کد مقاله | کد نشریه | سال انتشار | مقاله انگلیسی | نسخه تمام متن |
---|---|---|---|---|
493560 | 721930 | 2006 | 10 صفحه PDF | دانلود رایگان |
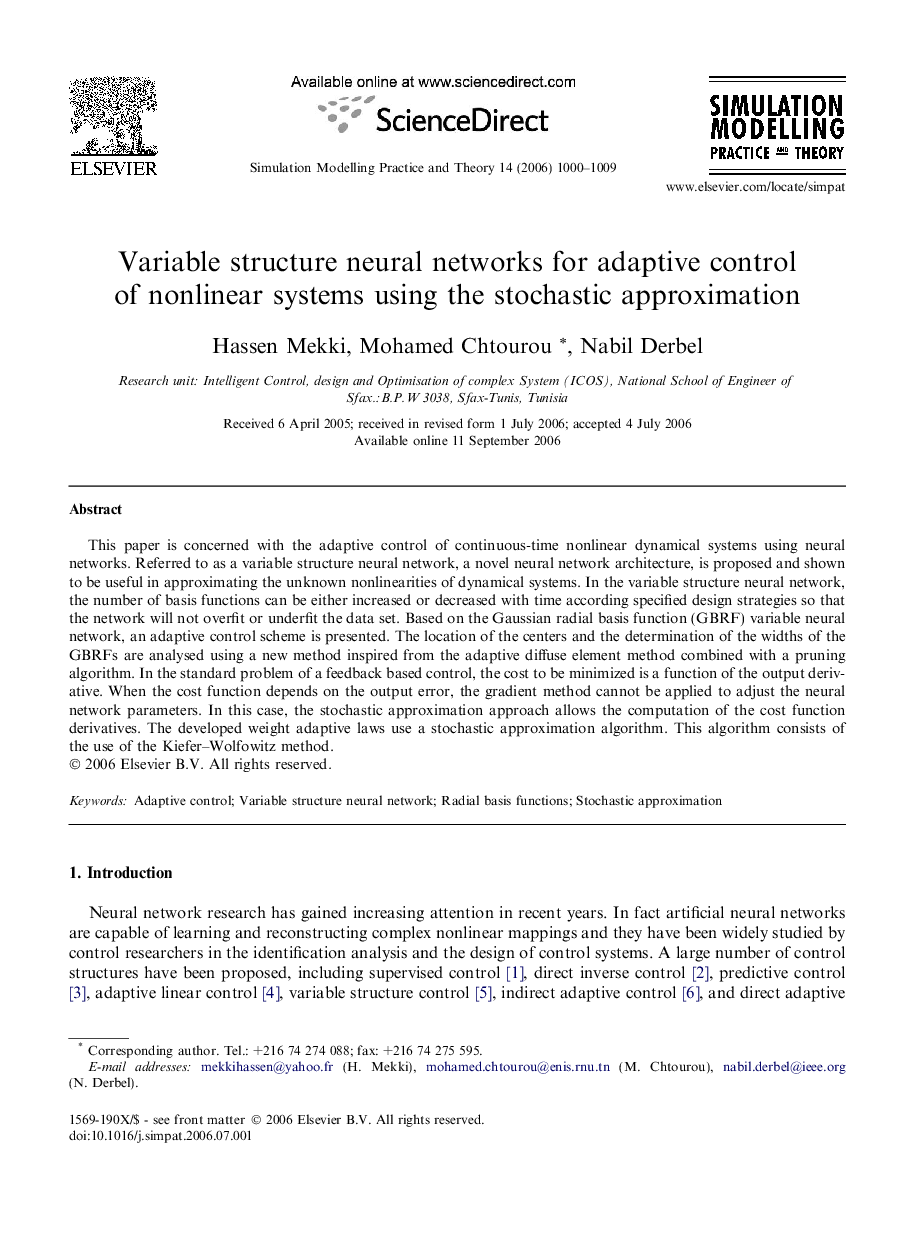
This paper is concerned with the adaptive control of continuous-time nonlinear dynamical systems using neural networks. Referred to as a variable structure neural network, a novel neural network architecture, is proposed and shown to be useful in approximating the unknown nonlinearities of dynamical systems. In the variable structure neural network, the number of basis functions can be either increased or decreased with time according specified design strategies so that the network will not overfit or underfit the data set. Based on the Gaussian radial basis function (GBRF) variable neural network, an adaptive control scheme is presented. The location of the centers and the determination of the widths of the GBRFs are analysed using a new method inspired from the adaptive diffuse element method combined with a pruning algorithm. In the standard problem of a feedback based control, the cost to be minimized is a function of the output derivative. When the cost function depends on the output error, the gradient method cannot be applied to adjust the neural network parameters. In this case, the stochastic approximation approach allows the computation of the cost function derivatives. The developed weight adaptive laws use a stochastic approximation algorithm. This algorithm consists of the use of the Kiefer–Wolfowitz method.
Journal: Simulation Modelling Practice and Theory - Volume 14, Issue 7, October 2006, Pages 1000–1009