کد مقاله | کد نشریه | سال انتشار | مقاله انگلیسی | نسخه تمام متن |
---|---|---|---|---|
415868 | 681247 | 2012 | 18 صفحه PDF | دانلود رایگان |
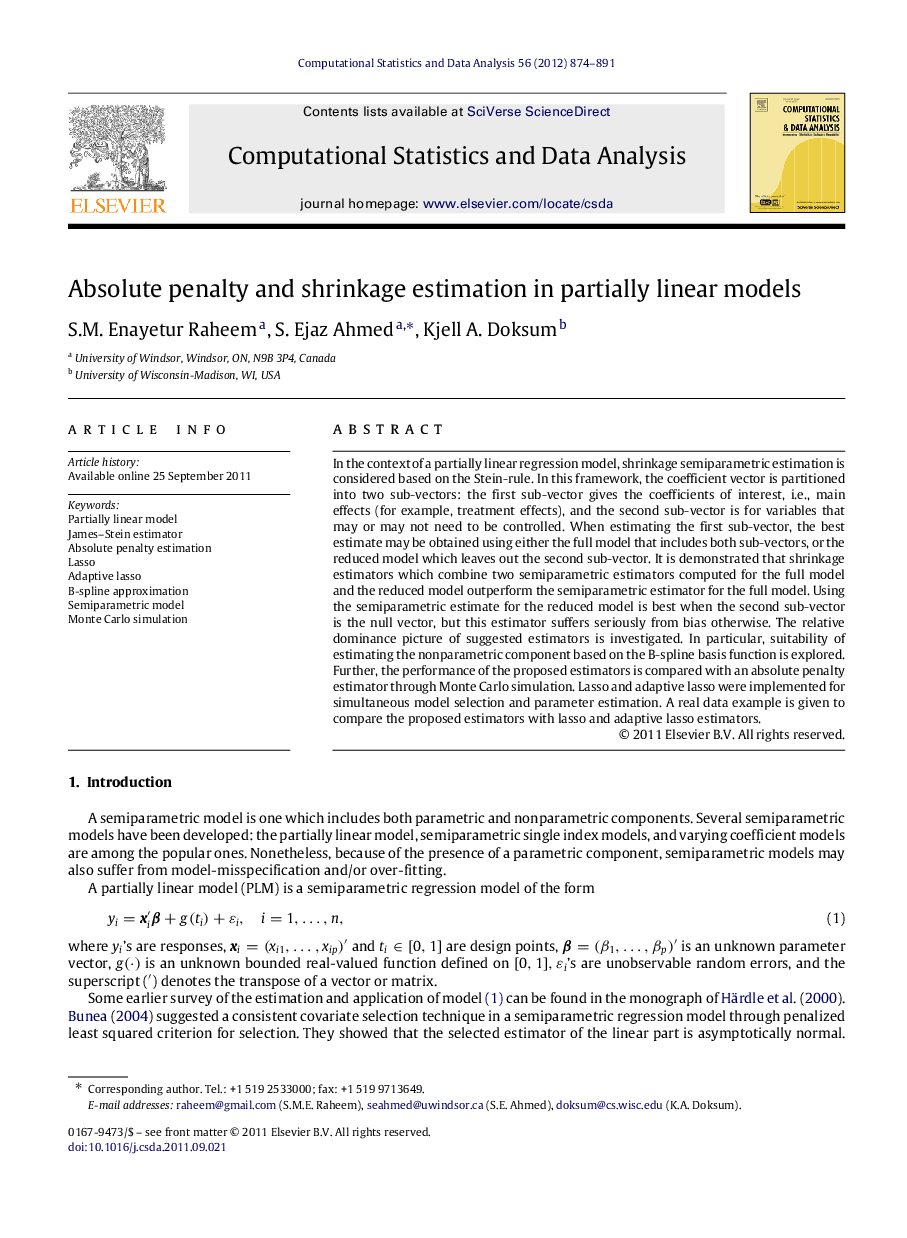
In the context of a partially linear regression model, shrinkage semiparametric estimation is considered based on the Stein-rule. In this framework, the coefficient vector is partitioned into two sub-vectors: the first sub-vector gives the coefficients of interest, i.e., main effects (for example, treatment effects), and the second sub-vector is for variables that may or may not need to be controlled. When estimating the first sub-vector, the best estimate may be obtained using either the full model that includes both sub-vectors, or the reduced model which leaves out the second sub-vector. It is demonstrated that shrinkage estimators which combine two semiparametric estimators computed for the full model and the reduced model outperform the semiparametric estimator for the full model. Using the semiparametric estimate for the reduced model is best when the second sub-vector is the null vector, but this estimator suffers seriously from bias otherwise. The relative dominance picture of suggested estimators is investigated. In particular, suitability of estimating the nonparametric component based on the B-spline basis function is explored. Further, the performance of the proposed estimators is compared with an absolute penalty estimator through Monte Carlo simulation. Lasso and adaptive lasso were implemented for simultaneous model selection and parameter estimation. A real data example is given to compare the proposed estimators with lasso and adaptive lasso estimators.
Journal: Computational Statistics & Data Analysis - Volume 56, Issue 4, 1 April 2012, Pages 874–891