کد مقاله | کد نشریه | سال انتشار | مقاله انگلیسی | نسخه تمام متن |
---|---|---|---|---|
415309 | 681201 | 2016 | 13 صفحه PDF | دانلود رایگان |
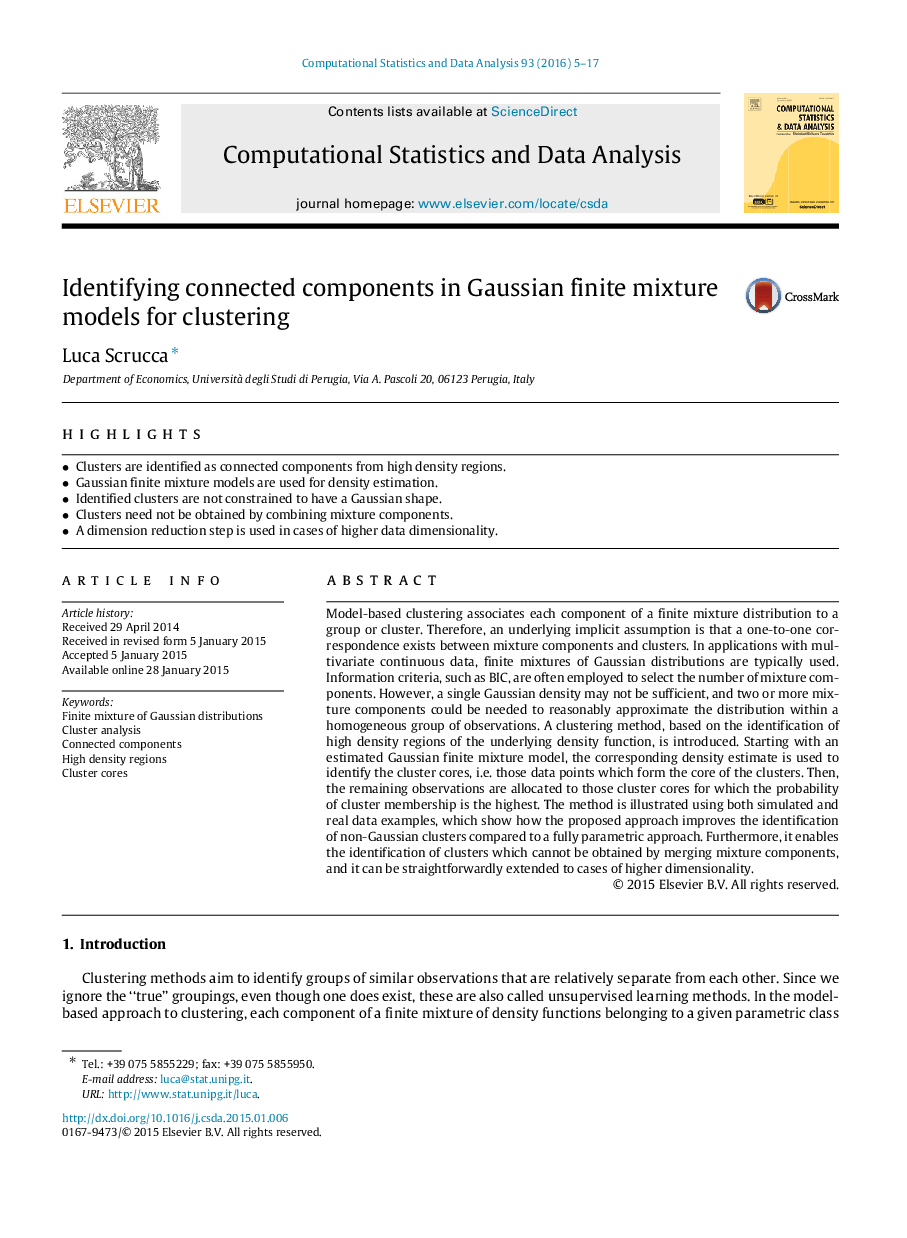
• Clusters are identified as connected components from high density regions.
• Gaussian finite mixture models are used for density estimation.
• Identified clusters are not constrained to have a Gaussian shape.
• Clusters need not be obtained by combining mixture components.
• A dimension reduction step is used in cases of higher data dimensionality.
Model-based clustering associates each component of a finite mixture distribution to a group or cluster. Therefore, an underlying implicit assumption is that a one-to-one correspondence exists between mixture components and clusters. In applications with multivariate continuous data, finite mixtures of Gaussian distributions are typically used. Information criteria, such as BIC, are often employed to select the number of mixture components. However, a single Gaussian density may not be sufficient, and two or more mixture components could be needed to reasonably approximate the distribution within a homogeneous group of observations. A clustering method, based on the identification of high density regions of the underlying density function, is introduced. Starting with an estimated Gaussian finite mixture model, the corresponding density estimate is used to identify the cluster cores, i.e. those data points which form the core of the clusters. Then, the remaining observations are allocated to those cluster cores for which the probability of cluster membership is the highest. The method is illustrated using both simulated and real data examples, which show how the proposed approach improves the identification of non-Gaussian clusters compared to a fully parametric approach. Furthermore, it enables the identification of clusters which cannot be obtained by merging mixture components, and it can be straightforwardly extended to cases of higher dimensionality.
Journal: Computational Statistics & Data Analysis - Volume 93, January 2016, Pages 5–17