کد مقاله | کد نشریه | سال انتشار | مقاله انگلیسی | نسخه تمام متن |
---|---|---|---|---|
4543656 | 1626846 | 2011 | 9 صفحه PDF | دانلود رایگان |
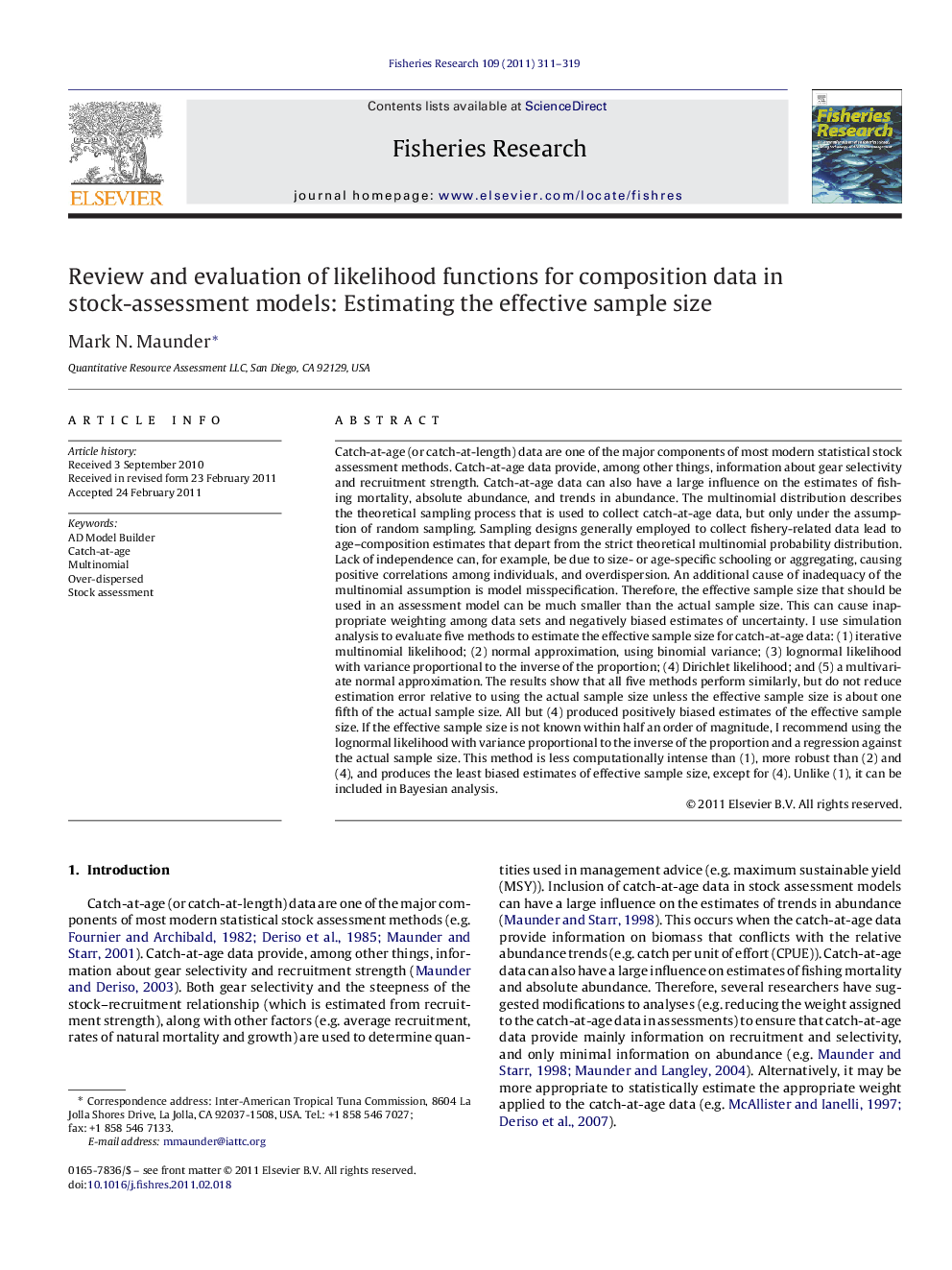
Catch-at-age (or catch-at-length) data are one of the major components of most modern statistical stock assessment methods. Catch-at-age data provide, among other things, information about gear selectivity and recruitment strength. Catch-at-age data can also have a large influence on the estimates of fishing mortality, absolute abundance, and trends in abundance. The multinomial distribution describes the theoretical sampling process that is used to collect catch-at-age data, but only under the assumption of random sampling. Sampling designs generally employed to collect fishery-related data lead to age–composition estimates that depart from the strict theoretical multinomial probability distribution. Lack of independence can, for example, be due to size- or age-specific schooling or aggregating, causing positive correlations among individuals, and overdispersion. An additional cause of inadequacy of the multinomial assumption is model misspecification. Therefore, the effective sample size that should be used in an assessment model can be much smaller than the actual sample size. This can cause inappropriate weighting among data sets and negatively biased estimates of uncertainty. I use simulation analysis to evaluate five methods to estimate the effective sample size for catch-at-age data: (1) iterative multinomial likelihood; (2) normal approximation, using binomial variance; (3) lognormal likelihood with variance proportional to the inverse of the proportion; (4) Dirichlet likelihood; and (5) a multivariate normal approximation. The results show that all five methods perform similarly, but do not reduce estimation error relative to using the actual sample size unless the effective sample size is about one fifth of the actual sample size. All but (4) produced positively biased estimates of the effective sample size. If the effective sample size is not known within half an order of magnitude, I recommend using the lognormal likelihood with variance proportional to the inverse of the proportion and a regression against the actual sample size. This method is less computationally intense than (1), more robust than (2) and (4), and produces the least biased estimates of effective sample size, except for (4). Unlike (1), it can be included in Bayesian analysis.
► Likelihood functions for composition data in stock-assessment models are described and evaluated using simulation analysis.
► Estimating the effective sample size does not reduce estimation error compared to using the actual sample size unless the effective sample size is about one fifth of the actual sample size.
► I recommend using the lognormal likelihood with variance proportional to the inverse of the proportion and a regression against the actual sample size.
Journal: Fisheries Research - Volume 109, Issues 2–3, May 2011, Pages 311–319