کد مقاله | کد نشریه | سال انتشار | مقاله انگلیسی | نسخه تمام متن |
---|---|---|---|---|
4946087 | 1439270 | 2017 | 22 صفحه PDF | دانلود رایگان |
عنوان انگلیسی مقاله ISI
Parameter-less Auto-weighted multiple graph regularized Nonnegative Matrix Factorization for data representation
دانلود مقاله + سفارش ترجمه
دانلود مقاله ISI انگلیسی
رایگان برای ایرانیان
موضوعات مرتبط
مهندسی و علوم پایه
مهندسی کامپیوتر
هوش مصنوعی
پیش نمایش صفحه اول مقاله
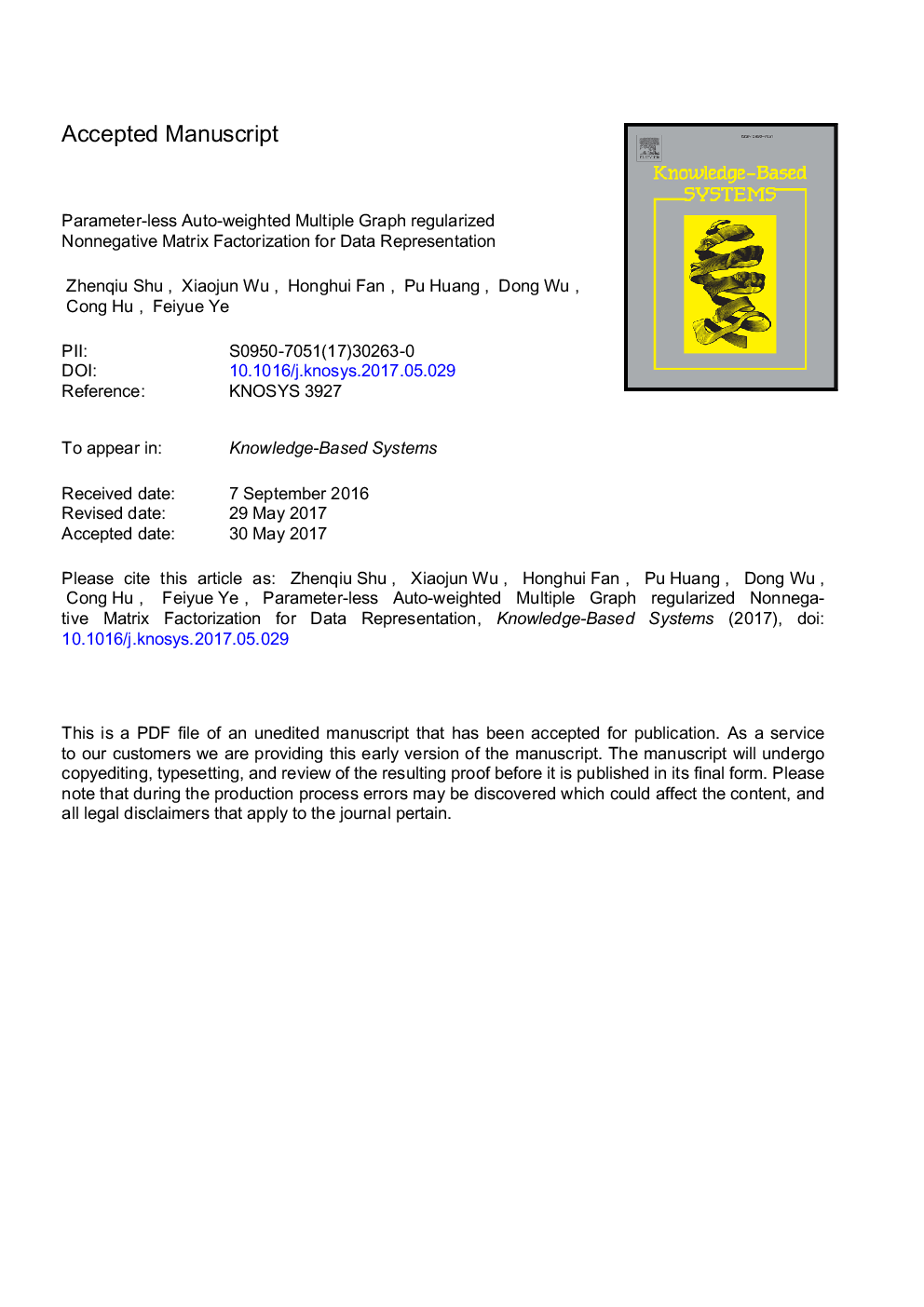
چکیده انگلیسی
Recently, multiple graph regularizer based methods have shown promising performances in data representation. However, the parameter choice of the regularizer is crucial to the performance of clustering and its optimal value changes for different real datasets. To deal with this problem, we propose a novel method called Parameter-less Auto-weighted Multiple Graph regularized Nonnegative Matrix Factorization (PAMGNMF) in this paper. PAMGNMF employs the linear combination of multiple simple graphs to approximate the manifold structure of data as previous methods do. Moreover, the proposed method can automatically learn an optimal weight for each graph without introducing an additive parameter. Therefore, the proposed PAMGNMF method is easily applied to practical problems. Extensive experimental results on different real-world datasets have demonstrated that the proposed method achieves better performance than the state-of-the-art approaches.
ناشر
Database: Elsevier - ScienceDirect (ساینس دایرکت)
Journal: Knowledge-Based Systems - Volume 131, 1 September 2017, Pages 105-112
Journal: Knowledge-Based Systems - Volume 131, 1 September 2017, Pages 105-112
نویسندگان
Zhenqiu Shu, Xiaojun Wu, Honghui Fan, Pu Huang, Dong Wu, Cong Hu, Feiyue Ye,