کد مقاله | کد نشریه | سال انتشار | مقاله انگلیسی | نسخه تمام متن |
---|---|---|---|---|
483756 | 1446476 | 2015 | 11 صفحه PDF | دانلود رایگان |
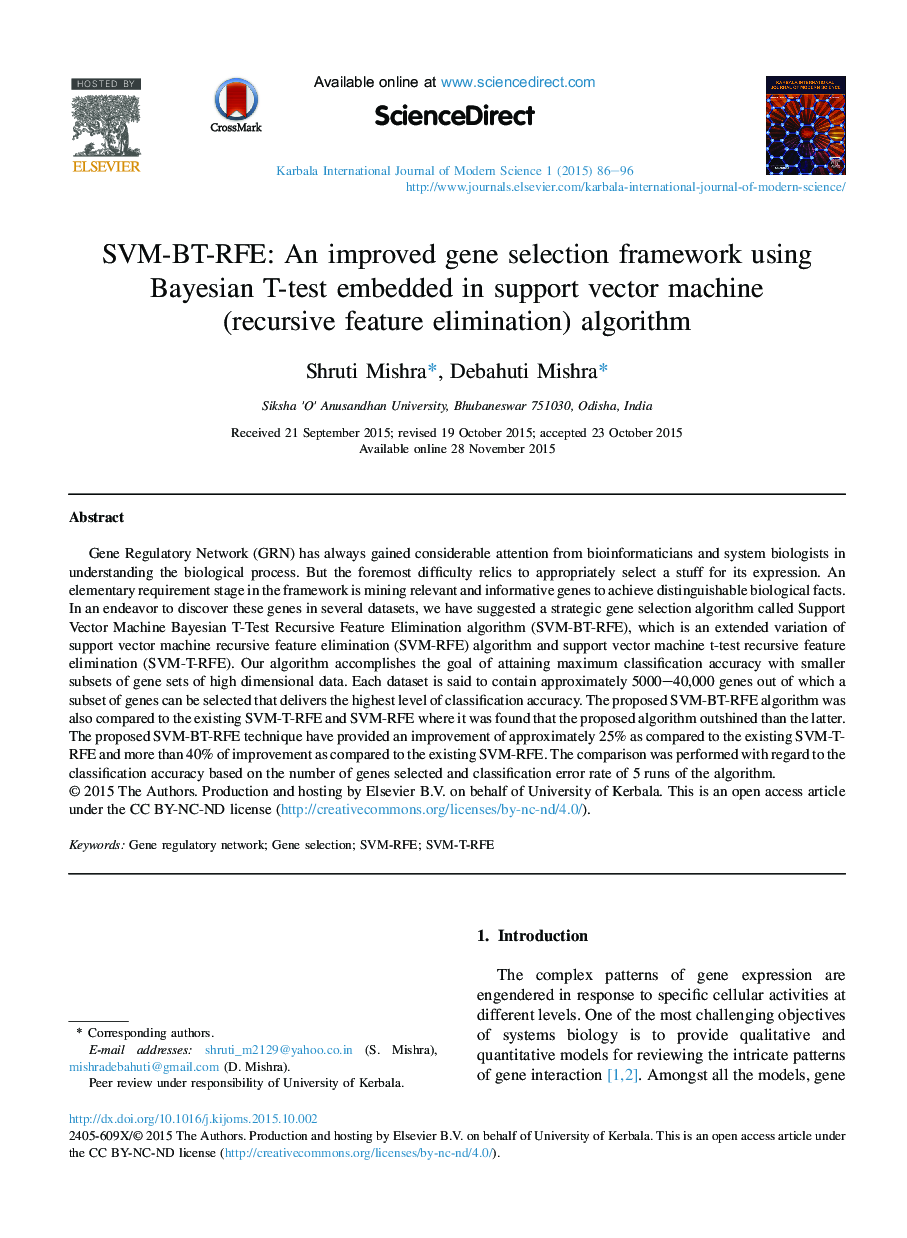
Gene Regulatory Network (GRN) has always gained considerable attention from bioinformaticians and system biologists in understanding the biological process. But the foremost difficulty relics to appropriately select a stuff for its expression. An elementary requirement stage in the framework is mining relevant and informative genes to achieve distinguishable biological facts. In an endeavor to discover these genes in several datasets, we have suggested a strategic gene selection algorithm called Support Vector Machine Bayesian T-Test Recursive Feature Elimination algorithm (SVM-BT-RFE), which is an extended variation of support vector machine recursive feature elimination (SVM-RFE) algorithm and support vector machine t-test recursive feature elimination (SVM-T-RFE). Our algorithm accomplishes the goal of attaining maximum classification accuracy with smaller subsets of gene sets of high dimensional data. Each dataset is said to contain approximately 5000–40,000 genes out of which a subset of genes can be selected that delivers the highest level of classification accuracy. The proposed SVM-BT-RFE algorithm was also compared to the existing SVM-T-RFE and SVM-RFE where it was found that the proposed algorithm outshined than the latter. The proposed SVM-BT-RFE technique have provided an improvement of approximately 25% as compared to the existing SVM-T-RFE and more than 40% of improvement as compared to the existing SVM-RFE. The comparison was performed with regard to the classification accuracy based on the number of genes selected and classification error rate of 5 runs of the algorithm.
Journal: Karbala International Journal of Modern Science - Volume 1, Issue 2, October 2015, Pages 86–96