کد مقاله | کد نشریه | سال انتشار | مقاله انگلیسی | نسخه تمام متن |
---|---|---|---|---|
404479 | 677427 | 2010 | 8 صفحه PDF | دانلود رایگان |
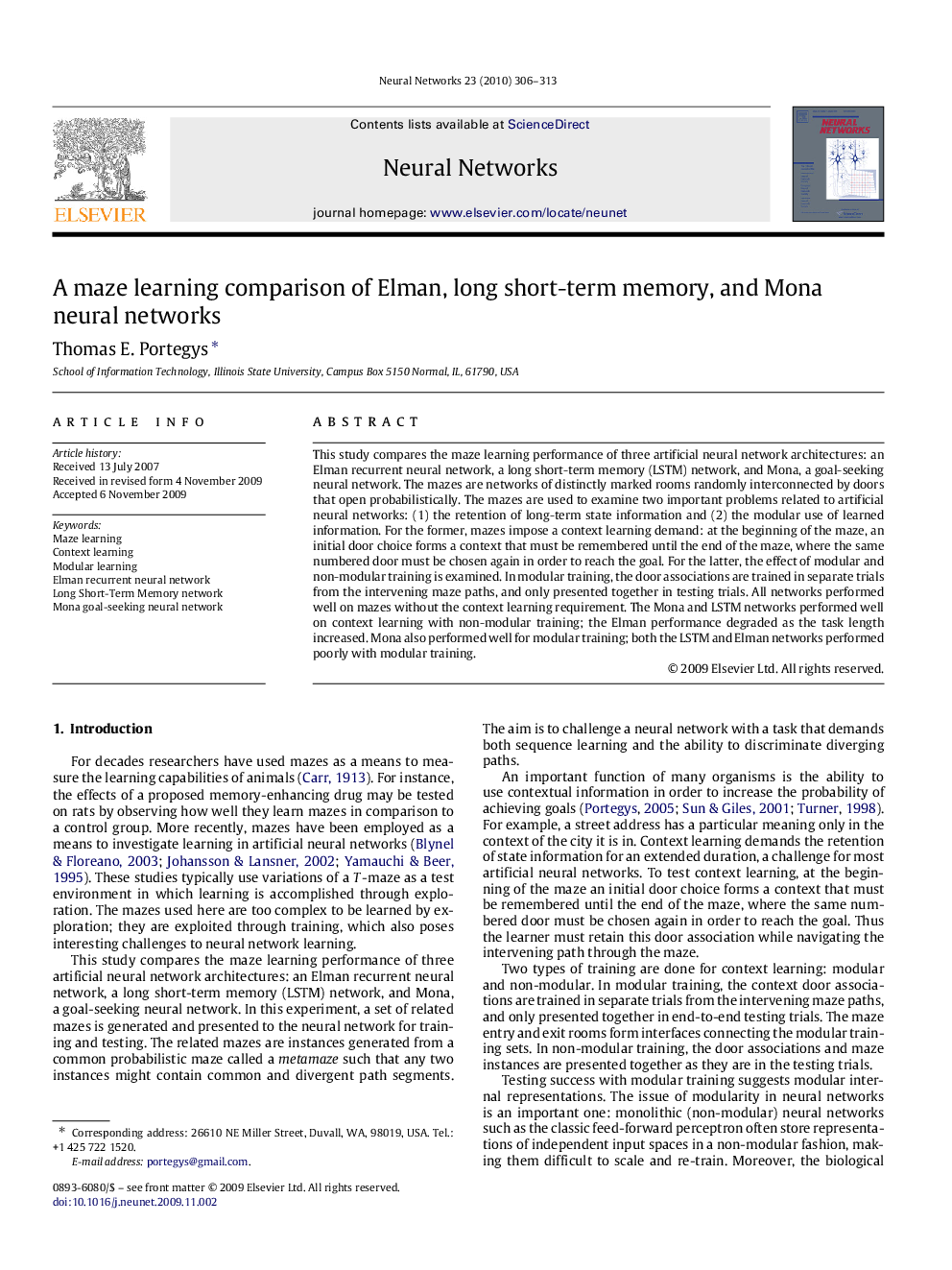
This study compares the maze learning performance of three artificial neural network architectures: an Elman recurrent neural network, a long short-term memory (LSTM) network, and Mona, a goal-seeking neural network. The mazes are networks of distinctly marked rooms randomly interconnected by doors that open probabilistically. The mazes are used to examine two important problems related to artificial neural networks: (1) the retention of long-term state information and (2) the modular use of learned information. For the former, mazes impose a context learning demand: at the beginning of the maze, an initial door choice forms a context that must be remembered until the end of the maze, where the same numbered door must be chosen again in order to reach the goal. For the latter, the effect of modular and non-modular training is examined. In modular training, the door associations are trained in separate trials from the intervening maze paths, and only presented together in testing trials. All networks performed well on mazes without the context learning requirement. The Mona and LSTM networks performed well on context learning with non-modular training; the Elman performance degraded as the task length increased. Mona also performed well for modular training; both the LSTM and Elman networks performed poorly with modular training.
Journal: Neural Networks - Volume 23, Issue 2, March 2010, Pages 306–313