کد مقاله | کد نشریه | سال انتشار | مقاله انگلیسی | نسخه تمام متن |
---|---|---|---|---|
425271 | 685710 | 2014 | 10 صفحه PDF | دانلود رایگان |
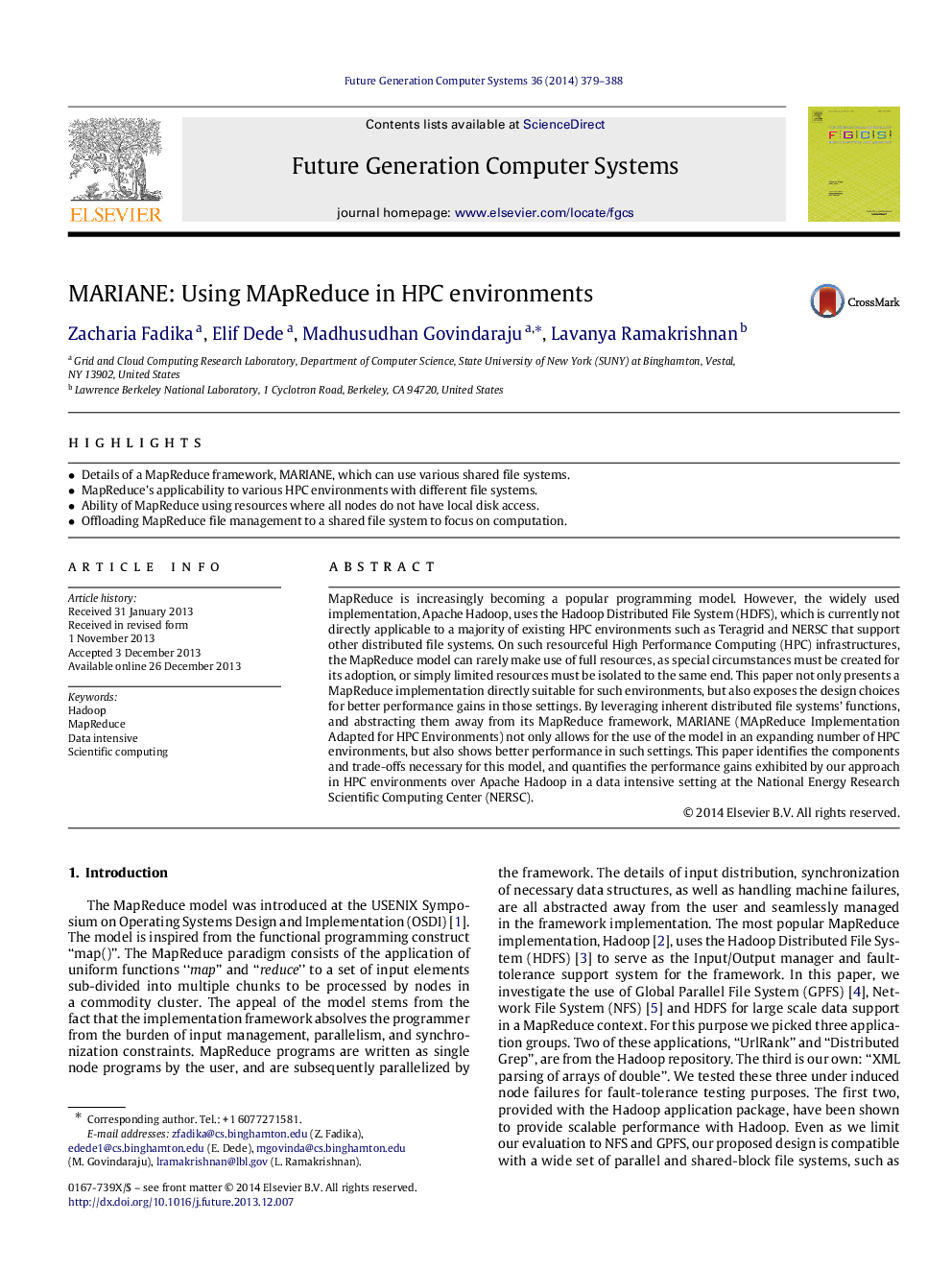
• Details of a MapReduce framework, MARIANE, which can use various shared file systems.
• MapReduce’s applicability to various HPC environments with different file systems.
• Ability of MapReduce using resources where all nodes do not have local disk access.
• Offloading MapReduce file management to a shared file system to focus on computation.
MapReduce is increasingly becoming a popular programming model. However, the widely used implementation, Apache Hadoop, uses the Hadoop Distributed File System (HDFS), which is currently not directly applicable to a majority of existing HPC environments such as Teragrid and NERSC that support other distributed file systems. On such resourceful High Performance Computing (HPC) infrastructures, the MapReduce model can rarely make use of full resources, as special circumstances must be created for its adoption, or simply limited resources must be isolated to the same end. This paper not only presents a MapReduce implementation directly suitable for such environments, but also exposes the design choices for better performance gains in those settings. By leveraging inherent distributed file systems’ functions, and abstracting them away from its MapReduce framework, MARIANE (MApReduce Implementation Adapted for HPC Environments) not only allows for the use of the model in an expanding number of HPC environments, but also shows better performance in such settings. This paper identifies the components and trade-offs necessary for this model, and quantifies the performance gains exhibited by our approach in HPC environments over Apache Hadoop in a data intensive setting at the National Energy Research Scientific Computing Center (NERSC).
Journal: Future Generation Computer Systems - Volume 36, July 2014, Pages 379–388