کد مقاله | کد نشریه | سال انتشار | مقاله انگلیسی | نسخه تمام متن |
---|---|---|---|---|
416786 | 681399 | 2013 | 11 صفحه PDF | دانلود رایگان |
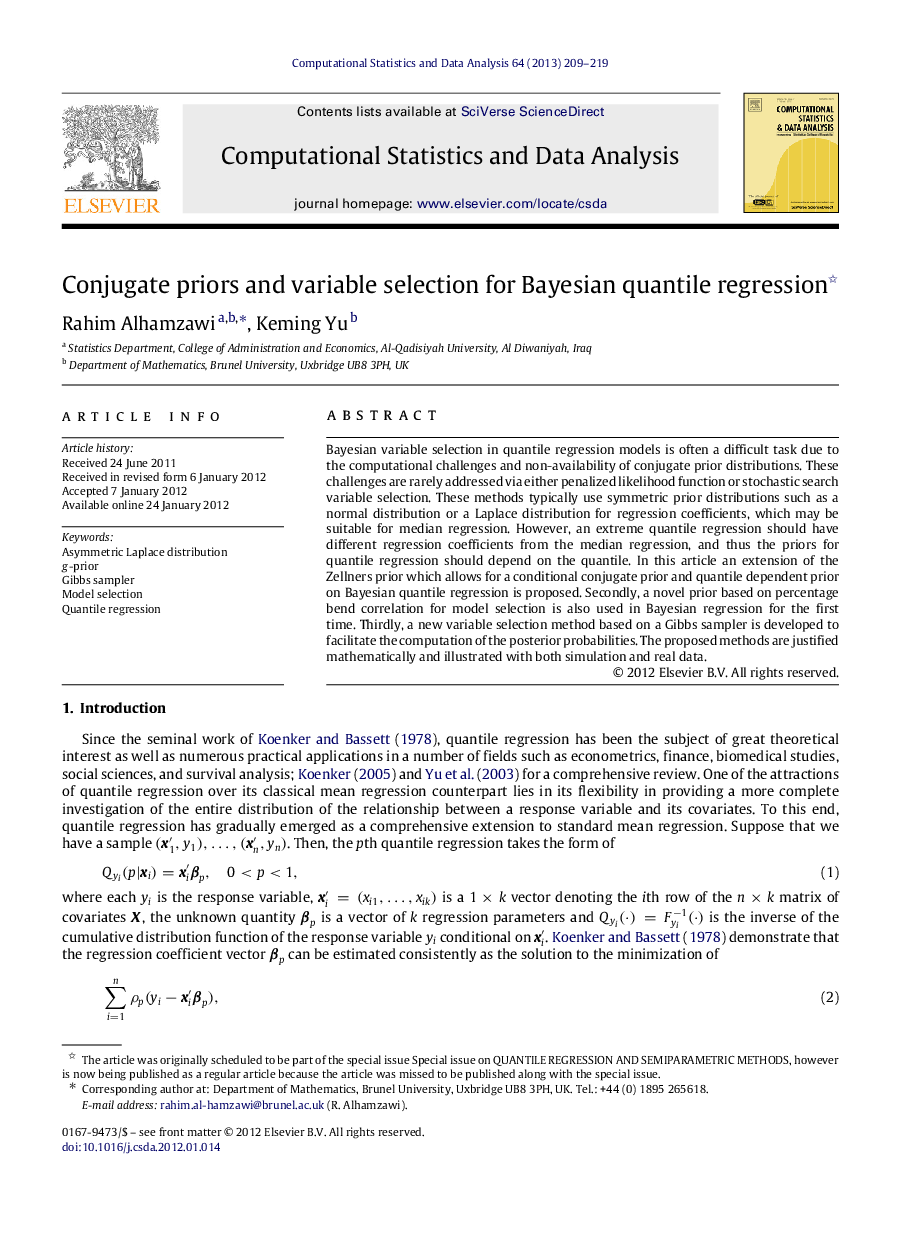
Bayesian variable selection in quantile regression models is often a difficult task due to the computational challenges and non-availability of conjugate prior distributions. These challenges are rarely addressed via either penalized likelihood function or stochastic search variable selection. These methods typically use symmetric prior distributions such as a normal distribution or a Laplace distribution for regression coefficients, which may be suitable for median regression. However, an extreme quantile regression should have different regression coefficients from the median regression, and thus the priors for quantile regression should depend on the quantile. In this article an extension of the Zellners prior which allows for a conditional conjugate prior and quantile dependent prior on Bayesian quantile regression is proposed. Secondly, a novel prior based on percentage bend correlation for model selection is also used in Bayesian regression for the first time. Thirdly, a new variable selection method based on a Gibbs sampler is developed to facilitate the computation of the posterior probabilities. The proposed methods are justified mathematically and illustrated with both simulation and real data.
Journal: Computational Statistics & Data Analysis - Volume 64, August 2013, Pages 209–219