کد مقاله | کد نشریه | سال انتشار | مقاله انگلیسی | نسخه تمام متن |
---|---|---|---|---|
417001 | 681434 | 2010 | 15 صفحه PDF | دانلود رایگان |
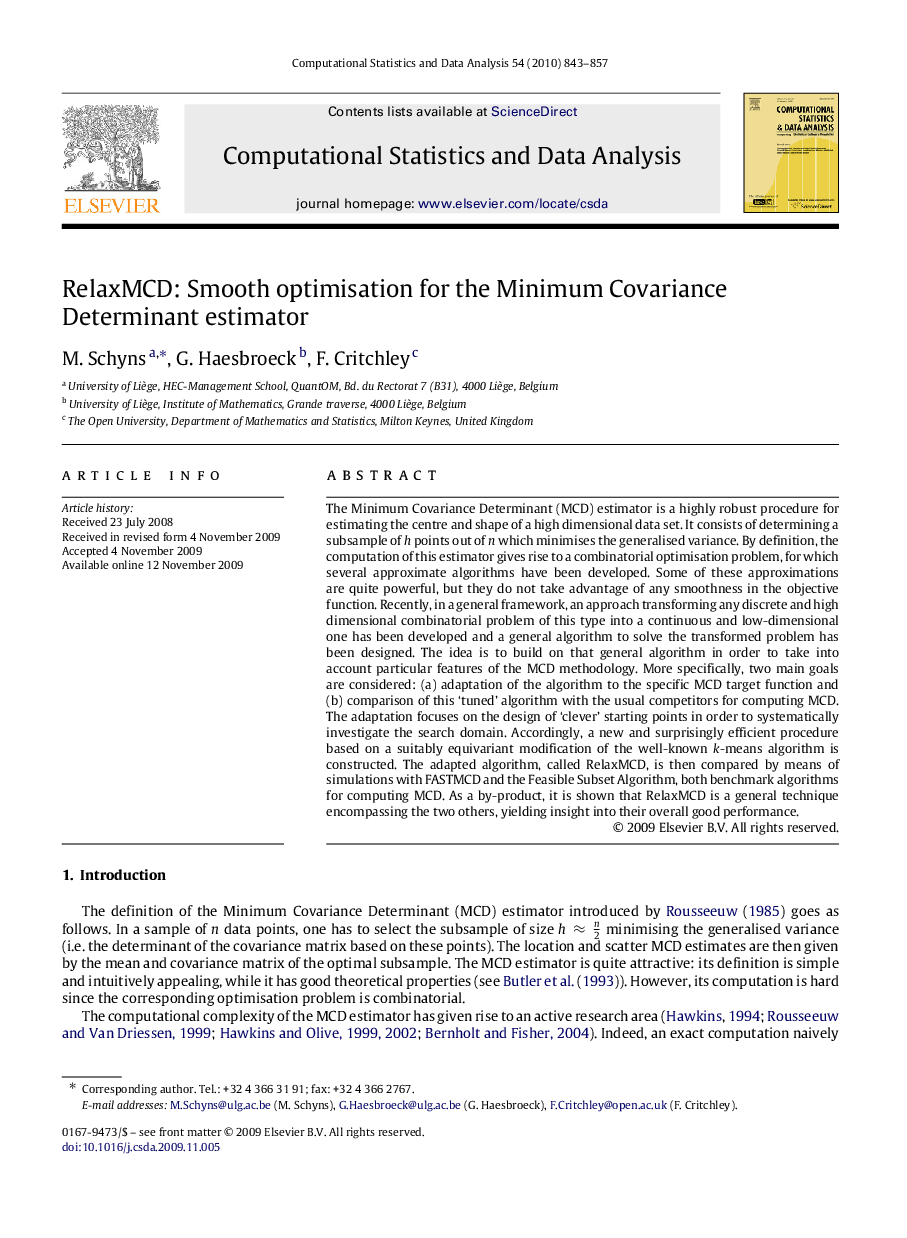
The Minimum Covariance Determinant (MCD) estimator is a highly robust procedure for estimating the centre and shape of a high dimensional data set. It consists of determining a subsample of hh points out of nn which minimises the generalised variance. By definition, the computation of this estimator gives rise to a combinatorial optimisation problem, for which several approximate algorithms have been developed. Some of these approximations are quite powerful, but they do not take advantage of any smoothness in the objective function. Recently, in a general framework, an approach transforming any discrete and high dimensional combinatorial problem of this type into a continuous and low-dimensional one has been developed and a general algorithm to solve the transformed problem has been designed. The idea is to build on that general algorithm in order to take into account particular features of the MCD methodology. More specifically, two main goals are considered: (a) adaptation of the algorithm to the specific MCD target function and (b) comparison of this ‘tuned’ algorithm with the usual competitors for computing MCD. The adaptation focuses on the design of ‘clever’ starting points in order to systematically investigate the search domain. Accordingly, a new and surprisingly efficient procedure based on a suitably equivariant modification of the well-known kk-means algorithm is constructed. The adapted algorithm, called RelaxMCD, is then compared by means of simulations with FASTMCD and the Feasible Subset Algorithm, both benchmark algorithms for computing MCD. As a by-product, it is shown that RelaxMCD is a general technique encompassing the two others, yielding insight into their overall good performance.
Journal: Computational Statistics & Data Analysis - Volume 54, Issue 4, 1 April 2010, Pages 843–857