کد مقاله | کد نشریه | سال انتشار | مقاله انگلیسی | نسخه تمام متن |
---|---|---|---|---|
417602 | 681544 | 2012 | 10 صفحه PDF | دانلود رایگان |
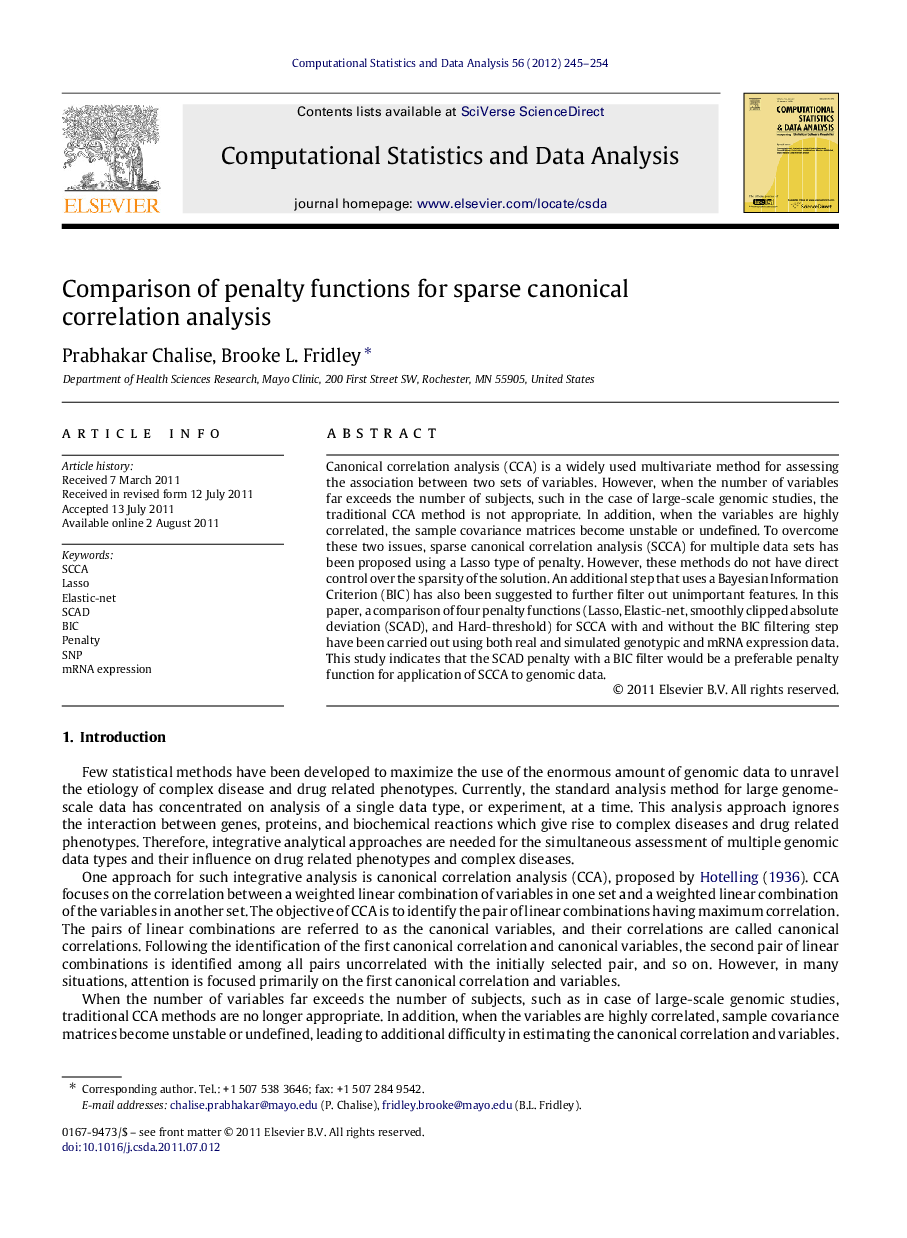
Canonical correlation analysis (CCA) is a widely used multivariate method for assessing the association between two sets of variables. However, when the number of variables far exceeds the number of subjects, such in the case of large-scale genomic studies, the traditional CCA method is not appropriate. In addition, when the variables are highly correlated, the sample covariance matrices become unstable or undefined. To overcome these two issues, sparse canonical correlation analysis (SCCA) for multiple data sets has been proposed using a Lasso type of penalty. However, these methods do not have direct control over the sparsity of the solution. An additional step that uses a Bayesian Information Criterion (BIC) has also been suggested to further filter out unimportant features. In this paper, a comparison of four penalty functions (Lasso, Elastic-net, smoothly clipped absolute deviation (SCAD), and Hard-threshold) for SCCA with and without the BIC filtering step have been carried out using both real and simulated genotypic and mRNA expression data. This study indicates that the SCAD penalty with a BIC filter would be a preferable penalty function for application of SCCA to genomic data.
► We compared various penalty functions for sparse canonical correlation analysis (SCCA).
► We also made an assessment using an additional Bayesian Information Criterion (BIC).
► We found the SCAD penalty with BIC filter preferable for SCCA of genomic data.
Journal: Computational Statistics & Data Analysis - Volume 56, Issue 2, 1 February 2012, Pages 245–254