کد مقاله | کد نشریه | سال انتشار | مقاله انگلیسی | نسخه تمام متن |
---|---|---|---|---|
416332 | 681334 | 2015 | 17 صفحه PDF | دانلود رایگان |
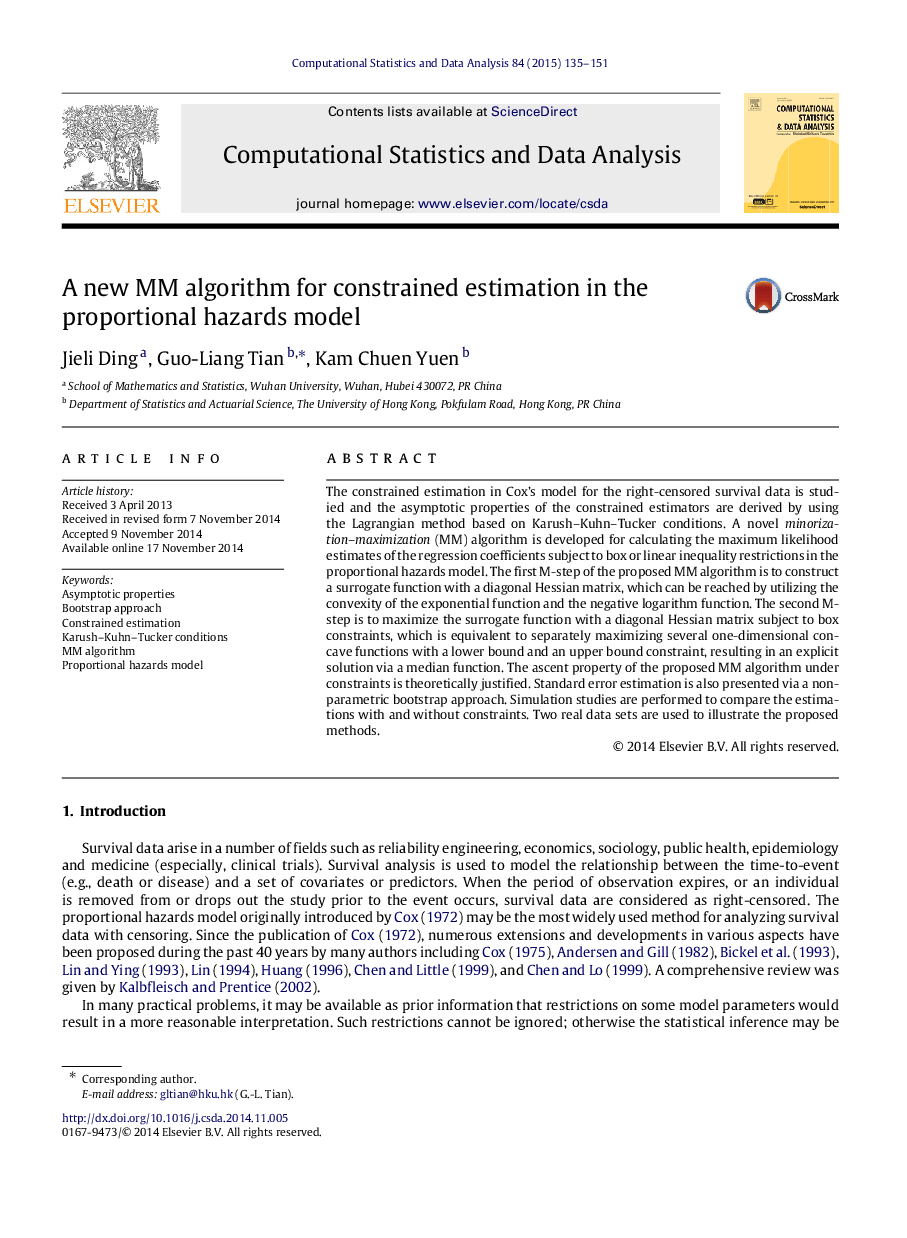
The constrained estimation in Cox’s model for the right-censored survival data is studied and the asymptotic properties of the constrained estimators are derived by using the Lagrangian method based on Karush–Kuhn–Tucker conditions. A novel minorization–maximization (MM) algorithm is developed for calculating the maximum likelihood estimates of the regression coefficients subject to box or linear inequality restrictions in the proportional hazards model. The first M-step of the proposed MM algorithm is to construct a surrogate function with a diagonal Hessian matrix, which can be reached by utilizing the convexity of the exponential function and the negative logarithm function. The second M-step is to maximize the surrogate function with a diagonal Hessian matrix subject to box constraints, which is equivalent to separately maximizing several one-dimensional concave functions with a lower bound and an upper bound constraint, resulting in an explicit solution via a median function. The ascent property of the proposed MM algorithm under constraints is theoretically justified. Standard error estimation is also presented via a non-parametric bootstrap approach. Simulation studies are performed to compare the estimations with and without constraints. Two real data sets are used to illustrate the proposed methods.
Journal: Computational Statistics & Data Analysis - Volume 84, April 2015, Pages 135–151