کد مقاله | کد نشریه | سال انتشار | مقاله انگلیسی | نسخه تمام متن |
---|---|---|---|---|
404634 | 677441 | 2009 | 10 صفحه PDF | دانلود رایگان |
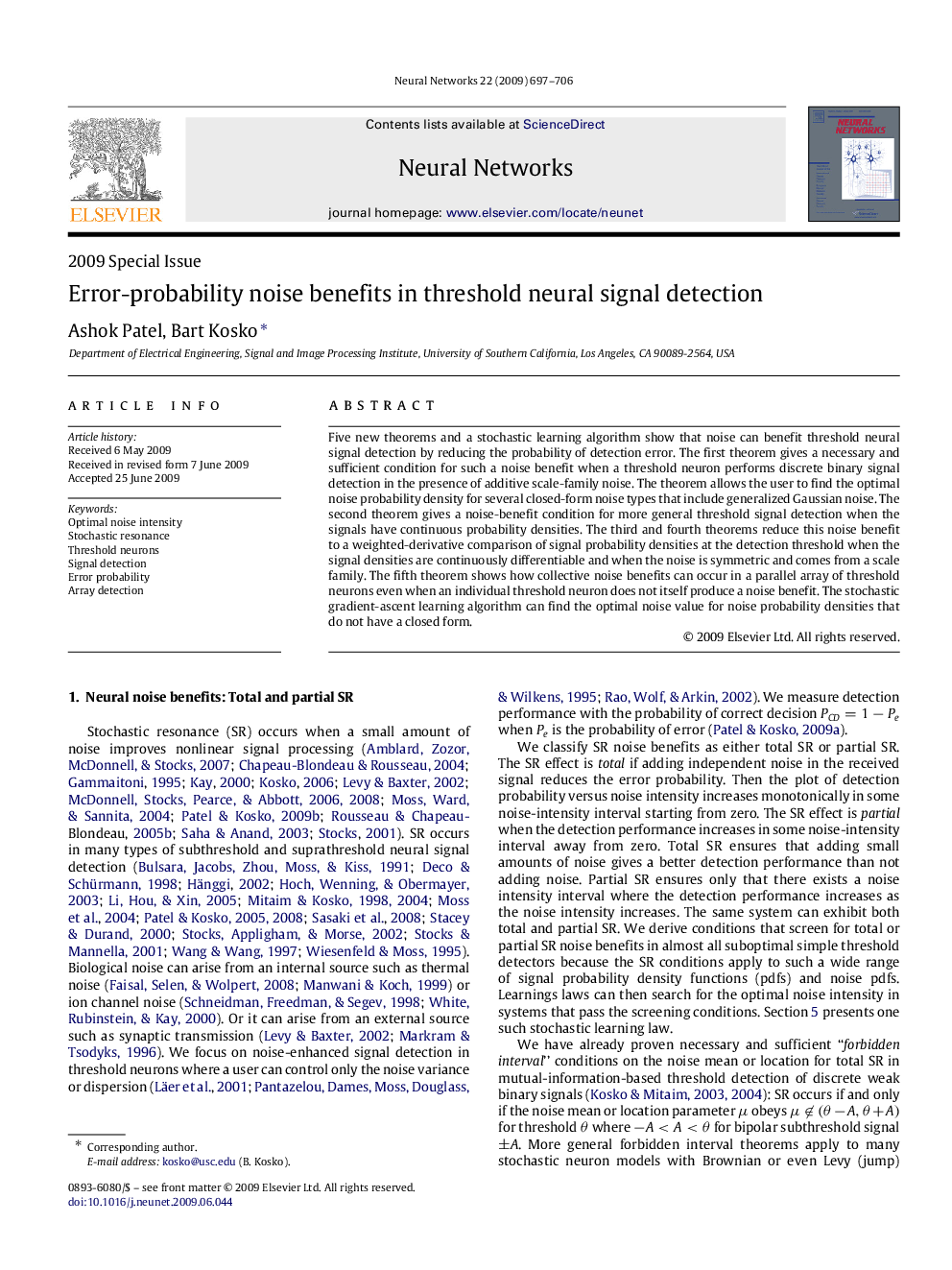
Five new theorems and a stochastic learning algorithm show that noise can benefit threshold neural signal detection by reducing the probability of detection error. The first theorem gives a necessary and sufficient condition for such a noise benefit when a threshold neuron performs discrete binary signal detection in the presence of additive scale-family noise. The theorem allows the user to find the optimal noise probability density for several closed-form noise types that include generalized Gaussian noise. The second theorem gives a noise-benefit condition for more general threshold signal detection when the signals have continuous probability densities. The third and fourth theorems reduce this noise benefit to a weighted-derivative comparison of signal probability densities at the detection threshold when the signal densities are continuously differentiable and when the noise is symmetric and comes from a scale family. The fifth theorem shows how collective noise benefits can occur in a parallel array of threshold neurons even when an individual threshold neuron does not itself produce a noise benefit. The stochastic gradient-ascent learning algorithm can find the optimal noise value for noise probability densities that do not have a closed form.
Journal: Neural Networks - Volume 22, Issues 5–6, July–August 2009, Pages 697–706