کد مقاله | کد نشریه | سال انتشار | مقاله انگلیسی | نسخه تمام متن |
---|---|---|---|---|
441902 | 692013 | 2014 | 10 صفحه PDF | دانلود رایگان |
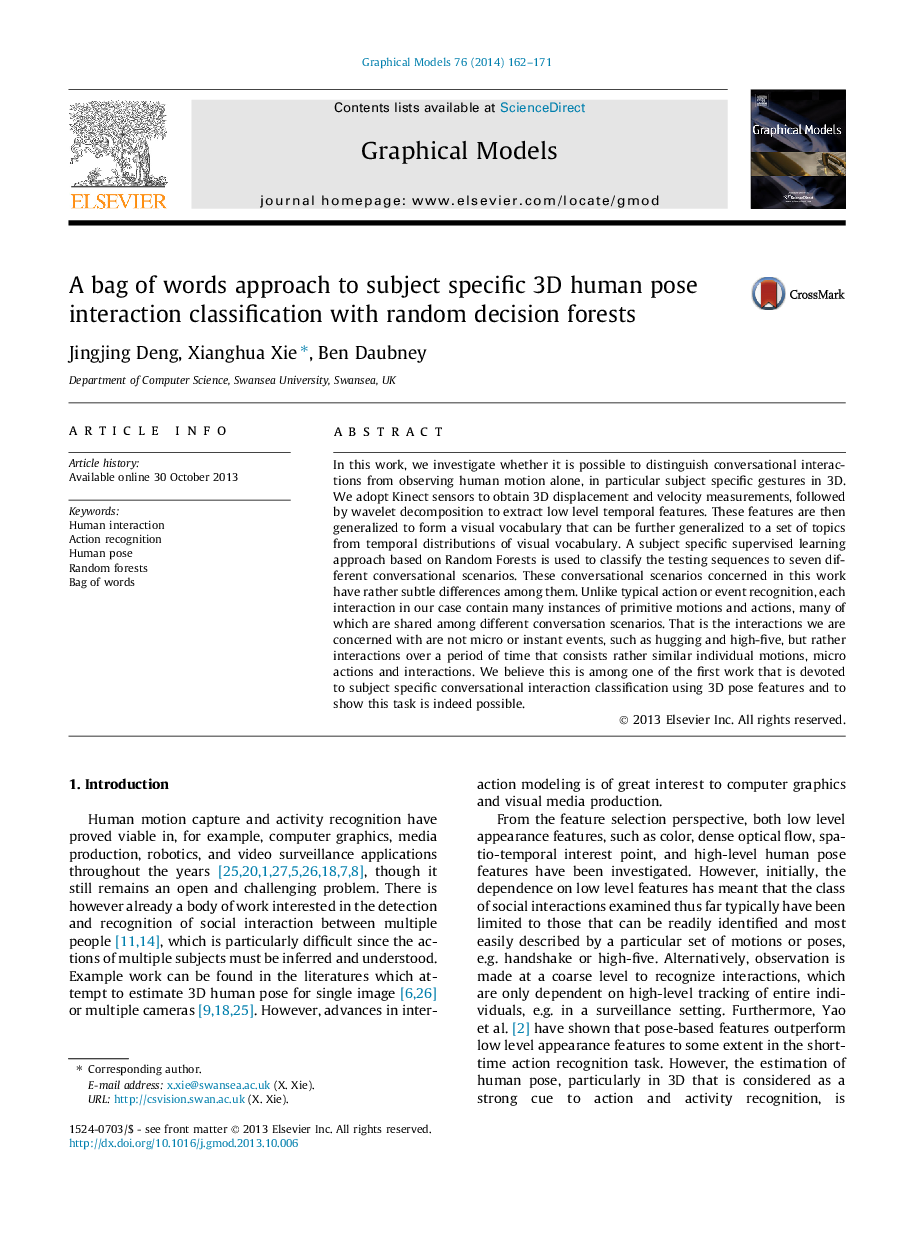
In this work, we investigate whether it is possible to distinguish conversational interactions from observing human motion alone, in particular subject specific gestures in 3D. We adopt Kinect sensors to obtain 3D displacement and velocity measurements, followed by wavelet decomposition to extract low level temporal features. These features are then generalized to form a visual vocabulary that can be further generalized to a set of topics from temporal distributions of visual vocabulary. A subject specific supervised learning approach based on Random Forests is used to classify the testing sequences to seven different conversational scenarios. These conversational scenarios concerned in this work have rather subtle differences among them. Unlike typical action or event recognition, each interaction in our case contain many instances of primitive motions and actions, many of which are shared among different conversation scenarios. That is the interactions we are concerned with are not micro or instant events, such as hugging and high-five, but rather interactions over a period of time that consists rather similar individual motions, micro actions and interactions. We believe this is among one of the first work that is devoted to subject specific conversational interaction classification using 3D pose features and to show this task is indeed possible.
In this work, we investigate whether it is possible to distinguish conversational interactions from observing human motion alone, in particular gestures in 3D. We adopt Kinect sensors to obtain 3D displacement and velocity measurements, followed by wavelet decomposition to extract low level temporal features. These features are then generalized to form a visual vocabulary that can be further generalized to a set of topics from temporal distributions of visual vocabulary. A supervised learning approach based on Random Forests is used to classify the testing sequences to seven different conversational scenarios. These conversational scenarios concerned in this work have rather subtle differences among them. Unlike typical action or event recognition, each interaction in our case contain many instances of primitive motions and actions, many of which are shared among different conversation scenarios. That is the interactions we are concerned with are not micro or instant events, such as hugging and high-five, but rather interactions over a period of time that consists rather similar individual motions, micro actions and interactions. We believe this is among one of the first work that is devoted to conversational interaction classification using 3D pose features and to show this task is indeed possible.The proposed method first extract displacement and velocity measurements from the Kinect output. Wavelet decomposition is then applied to extract low level features from each of those measurements. The wavelet coefficients represent sudden changes in measurements at different temporal scales, and they are treated as the low level motion features. A temporal generalization of those features are then carried out to encapsulate temporal dynamics, which first produces a visual vocabulary and then further generalized them to visual topics through Latent Dirichet Allocation analysis. A discriminative model based on Random Forests is then trained and applied to classify different types of conversational interactions. The flowchart shown in Fig. 1 illustrates the steps from pose measurements, to wavelet analysis, to unsupervised clustering and generalization, and to supervised classification.Figure optionsDownload as PowerPoint slide
Journal: Graphical Models - Volume 76, Issue 3, May 2014, Pages 162–171