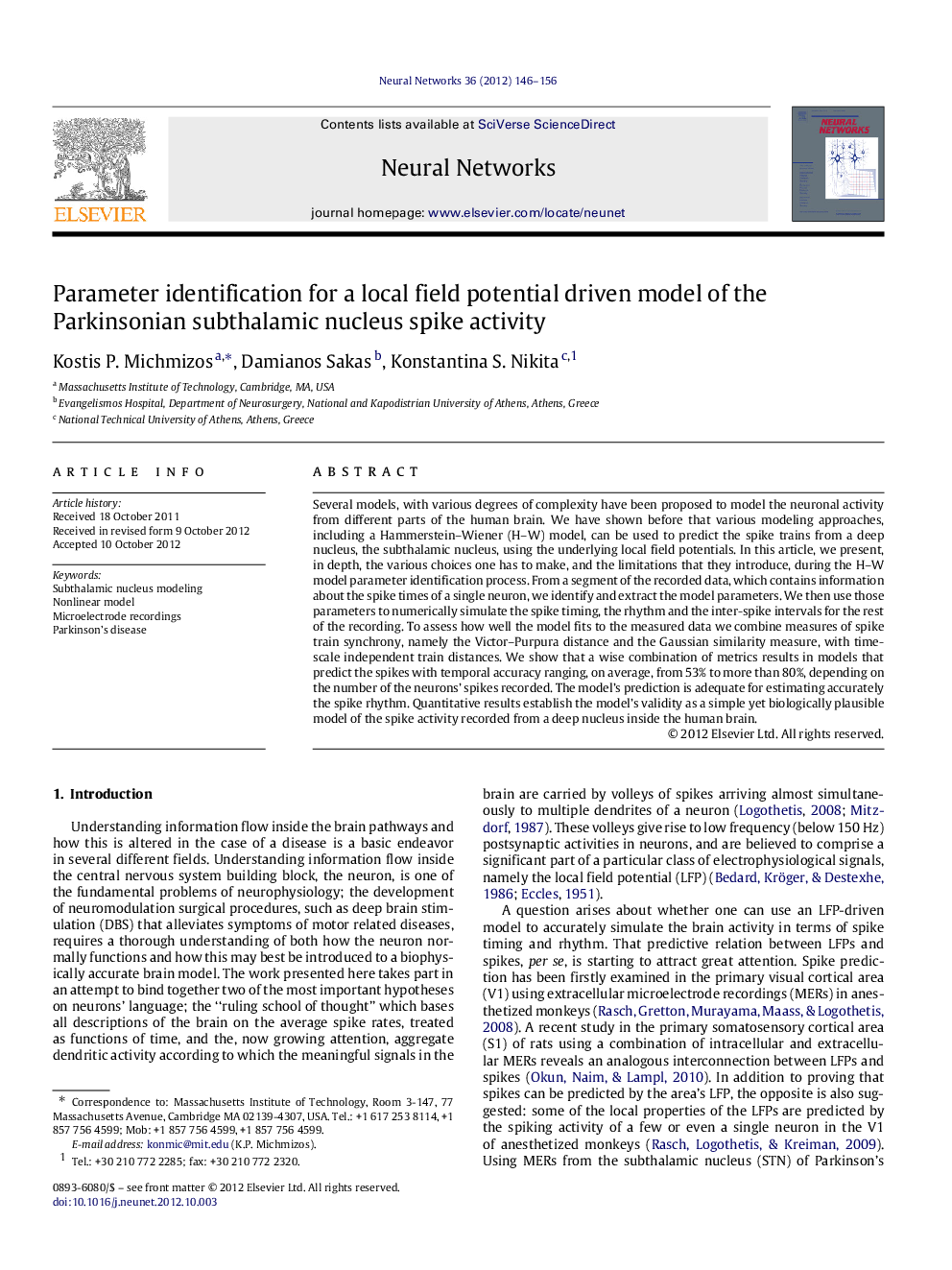
Several models, with various degrees of complexity have been proposed to model the neuronal activity from different parts of the human brain. We have shown before that various modeling approaches, including a Hammerstein–Wiener (H–W) model, can be used to predict the spike trains from a deep nucleus, the subthalamic nucleus, using the underlying local field potentials. In this article, we present, in depth, the various choices one has to make, and the limitations that they introduce, during the H–W model parameter identification process. From a segment of the recorded data, which contains information about the spike times of a single neuron, we identify and extract the model parameters. We then use those parameters to numerically simulate the spike timing, the rhythm and the inter-spike intervals for the rest of the recording. To assess how well the model fits to the measured data we combine measures of spike train synchrony, namely the Victor–Purpura distance and the Gaussian similarity measure, with time-scale independent train distances. We show that a wise combination of metrics results in models that predict the spikes with temporal accuracy ranging, on average, from 53% to more than 80%, depending on the number of the neurons’ spikes recorded. The model’s prediction is adequate for estimating accurately the spike rhythm. Quantitative results establish the model’s validity as a simple yet biologically plausible model of the spike activity recorded from a deep nucleus inside the human brain.
Journal: Neural Networks - Volume 36, December 2012, Pages 146–156