کد مقاله | کد نشریه | سال انتشار | مقاله انگلیسی | نسخه تمام متن |
---|---|---|---|---|
405431 | 677614 | 2016 | 13 صفحه PDF | دانلود رایگان |
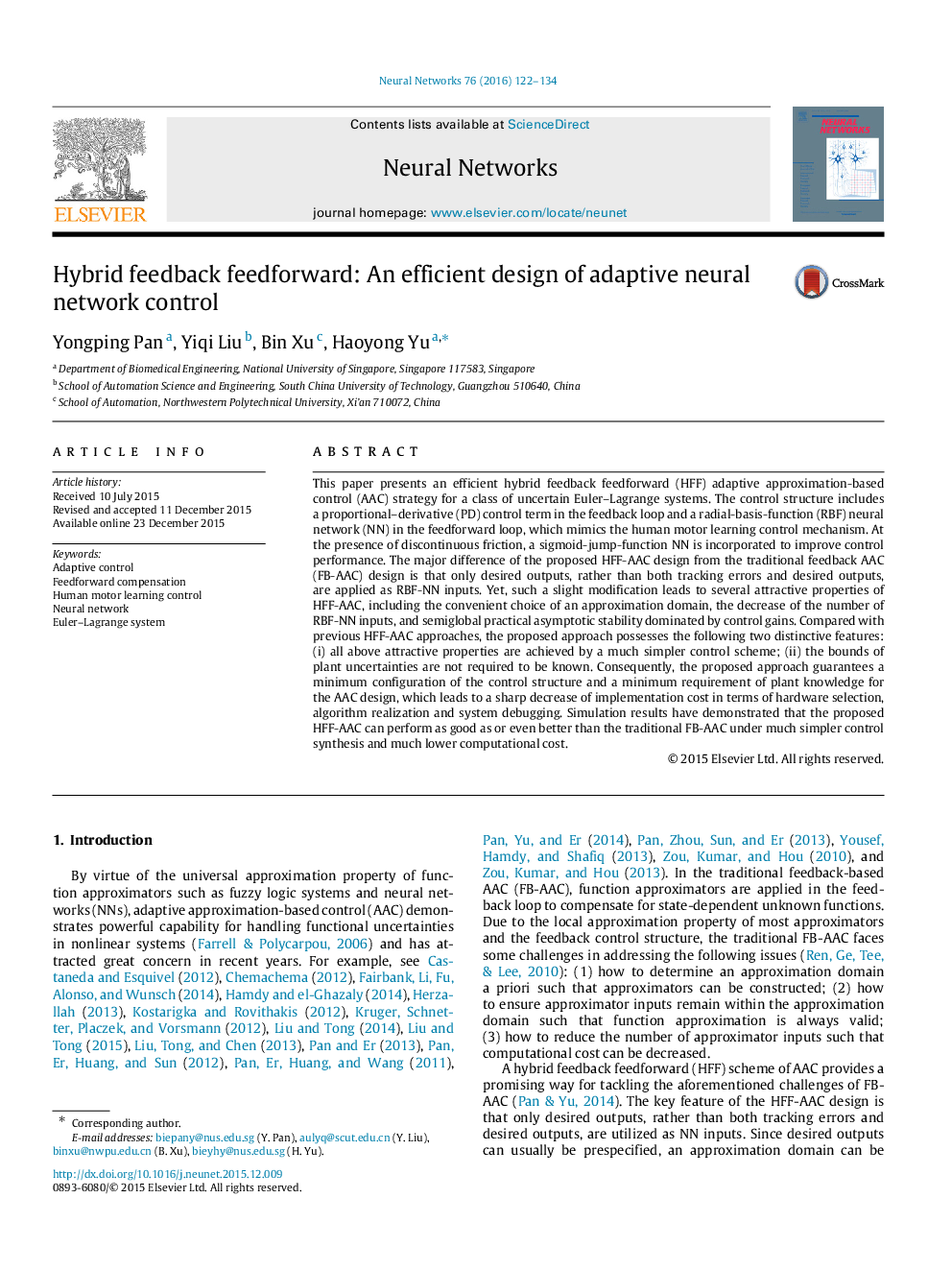
This paper presents an efficient hybrid feedback feedforward (HFF) adaptive approximation-based control (AAC) strategy for a class of uncertain Euler–Lagrange systems. The control structure includes a proportional–derivative (PD) control term in the feedback loop and a radial-basis-function (RBF) neural network (NN) in the feedforward loop, which mimics the human motor learning control mechanism. At the presence of discontinuous friction, a sigmoid-jump-function NN is incorporated to improve control performance. The major difference of the proposed HFF-AAC design from the traditional feedback AAC (FB-AAC) design is that only desired outputs, rather than both tracking errors and desired outputs, are applied as RBF-NN inputs. Yet, such a slight modification leads to several attractive properties of HFF-AAC, including the convenient choice of an approximation domain, the decrease of the number of RBF-NN inputs, and semiglobal practical asymptotic stability dominated by control gains. Compared with previous HFF-AAC approaches, the proposed approach possesses the following two distinctive features: (i) all above attractive properties are achieved by a much simpler control scheme; (ii) the bounds of plant uncertainties are not required to be known. Consequently, the proposed approach guarantees a minimum configuration of the control structure and a minimum requirement of plant knowledge for the AAC design, which leads to a sharp decrease of implementation cost in terms of hardware selection, algorithm realization and system debugging. Simulation results have demonstrated that the proposed HFF-AAC can perform as good as or even better than the traditional FB-AAC under much simpler control synthesis and much lower computational cost.
Journal: Neural Networks - Volume 76, April 2016, Pages 122–134