کد مقاله | کد نشریه | سال انتشار | مقاله انگلیسی | نسخه تمام متن |
---|---|---|---|---|
404459 | 677425 | 2009 | 7 صفحه PDF | دانلود رایگان |
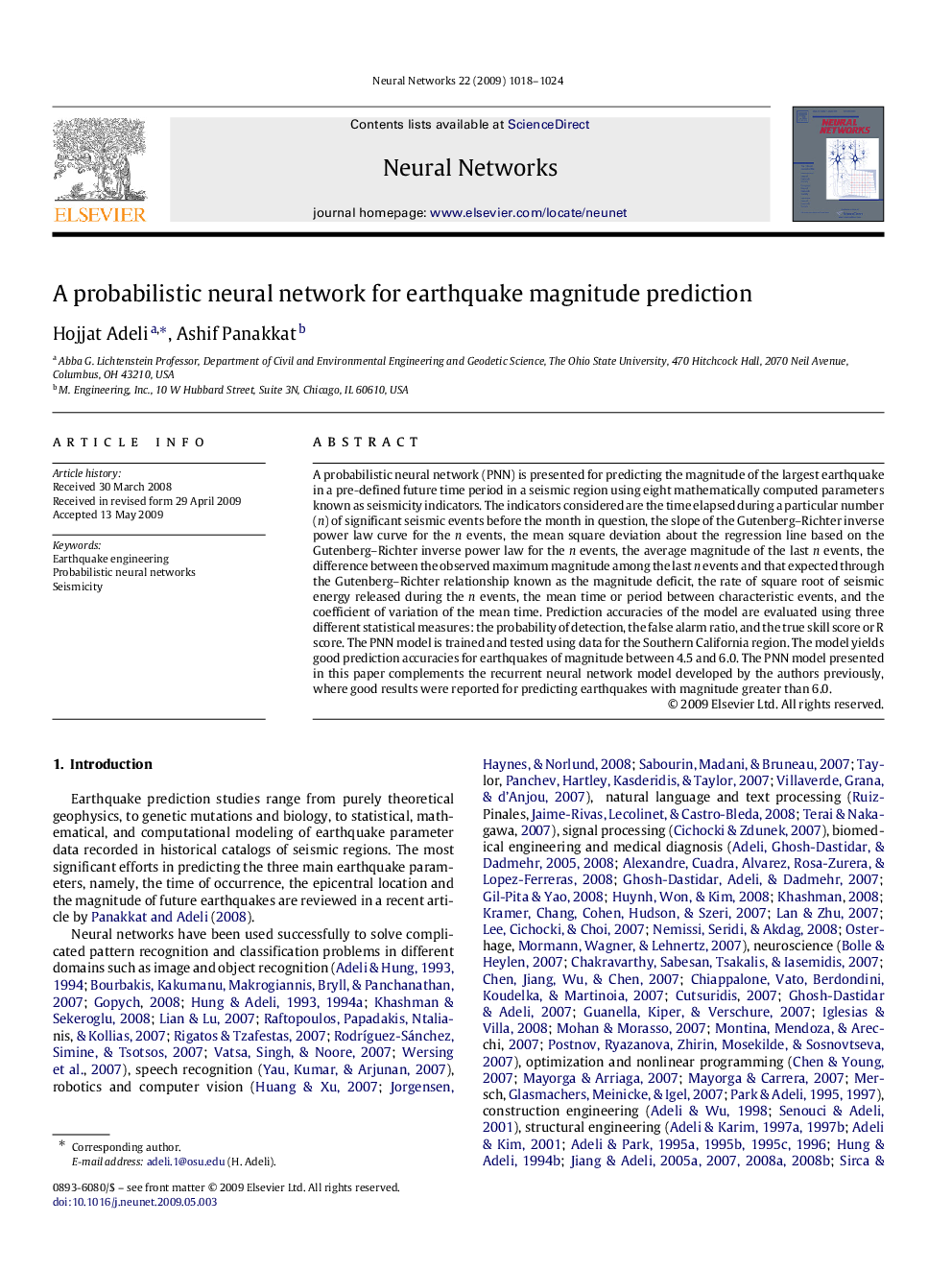
A probabilistic neural network (PNN) is presented for predicting the magnitude of the largest earthquake in a pre-defined future time period in a seismic region using eight mathematically computed parameters known as seismicity indicators. The indicators considered are the time elapsed during a particular number (nn) of significant seismic events before the month in question, the slope of the Gutenberg–Richter inverse power law curve for the nn events, the mean square deviation about the regression line based on the Gutenberg–Richter inverse power law for the nn events, the average magnitude of the last nn events, the difference between the observed maximum magnitude among the last nn events and that expected through the Gutenberg–Richter relationship known as the magnitude deficit, the rate of square root of seismic energy released during the nn events, the mean time or period between characteristic events, and the coefficient of variation of the mean time. Prediction accuracies of the model are evaluated using three different statistical measures: the probability of detection, the false alarm ratio, and the true skill score or R score. The PNN model is trained and tested using data for the Southern California region. The model yields good prediction accuracies for earthquakes of magnitude between 4.5 and 6.0. The PNN model presented in this paper complements the recurrent neural network model developed by the authors previously, where good results were reported for predicting earthquakes with magnitude greater than 6.0.
Journal: Neural Networks - Volume 22, Issue 7, September 2009, Pages 1018–1024