کد مقاله | کد نشریه | سال انتشار | مقاله انگلیسی | نسخه تمام متن |
---|---|---|---|---|
415544 | 681214 | 2007 | 18 صفحه PDF | دانلود رایگان |
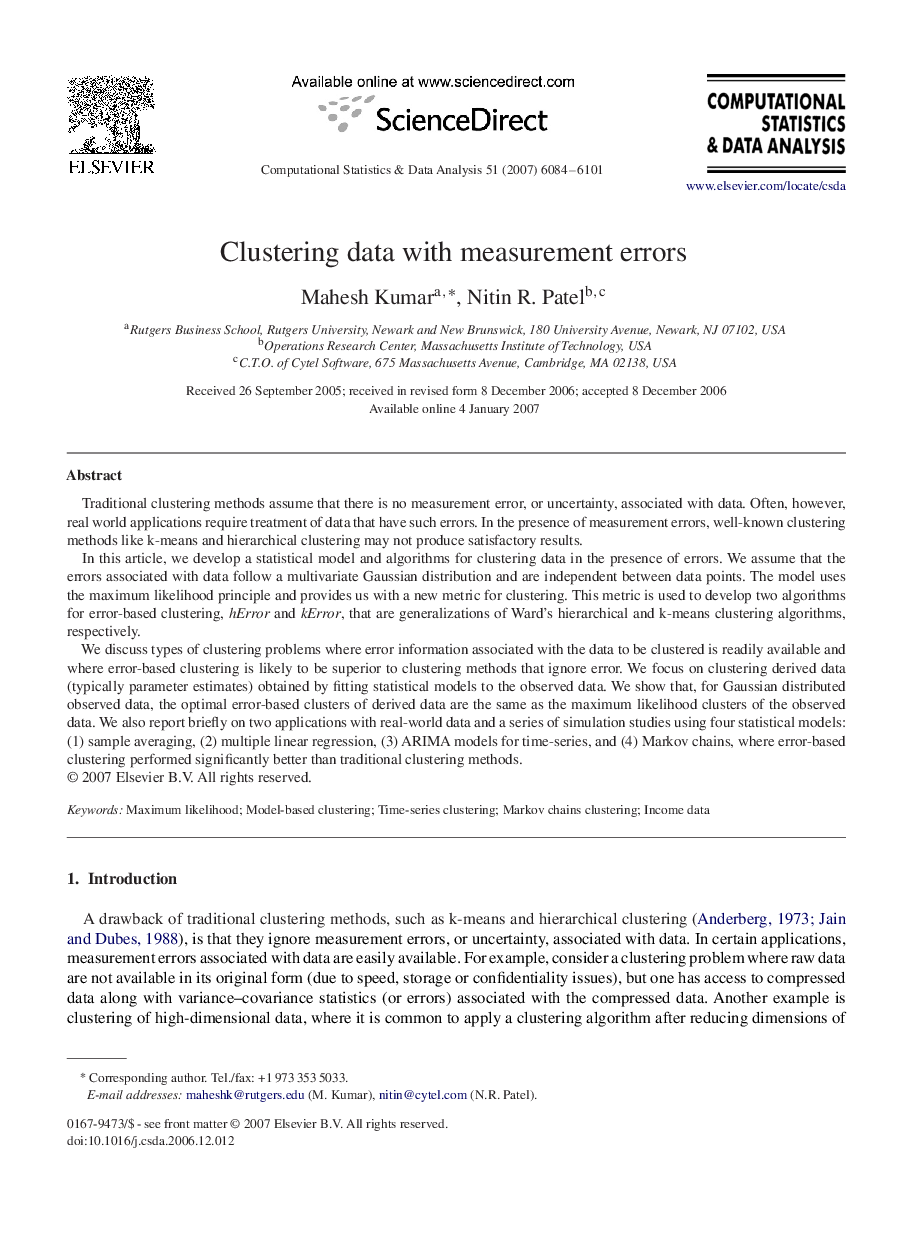
Traditional clustering methods assume that there is no measurement error, or uncertainty, associated with data. Often, however, real world applications require treatment of data that have such errors. In the presence of measurement errors, well-known clustering methods like k-means and hierarchical clustering may not produce satisfactory results.In this article, we develop a statistical model and algorithms for clustering data in the presence of errors. We assume that the errors associated with data follow a multivariate Gaussian distribution and are independent between data points. The model uses the maximum likelihood principle and provides us with a new metric for clustering. This metric is used to develop two algorithms for error-based clustering, hError and kError, that are generalizations of Ward's hierarchical and k-means clustering algorithms, respectively.We discuss types of clustering problems where error information associated with the data to be clustered is readily available and where error-based clustering is likely to be superior to clustering methods that ignore error. We focus on clustering derived data (typically parameter estimates) obtained by fitting statistical models to the observed data. We show that, for Gaussian distributed observed data, the optimal error-based clusters of derived data are the same as the maximum likelihood clusters of the observed data. We also report briefly on two applications with real-world data and a series of simulation studies using four statistical models: (1) sample averaging, (2) multiple linear regression, (3) ARIMA models for time-series, and (4) Markov chains, where error-based clustering performed significantly better than traditional clustering methods.
Journal: Computational Statistics & Data Analysis - Volume 51, Issue 12, 15 August 2007, Pages 6084–6101