کد مقاله | کد نشریه | سال انتشار | مقاله انگلیسی | نسخه تمام متن |
---|---|---|---|---|
494682 | 862802 | 2016 | 11 صفحه PDF | دانلود رایگان |
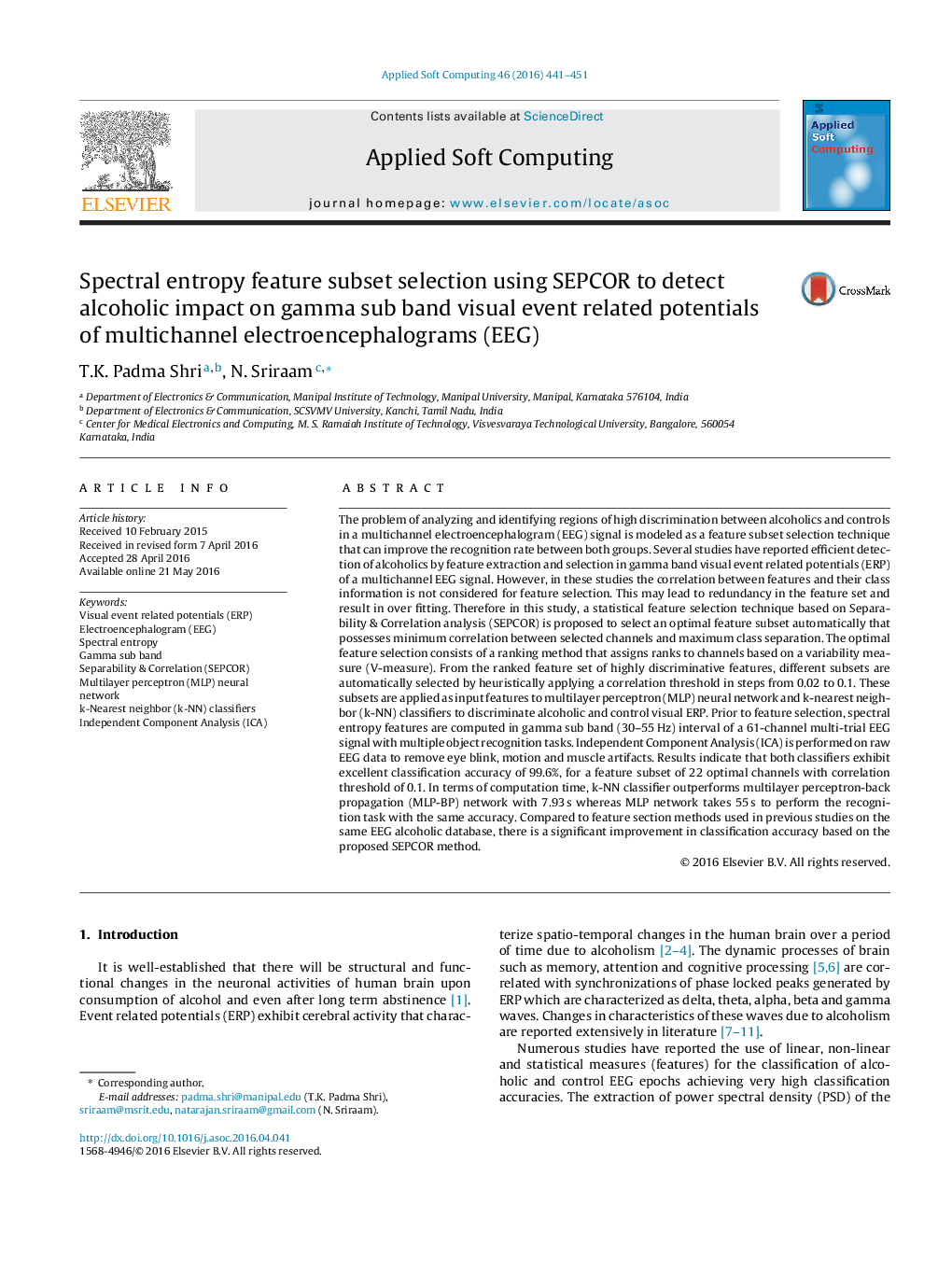
• The proposed feature selection method is based on maximization of class separability and minimum correlation between selected features.
• ICA is applied on Physionet alcoholic EEG database to separate pure EEG epochs from artifacts such as eye blink, body and cranial muscle movements.
• As per our knowledge, this is the first attempt to remove eye blink and muscle activity (> 30 Hz) from the EEG epochs of the alcoholic EEG database.
• Results show better classification accuracy compared to previous studieson the same Physionet alcoholic EEG database.
• The normalized spectral entropy feature is used for extensive analysis in this study for the first time.
The problem of analyzing and identifying regions of high discrimination between alcoholics and controls in a multichannel electroencephalogram (EEG) signal is modeled as a feature subset selection technique that can improve the recognition rate between both groups. Several studies have reported efficient detection of alcoholics by feature extraction and selection in gamma band visual event related potentials (ERP) of a multichannel EEG signal. However, in these studies the correlation between features and their class information is not considered for feature selection. This may lead to redundancy in the feature set and result in over fitting. Therefore in this study, a statistical feature selection technique based on Separability & Correlation analysis (SEPCOR) is proposed to select an optimal feature subset automatically that possesses minimum correlation between selected channels and maximum class separation. The optimal feature selection consists of a ranking method that assigns ranks to channels based on a variability measure (V-measure). From the ranked feature set of highly discriminative features, different subsets are automatically selected by heuristically applying a correlation threshold in steps from 0.02 to 0.1. These subsets are applied as input features to multilayer perceptron (MLP) neural network and k-nearest neighbor (k-NN) classifiers to discriminate alcoholic and control visual ERP. Prior to feature selection, spectral entropy features are computed in gamma sub band (30–55 Hz) interval of a 61-channel multi-trial EEG signal with multiple object recognition tasks. Independent Component Analysis (ICA) is performed on raw EEG data to remove eye blink, motion and muscle artifacts. Results indicate that both classifiers exhibit excellent classification accuracy of 99.6%, for a feature subset of 22 optimal channels with correlation threshold of 0.1. In terms of computation time, k-NN classifier outperforms multilayer perceptron-back propagation (MLP-BP) network with 7.93 s whereas MLP network takes 55 s to perform the recognition task with the same accuracy. Compared to feature section methods used in previous studies on the same EEG alcoholic database, there is a significant improvement in classification accuracy based on the proposed SEPCOR method.
Figure optionsDownload as PowerPoint slide
Journal: Applied Soft Computing - Volume 46, September 2016, Pages 441–451