کد مقاله | کد نشریه | سال انتشار | مقاله انگلیسی | نسخه تمام متن |
---|---|---|---|---|
405571 | 677676 | 2011 | 8 صفحه PDF | دانلود رایگان |
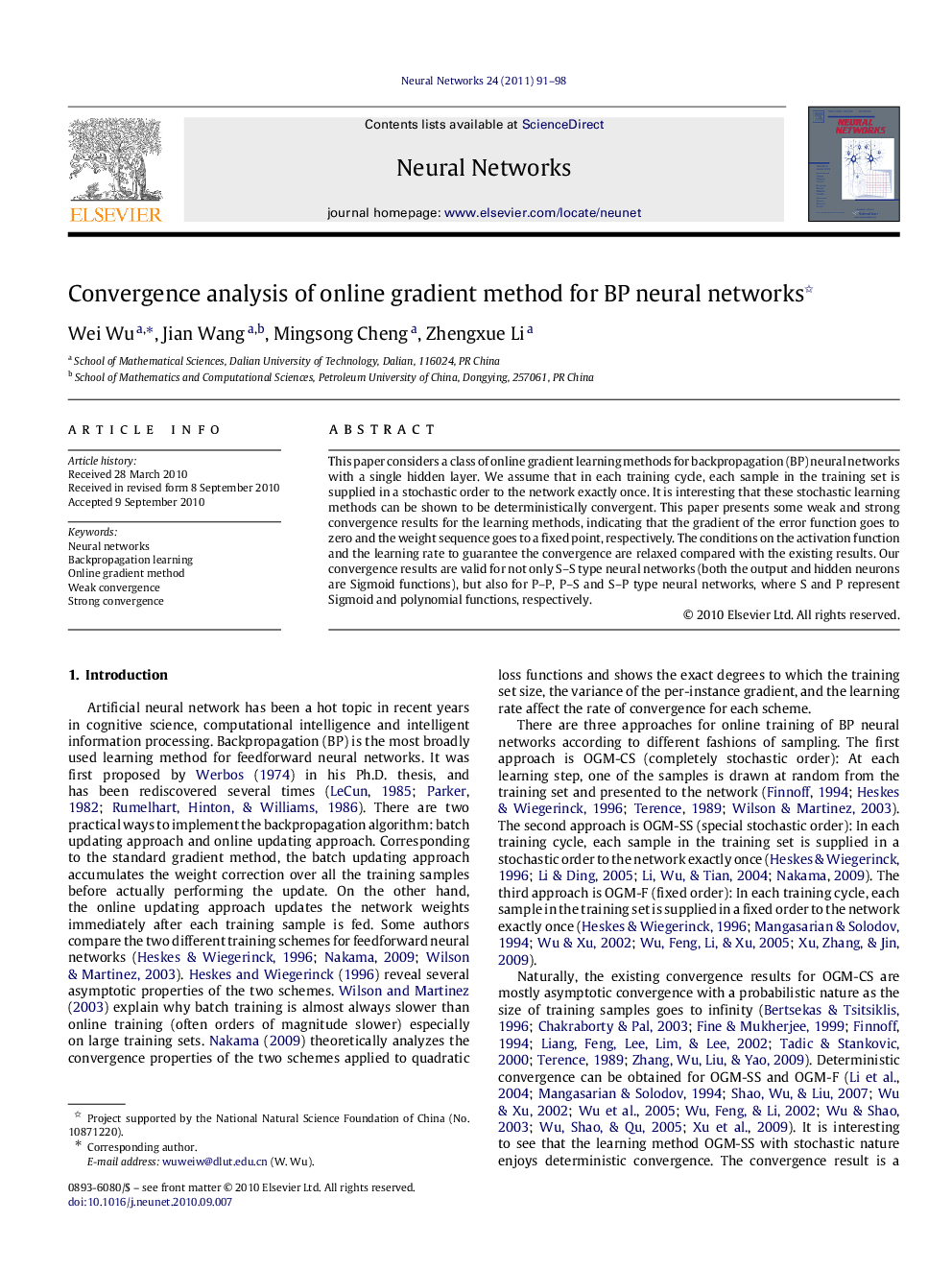
This paper considers a class of online gradient learning methods for backpropagation (BP) neural networks with a single hidden layer. We assume that in each training cycle, each sample in the training set is supplied in a stochastic order to the network exactly once. It is interesting that these stochastic learning methods can be shown to be deterministically convergent. This paper presents some weak and strong convergence results for the learning methods, indicating that the gradient of the error function goes to zero and the weight sequence goes to a fixed point, respectively. The conditions on the activation function and the learning rate to guarantee the convergence are relaxed compared with the existing results. Our convergence results are valid for not only S–S type neural networks (both the output and hidden neurons are Sigmoid functions), but also for P–P, P–S and S–P type neural networks, where S and P represent Sigmoid and polynomial functions, respectively.
Journal: Neural Networks - Volume 24, Issue 1, January 2011, Pages 91–98