کد مقاله | کد نشریه | سال انتشار | مقاله انگلیسی | نسخه تمام متن |
---|---|---|---|---|
417139 | 681454 | 2009 | 17 صفحه PDF | دانلود رایگان |
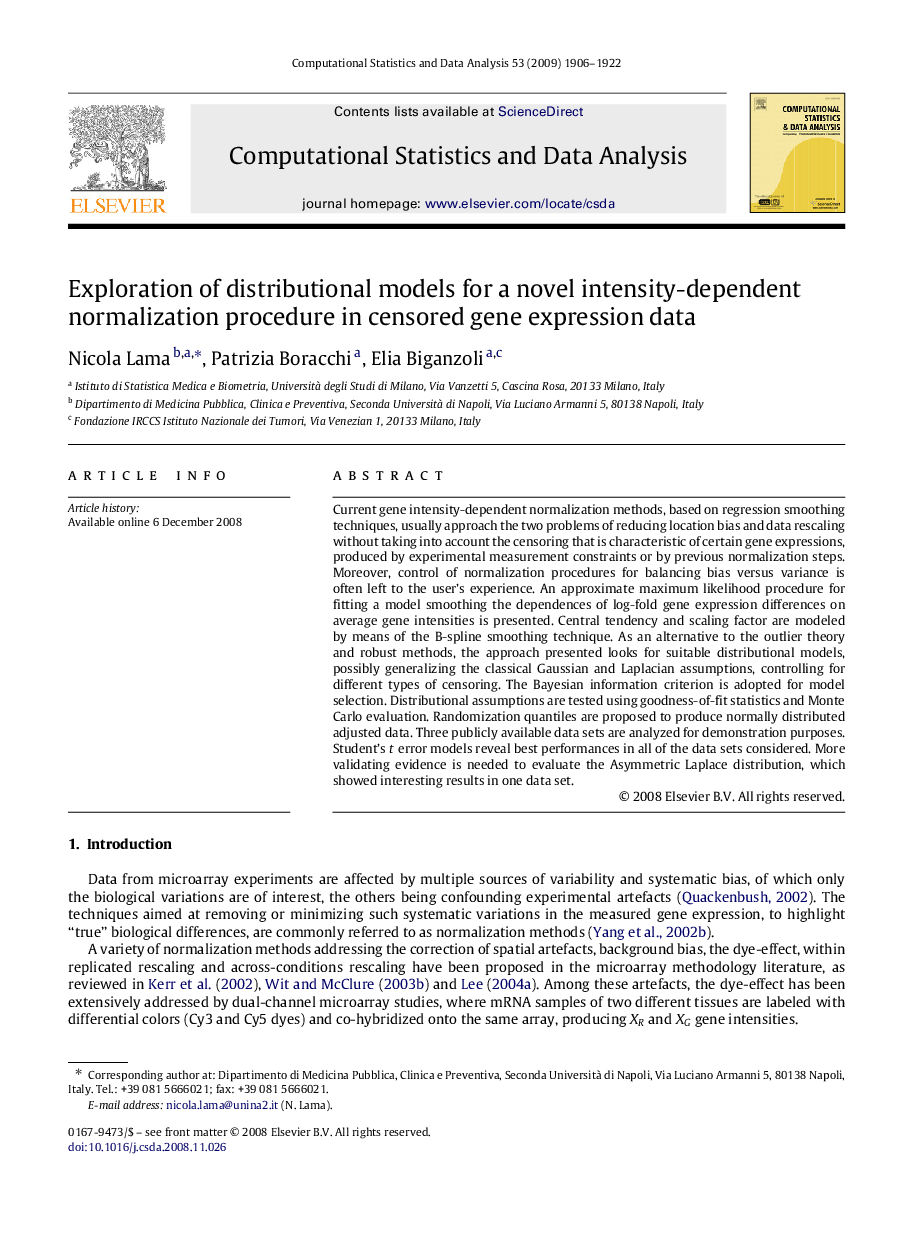
Current gene intensity-dependent normalization methods, based on regression smoothing techniques, usually approach the two problems of reducing location bias and data rescaling without taking into account the censoring that is characteristic of certain gene expressions, produced by experimental measurement constraints or by previous normalization steps. Moreover, control of normalization procedures for balancing bias versus variance is often left to the user’s experience. An approximate maximum likelihood procedure for fitting a model smoothing the dependences of log-fold gene expression differences on average gene intensities is presented. Central tendency and scaling factor are modeled by means of the B-spline smoothing technique. As an alternative to the outlier theory and robust methods, the approach presented looks for suitable distributional models, possibly generalizing the classical Gaussian and Laplacian assumptions, controlling for different types of censoring. The Bayesian information criterion is adopted for model selection. Distributional assumptions are tested using goodness-of-fit statistics and Monte Carlo evaluation. Randomization quantiles are proposed to produce normally distributed adjusted data. Three publicly available data sets are analyzed for demonstration purposes. Student’s tt error models reveal best performances in all of the data sets considered. More validating evidence is needed to evaluate the Asymmetric Laplace distribution, which showed interesting results in one data set.
Journal: Computational Statistics & Data Analysis - Volume 53, Issue 5, 15 March 2009, Pages 1906–1922