کد مقاله | کد نشریه | سال انتشار | مقاله انگلیسی | نسخه تمام متن |
---|---|---|---|---|
4375025 | 1617220 | 2012 | 12 صفحه PDF | دانلود رایگان |
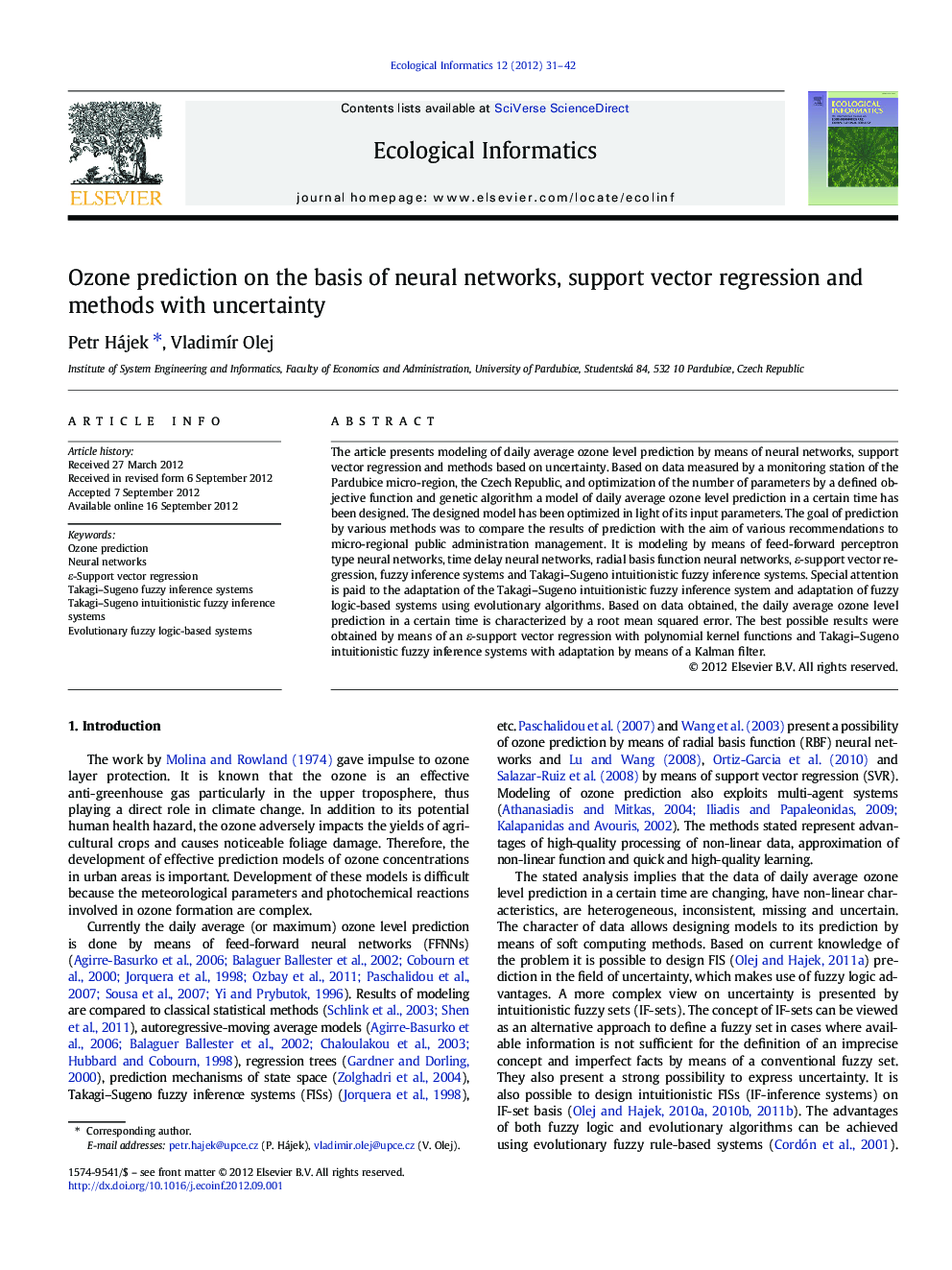
The article presents modeling of daily average ozone level prediction by means of neural networks, support vector regression and methods based on uncertainty. Based on data measured by a monitoring station of the Pardubice micro-region, the Czech Republic, and optimization of the number of parameters by a defined objective function and genetic algorithm a model of daily average ozone level prediction in a certain time has been designed. The designed model has been optimized in light of its input parameters. The goal of prediction by various methods was to compare the results of prediction with the aim of various recommendations to micro-regional public administration management. It is modeling by means of feed-forward perceptron type neural networks, time delay neural networks, radial basis function neural networks, ε-support vector regression, fuzzy inference systems and Takagi–Sugeno intuitionistic fuzzy inference systems. Special attention is paid to the adaptation of the Takagi–Sugeno intuitionistic fuzzy inference system and adaptation of fuzzy logic-based systems using evolutionary algorithms. Based on data obtained, the daily average ozone level prediction in a certain time is characterized by a root mean squared error. The best possible results were obtained by means of an ε-support vector regression with polynomial kernel functions and Takagi–Sugeno intuitionistic fuzzy inference systems with adaptation by means of a Kalman filter.
► We employ various soft computing methods for daily average ozone prediction.
► ε-Support vector regression outperforms neural networks in terms of RMSE.
► Uncertainty in ozone prediction data can be effectively processed using fuzzy logic.
Journal: Ecological Informatics - Volume 12, November 2012, Pages 31–42