کد مقاله | کد نشریه | سال انتشار | مقاله انگلیسی | نسخه تمام متن |
---|---|---|---|---|
405515 | 677655 | 2012 | 10 صفحه PDF | دانلود رایگان |
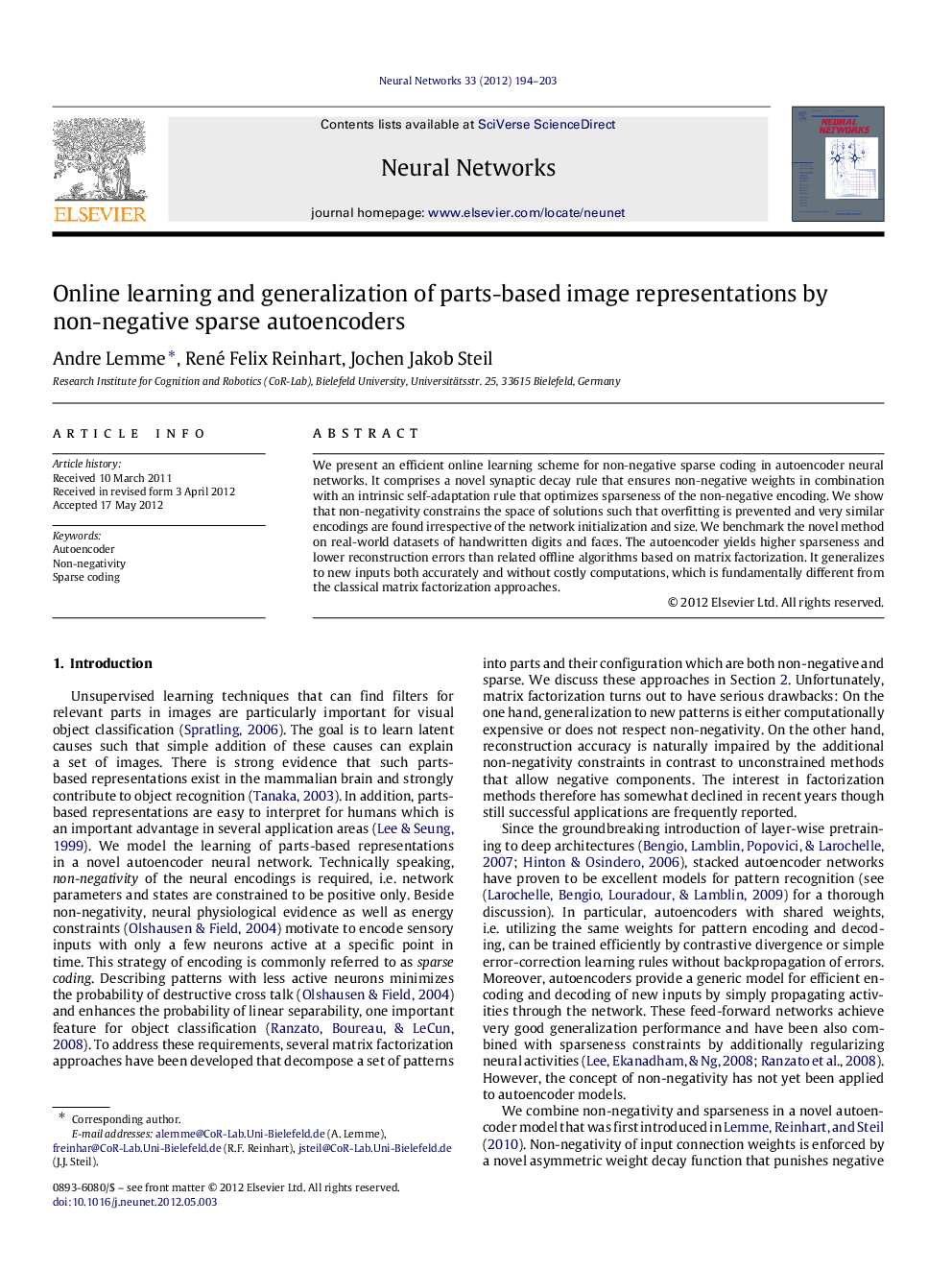
We present an efficient online learning scheme for non-negative sparse coding in autoencoder neural networks. It comprises a novel synaptic decay rule that ensures non-negative weights in combination with an intrinsic self-adaptation rule that optimizes sparseness of the non-negative encoding. We show that non-negativity constrains the space of solutions such that overfitting is prevented and very similar encodings are found irrespective of the network initialization and size. We benchmark the novel method on real-world datasets of handwritten digits and faces. The autoencoder yields higher sparseness and lower reconstruction errors than related offline algorithms based on matrix factorization. It generalizes to new inputs both accurately and without costly computations, which is fundamentally different from the classical matrix factorization approaches.
Journal: Neural Networks - Volume 33, September 2012, Pages 194–203