کد مقاله | کد نشریه | سال انتشار | مقاله انگلیسی | نسخه تمام متن |
---|---|---|---|---|
407109 | 678128 | 2010 | 14 صفحه PDF | دانلود رایگان |
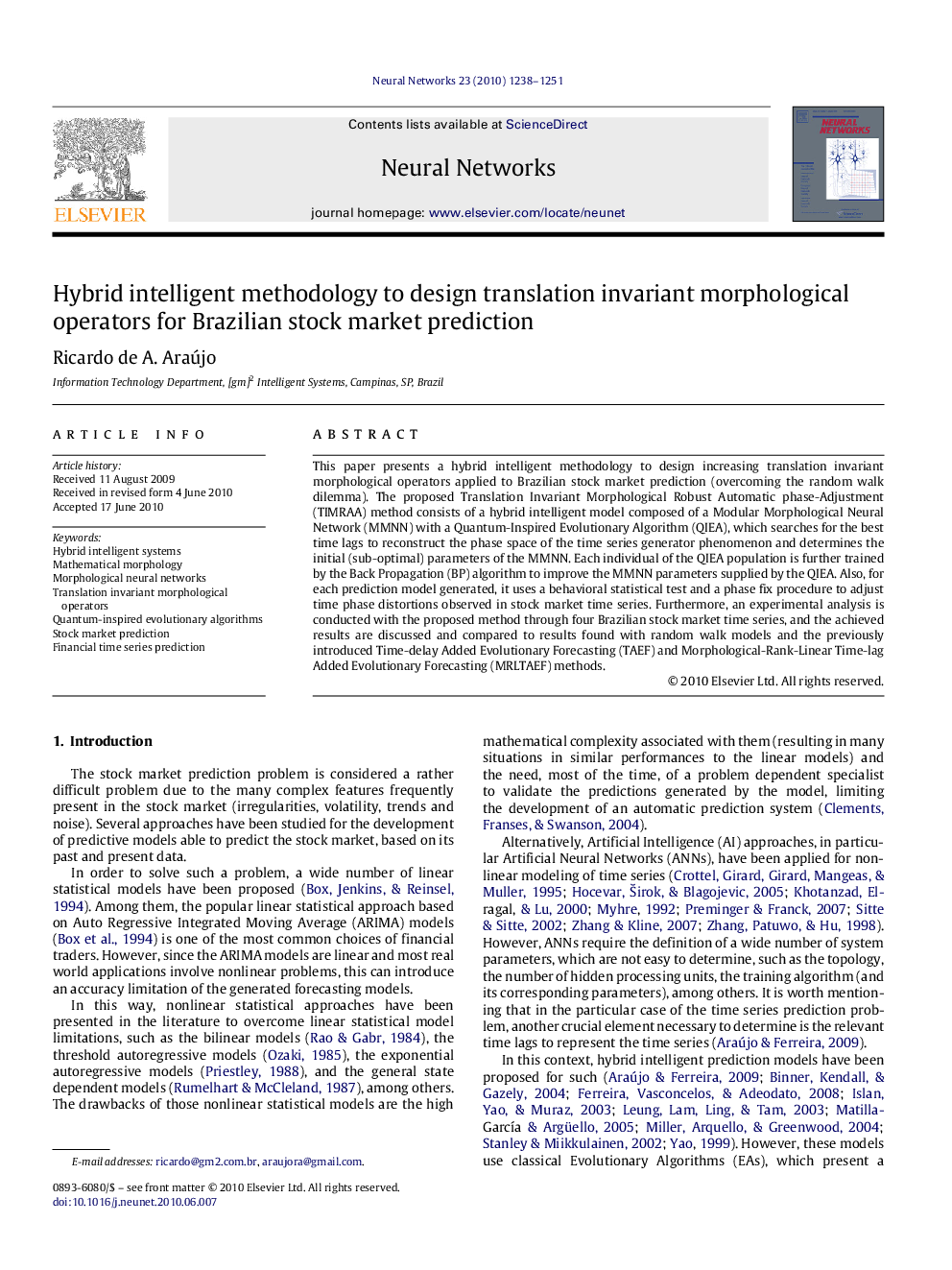
This paper presents a hybrid intelligent methodology to design increasing translation invariant morphological operators applied to Brazilian stock market prediction (overcoming the random walk dilemma). The proposed Translation Invariant Morphological Robust Automatic phase-Adjustment (TIMRAA) method consists of a hybrid intelligent model composed of a Modular Morphological Neural Network (MMNN) with a Quantum-Inspired Evolutionary Algorithm (QIEA), which searches for the best time lags to reconstruct the phase space of the time series generator phenomenon and determines the initial (sub-optimal) parameters of the MMNN. Each individual of the QIEA population is further trained by the Back Propagation (BP) algorithm to improve the MMNN parameters supplied by the QIEA. Also, for each prediction model generated, it uses a behavioral statistical test and a phase fix procedure to adjust time phase distortions observed in stock market time series. Furthermore, an experimental analysis is conducted with the proposed method through four Brazilian stock market time series, and the achieved results are discussed and compared to results found with random walk models and the previously introduced Time-delay Added Evolutionary Forecasting (TAEF) and Morphological-Rank-Linear Time-lag Added Evolutionary Forecasting (MRLTAEF) methods.
Journal: Neural Networks - Volume 23, Issue 10, December 2010, Pages 1238–1251