کد مقاله | کد نشریه | سال انتشار | مقاله انگلیسی | نسخه تمام متن |
---|---|---|---|---|
456583 | 695741 | 2009 | 21 صفحه PDF | دانلود رایگان |
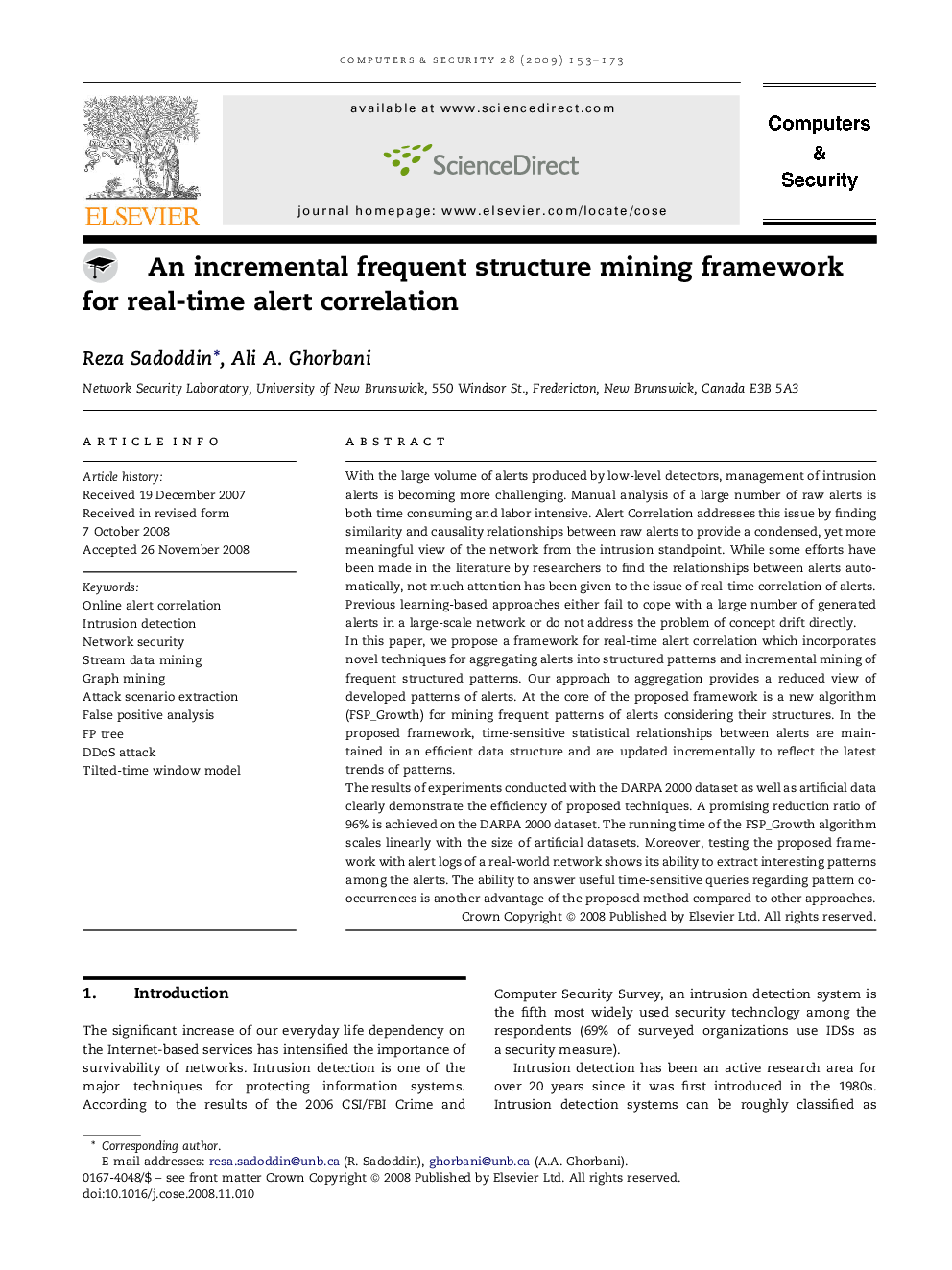
With the large volume of alerts produced by low-level detectors, management of intrusion alerts is becoming more challenging. Manual analysis of a large number of raw alerts is both time consuming and labor intensive. Alert Correlation addresses this issue by finding similarity and causality relationships between raw alerts to provide a condensed, yet more meaningful view of the network from the intrusion standpoint. While some efforts have been made in the literature by researchers to find the relationships between alerts automatically, not much attention has been given to the issue of real-time correlation of alerts. Previous learning-based approaches either fail to cope with a large number of generated alerts in a large-scale network or do not address the problem of concept drift directly.In this paper, we propose a framework for real-time alert correlation which incorporates novel techniques for aggregating alerts into structured patterns and incremental mining of frequent structured patterns. Our approach to aggregation provides a reduced view of developed patterns of alerts. At the core of the proposed framework is a new algorithm (FSP_Growth) for mining frequent patterns of alerts considering their structures. In the proposed framework, time-sensitive statistical relationships between alerts are maintained in an efficient data structure and are updated incrementally to reflect the latest trends of patterns.The results of experiments conducted with the DARPA 2000 dataset as well as artificial data clearly demonstrate the efficiency of proposed techniques. A promising reduction ratio of 96% is achieved on the DARPA 2000 dataset. The running time of the FSP_Growth algorithm scales linearly with the size of artificial datasets. Moreover, testing the proposed framework with alert logs of a real-world network shows its ability to extract interesting patterns among the alerts. The ability to answer useful time-sensitive queries regarding pattern co-occurrences is another advantage of the proposed method compared to other approaches.
Journal: Computers & Security - Volume 28, Issues 3–4, May–June 2009, Pages 153–173