کد مقاله | کد نشریه | سال انتشار | مقاله انگلیسی | نسخه تمام متن |
---|---|---|---|---|
416395 | 681366 | 2012 | 11 صفحه PDF | دانلود رایگان |
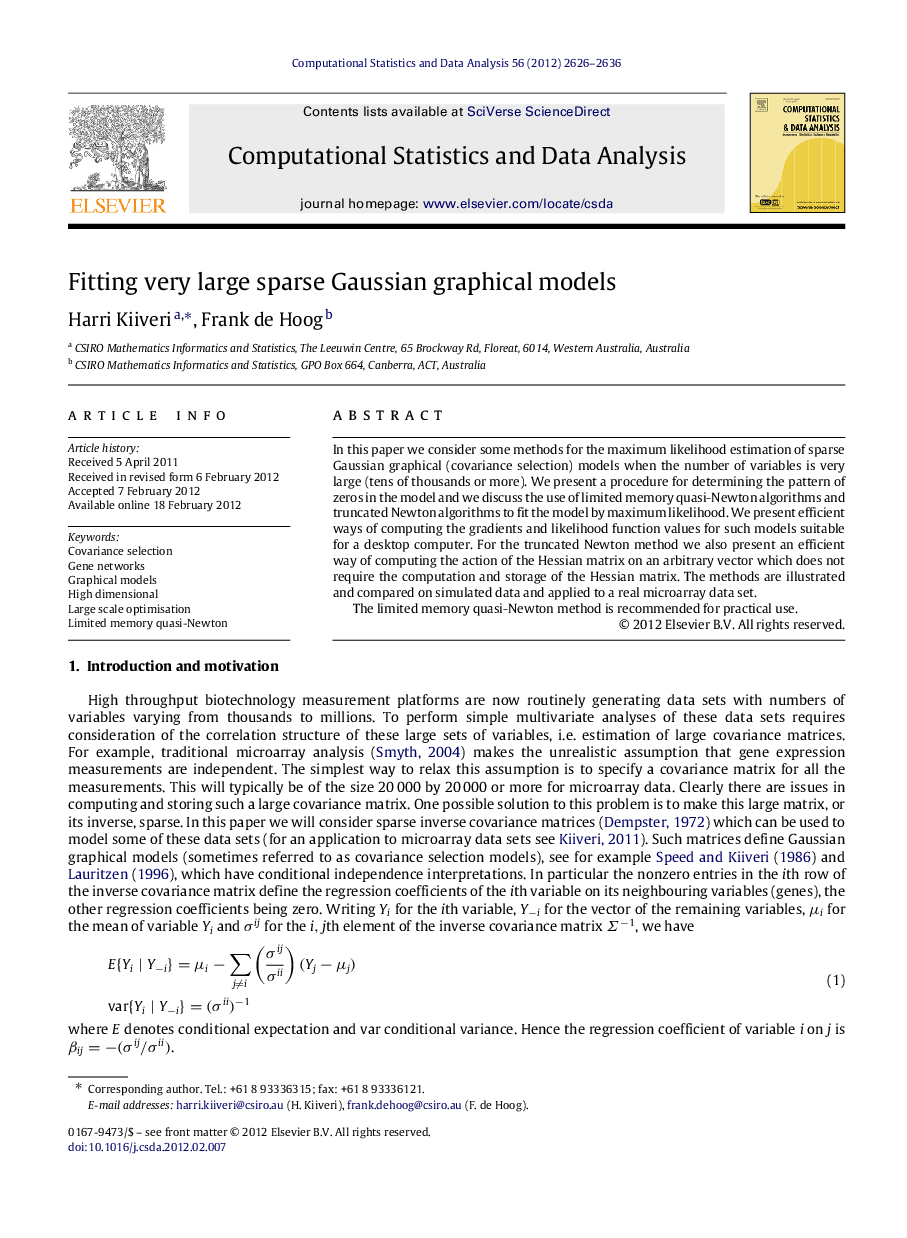
In this paper we consider some methods for the maximum likelihood estimation of sparse Gaussian graphical (covariance selection) models when the number of variables is very large (tens of thousands or more). We present a procedure for determining the pattern of zeros in the model and we discuss the use of limited memory quasi-Newton algorithms and truncated Newton algorithms to fit the model by maximum likelihood. We present efficient ways of computing the gradients and likelihood function values for such models suitable for a desktop computer. For the truncated Newton method we also present an efficient way of computing the action of the Hessian matrix on an arbitrary vector which does not require the computation and storage of the Hessian matrix. The methods are illustrated and compared on simulated data and applied to a real microarray data set.The limited memory quasi-Newton method is recommended for practical use.
Journal: Computational Statistics & Data Analysis - Volume 56, Issue 9, September 2012, Pages 2626–2636