کد مقاله | کد نشریه | سال انتشار | مقاله انگلیسی | نسخه تمام متن |
---|---|---|---|---|
495159 | 862817 | 2015 | 13 صفحه PDF | دانلود رایگان |
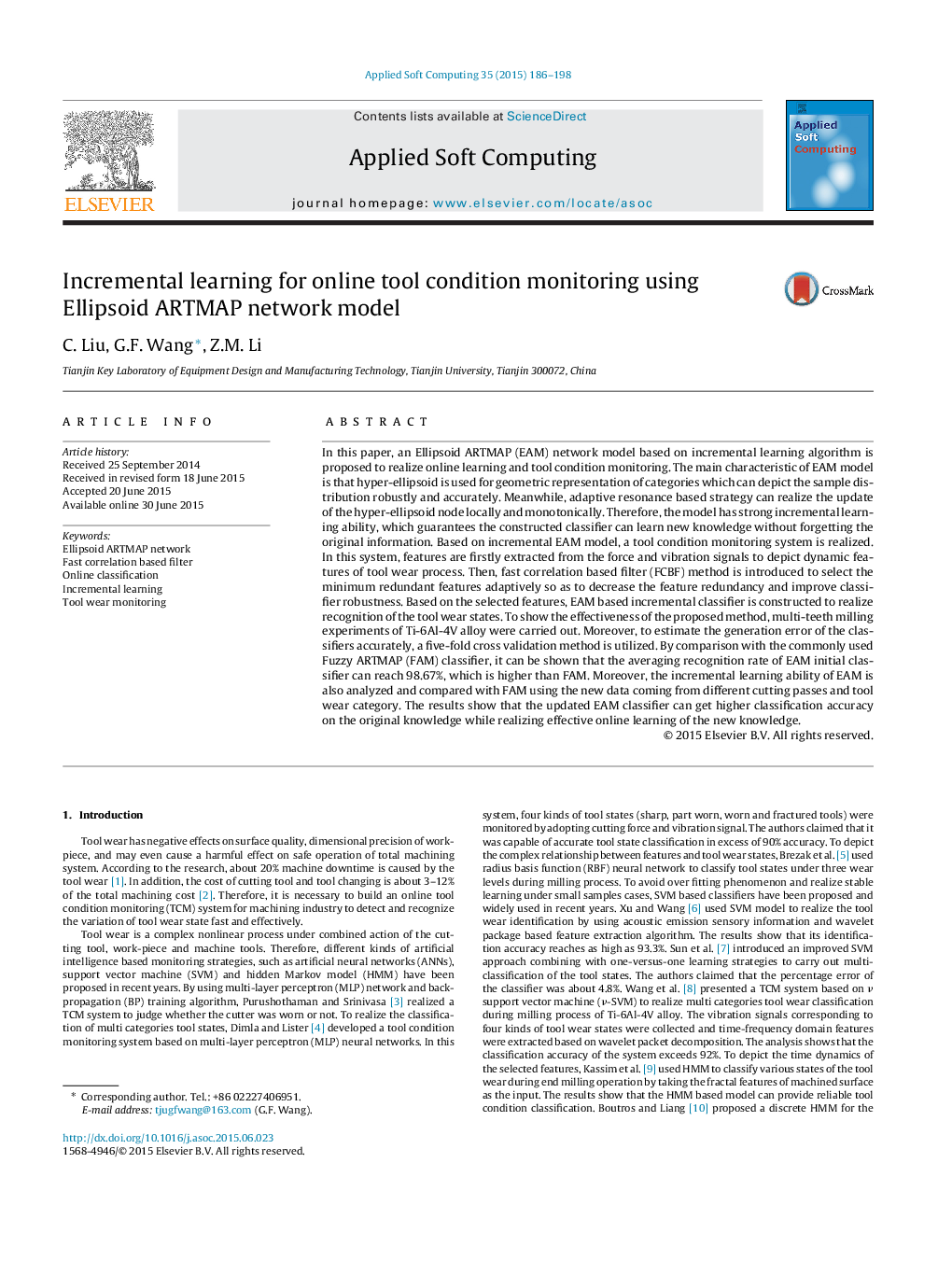
• EAM based incremental learning algorithm is presented.
• FCBF is adopted to select the minimum redundant features adaptively.
• EAM gets higher accuracy rate than FAM.
• EAM can learn new data incrementally without forgetting the original knowledge.
In this paper, an Ellipsoid ARTMAP (EAM) network model based on incremental learning algorithm is proposed to realize online learning and tool condition monitoring. The main characteristic of EAM model is that hyper-ellipsoid is used for geometric representation of categories which can depict the sample distribution robustly and accurately. Meanwhile, adaptive resonance based strategy can realize the update of the hyper-ellipsoid node locally and monotonically. Therefore, the model has strong incremental learning ability, which guarantees the constructed classifier can learn new knowledge without forgetting the original information. Based on incremental EAM model, a tool condition monitoring system is realized. In this system, features are firstly extracted from the force and vibration signals to depict dynamic features of tool wear process. Then, fast correlation based filter (FCBF) method is introduced to select the minimum redundant features adaptively so as to decrease the feature redundancy and improve classifier robustness. Based on the selected features, EAM based incremental classifier is constructed to realize recognition of the tool wear states. To show the effectiveness of the proposed method, multi-teeth milling experiments of Ti-6Al-4V alloy were carried out. Moreover, to estimate the generation error of the classifiers accurately, a five-fold cross validation method is utilized. By comparison with the commonly used Fuzzy ARTMAP (FAM) classifier, it can be shown that the averaging recognition rate of EAM initial classifier can reach 98.67%, which is higher than FAM. Moreover, the incremental learning ability of EAM is also analyzed and compared with FAM using the new data coming from different cutting passes and tool wear category. The results show that the updated EAM classifier can get higher classification accuracy on the original knowledge while realizing effective online learning of the new knowledge.
Figure optionsDownload as PowerPoint slide
Journal: Applied Soft Computing - Volume 35, October 2015, Pages 186–198