کد مقاله | کد نشریه | سال انتشار | مقاله انگلیسی | نسخه تمام متن |
---|---|---|---|---|
404289 | 677410 | 2011 | 8 صفحه PDF | دانلود رایگان |
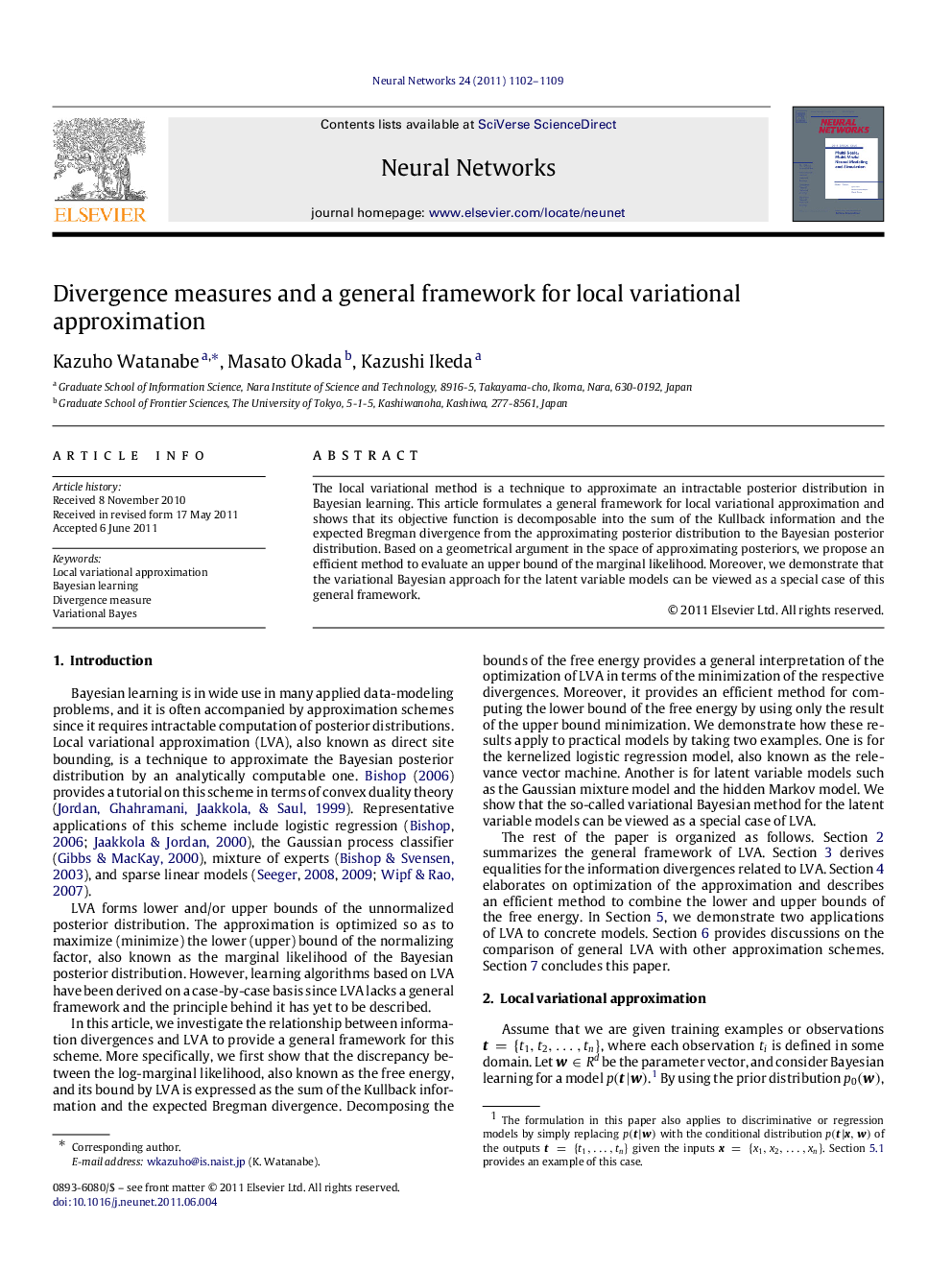
The local variational method is a technique to approximate an intractable posterior distribution in Bayesian learning. This article formulates a general framework for local variational approximation and shows that its objective function is decomposable into the sum of the Kullback information and the expected Bregman divergence from the approximating posterior distribution to the Bayesian posterior distribution. Based on a geometrical argument in the space of approximating posteriors, we propose an efficient method to evaluate an upper bound of the marginal likelihood. Moreover, we demonstrate that the variational Bayesian approach for the latent variable models can be viewed as a special case of this general framework.
► We present a general framework for local variational approximation of Bayesian learning.
► We provide decompositions of the free energy bounds in terms of the Kullback information and the Bregman divergence.
► We also provide an efficient method for computing the upper bound of the marginal likelihood and its geometrical interpretation.
► Applications to the kernelized logistic regression model and latent variable models are given.
Journal: Neural Networks - Volume 24, Issue 10, December 2011, Pages 1102–1109