کد مقاله | کد نشریه | سال انتشار | مقاله انگلیسی | نسخه تمام متن |
---|---|---|---|---|
416061 | 681282 | 2009 | 12 صفحه PDF | دانلود رایگان |
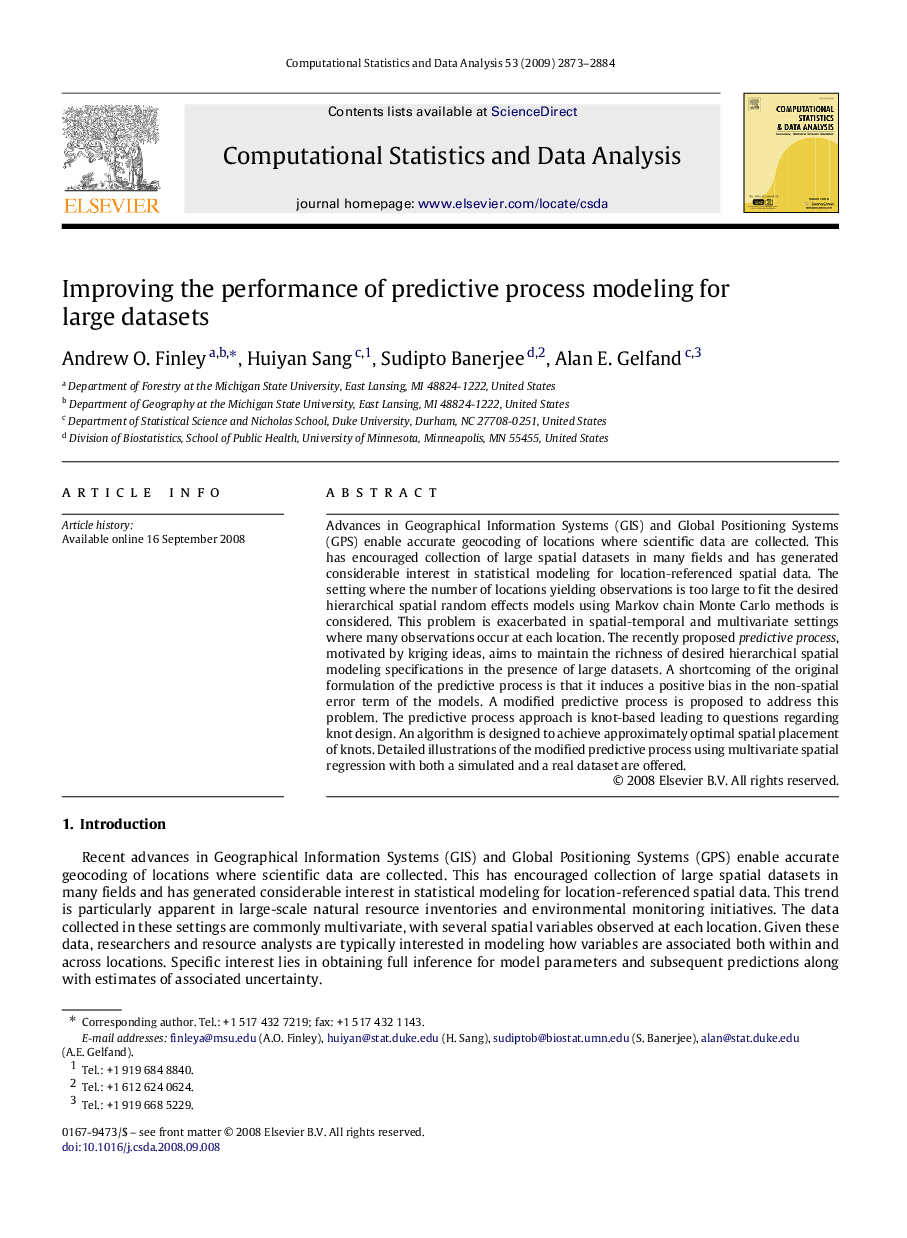
Advances in Geographical Information Systems (GIS) and Global Positioning Systems (GPS) enable accurate geocoding of locations where scientific data are collected. This has encouraged collection of large spatial datasets in many fields and has generated considerable interest in statistical modeling for location-referenced spatial data. The setting where the number of locations yielding observations is too large to fit the desired hierarchical spatial random effects models using Markov chain Monte Carlo methods is considered. This problem is exacerbated in spatial-temporal and multivariate settings where many observations occur at each location. The recently proposed predictive process, motivated by kriging ideas, aims to maintain the richness of desired hierarchical spatial modeling specifications in the presence of large datasets. A shortcoming of the original formulation of the predictive process is that it induces a positive bias in the non-spatial error term of the models. A modified predictive process is proposed to address this problem. The predictive process approach is knot-based leading to questions regarding knot design. An algorithm is designed to achieve approximately optimal spatial placement of knots. Detailed illustrations of the modified predictive process using multivariate spatial regression with both a simulated and a real dataset are offered.
Journal: Computational Statistics & Data Analysis - Volume 53, Issue 8, 15 June 2009, Pages 2873–2884