کد مقاله | کد نشریه | سال انتشار | مقاله انگلیسی | نسخه تمام متن |
---|---|---|---|---|
461952 | 696649 | 2010 | 11 صفحه PDF | دانلود رایگان |
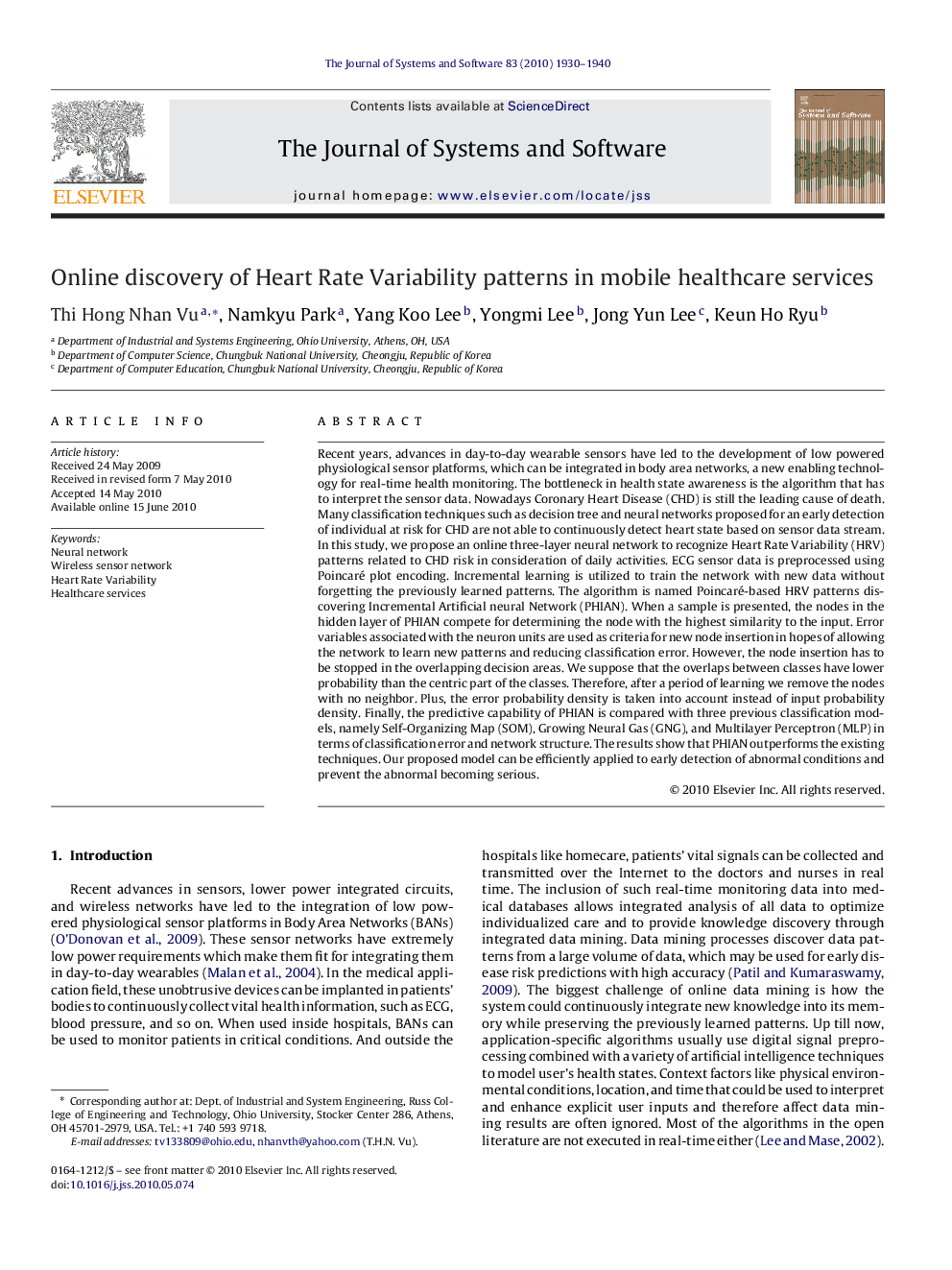
Recent years, advances in day-to-day wearable sensors have led to the development of low powered physiological sensor platforms, which can be integrated in body area networks, a new enabling technology for real-time health monitoring. The bottleneck in health state awareness is the algorithm that has to interpret the sensor data. Nowadays Coronary Heart Disease (CHD) is still the leading cause of death. Many classification techniques such as decision tree and neural networks proposed for an early detection of individual at risk for CHD are not able to continuously detect heart state based on sensor data stream. In this study, we propose an online three-layer neural network to recognize Heart Rate Variability (HRV) patterns related to CHD risk in consideration of daily activities. ECG sensor data is preprocessed using Poincaré plot encoding. Incremental learning is utilized to train the network with new data without forgetting the previously learned patterns. The algorithm is named Poincaré-based HRV patterns discovering Incremental Artificial neural Network (PHIAN). When a sample is presented, the nodes in the hidden layer of PHIAN compete for determining the node with the highest similarity to the input. Error variables associated with the neuron units are used as criteria for new node insertion in hopes of allowing the network to learn new patterns and reducing classification error. However, the node insertion has to be stopped in the overlapping decision areas. We suppose that the overlaps between classes have lower probability than the centric part of the classes. Therefore, after a period of learning we remove the nodes with no neighbor. Plus, the error probability density is taken into account instead of input probability density. Finally, the predictive capability of PHIAN is compared with three previous classification models, namely Self-Organizing Map (SOM), Growing Neural Gas (GNG), and Multilayer Perceptron (MLP) in terms of classification error and network structure. The results show that PHIAN outperforms the existing techniques. Our proposed model can be efficiently applied to early detection of abnormal conditions and prevent the abnormal becoming serious.
Journal: Journal of Systems and Software - Volume 83, Issue 10, October 2010, Pages 1930–1940