کد مقاله | کد نشریه | سال انتشار | مقاله انگلیسی | نسخه تمام متن |
---|---|---|---|---|
415039 | 681162 | 2012 | 13 صفحه PDF | دانلود رایگان |
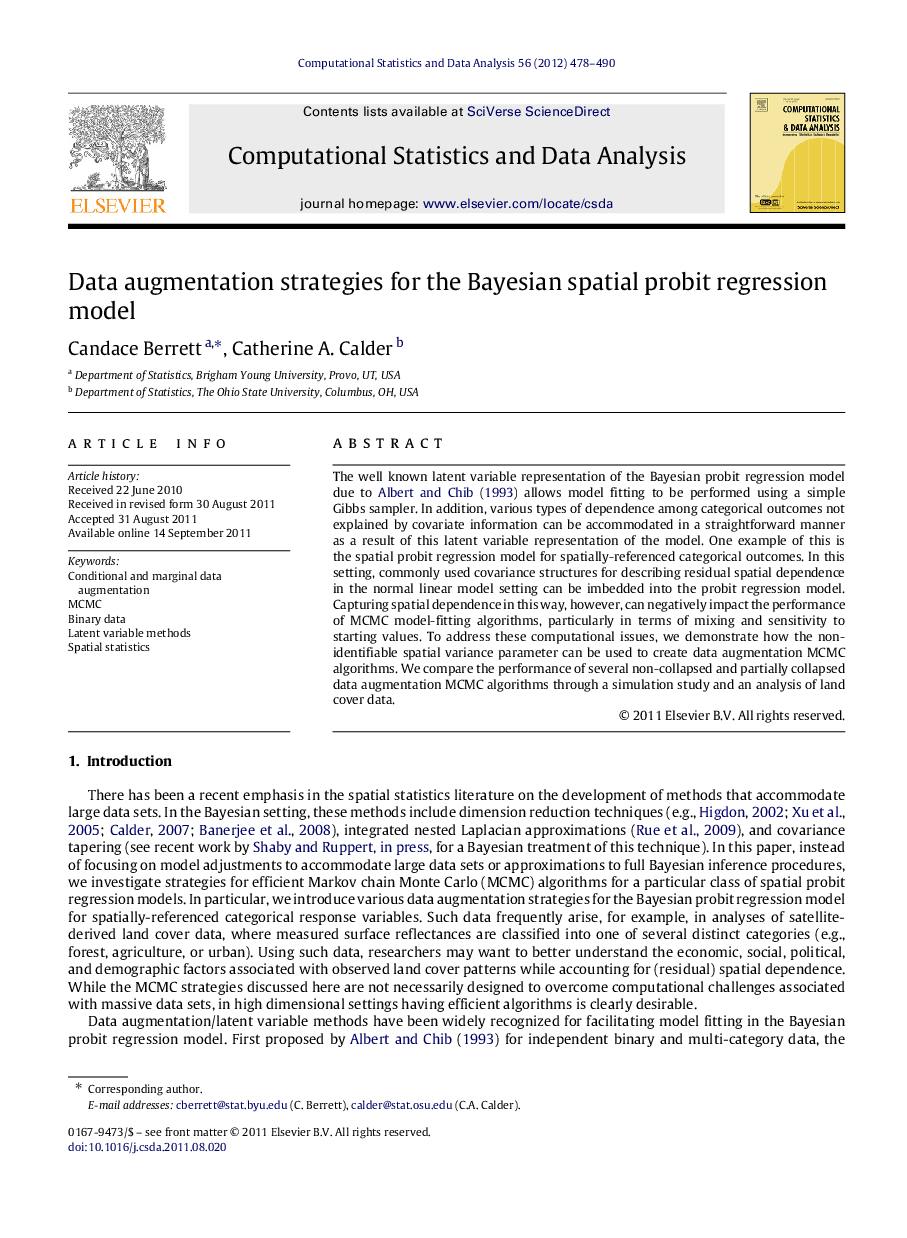
The well known latent variable representation of the Bayesian probit regression model due to Albert and Chib (1993) allows model fitting to be performed using a simple Gibbs sampler. In addition, various types of dependence among categorical outcomes not explained by covariate information can be accommodated in a straightforward manner as a result of this latent variable representation of the model. One example of this is the spatial probit regression model for spatially-referenced categorical outcomes. In this setting, commonly used covariance structures for describing residual spatial dependence in the normal linear model setting can be imbedded into the probit regression model. Capturing spatial dependence in this way, however, can negatively impact the performance of MCMC model-fitting algorithms, particularly in terms of mixing and sensitivity to starting values. To address these computational issues, we demonstrate how the non-identifiable spatial variance parameter can be used to create data augmentation MCMC algorithms. We compare the performance of several non-collapsed and partially collapsed data augmentation MCMC algorithms through a simulation study and an analysis of land cover data.
Journal: Computational Statistics & Data Analysis - Volume 56, Issue 3, 1 March 2012, Pages 478–490