کد مقاله | کد نشریه | سال انتشار | مقاله انگلیسی | نسخه تمام متن |
---|---|---|---|---|
4946611 | 1439410 | 2017 | 30 صفحه PDF | دانلود رایگان |
عنوان انگلیسی مقاله ISI
Nonredundant sparse feature extraction using autoencoders with receptive fields clustering
دانلود مقاله + سفارش ترجمه
دانلود مقاله ISI انگلیسی
رایگان برای ایرانیان
کلمات کلیدی
موضوعات مرتبط
مهندسی و علوم پایه
مهندسی کامپیوتر
هوش مصنوعی
پیش نمایش صفحه اول مقاله
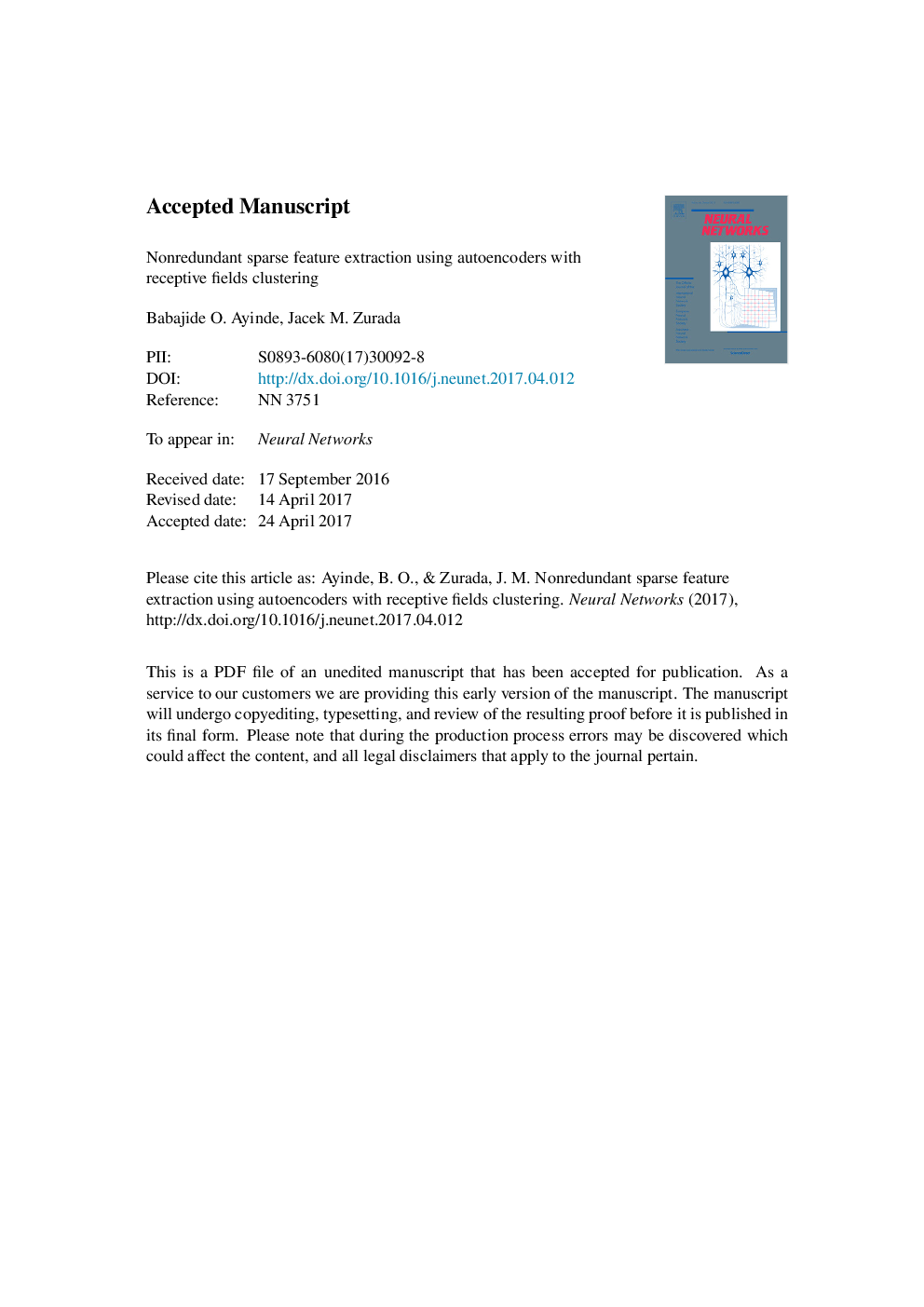
چکیده انگلیسی
This paper proposes new techniques for data representation in the context of deep learning using agglomerative clustering. Existing autoencoder-based data representation techniques tend to produce a number of encoding and decoding receptive fields of layered autoencoders that are duplicative, thereby leading to extraction of similar features, thus resulting in filtering redundancy. We propose a way to address this problem and show that such redundancy can be eliminated. This yields smaller networks and produces unique receptive fields that extract distinct features. It is also shown that autoencoders with nonnegativity constraints on weights are capable of extracting fewer redundant features than conventional sparse autoencoders. The concept is illustrated using conventional sparse autoencoder and nonnegativity-constrained autoencoders with MNIST digits recognition, NORB normalized-uniform object data and Yale face dataset.
ناشر
Database: Elsevier - ScienceDirect (ساینس دایرکت)
Journal: Neural Networks - Volume 93, September 2017, Pages 99-109
Journal: Neural Networks - Volume 93, September 2017, Pages 99-109
نویسندگان
Babajide O. Ayinde, Jacek M. Zurada,