کد مقاله | کد نشریه | سال انتشار | مقاله انگلیسی | نسخه تمام متن |
---|---|---|---|---|
495724 | 862835 | 2013 | 9 صفحه PDF | دانلود رایگان |
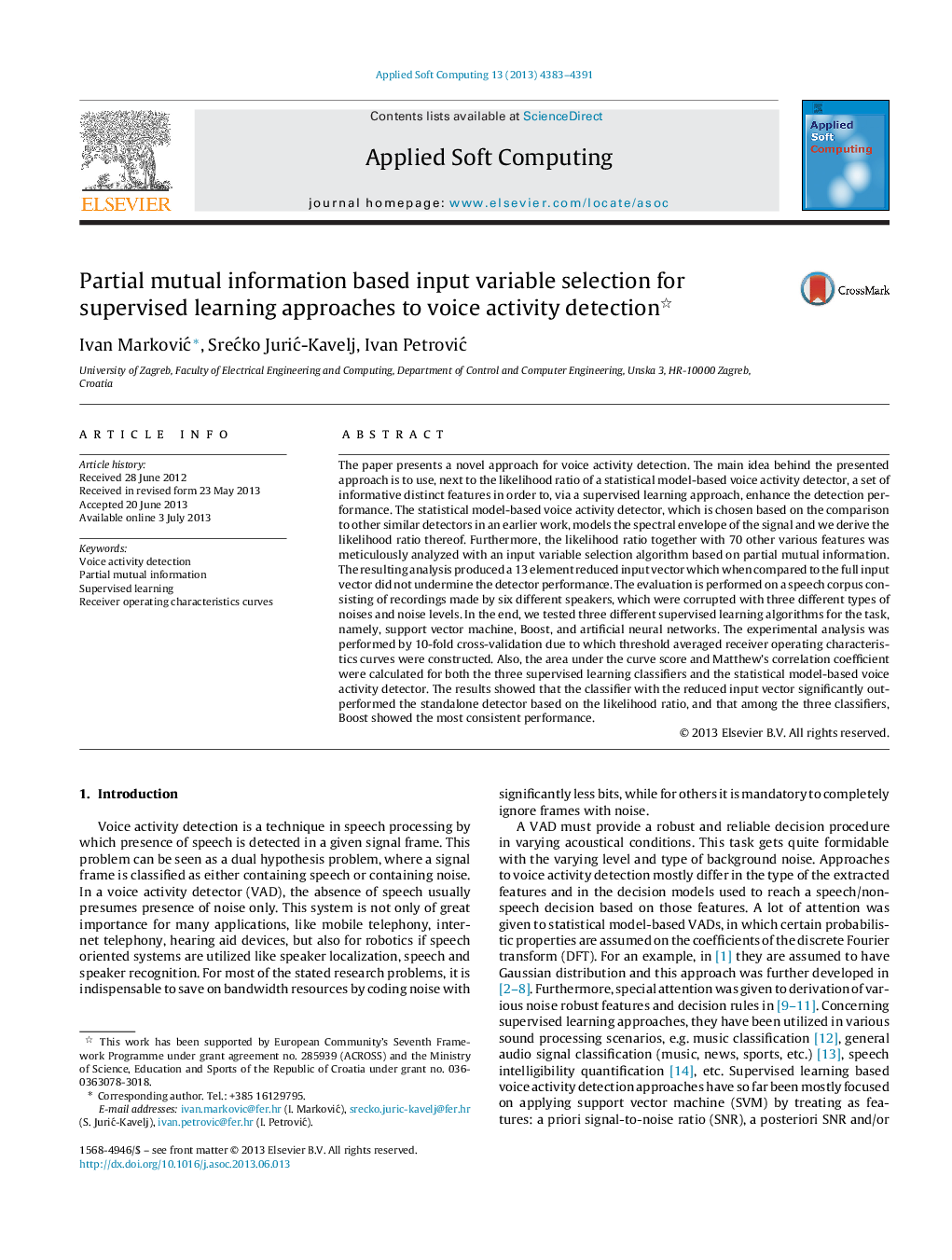
• Voice activity detector based on likelihood ratio and many distinct features.
• Substantial reduction in input vector dimension without undermining the detection performance.
• A supervised learning classifier significantly outperformed the statistical model-based detector.
• Among SVM, neural network and Boost, the latter showed more consistent performance.
The paper presents a novel approach for voice activity detection. The main idea behind the presented approach is to use, next to the likelihood ratio of a statistical model-based voice activity detector, a set of informative distinct features in order to, via a supervised learning approach, enhance the detection performance. The statistical model-based voice activity detector, which is chosen based on the comparison to other similar detectors in an earlier work, models the spectral envelope of the signal and we derive the likelihood ratio thereof. Furthermore, the likelihood ratio together with 70 other various features was meticulously analyzed with an input variable selection algorithm based on partial mutual information. The resulting analysis produced a 13 element reduced input vector which when compared to the full input vector did not undermine the detector performance. The evaluation is performed on a speech corpus consisting of recordings made by six different speakers, which were corrupted with three different types of noises and noise levels. In the end, we tested three different supervised learning algorithms for the task, namely, support vector machine, Boost, and artificial neural networks. The experimental analysis was performed by 10-fold cross-validation due to which threshold averaged receiver operating characteristics curves were constructed. Also, the area under the curve score and Matthew's correlation coefficient were calculated for both the three supervised learning classifiers and the statistical model-based voice activity detector. The results showed that the classifier with the reduced input vector significantly outperformed the standalone detector based on the likelihood ratio, and that among the three classifiers, Boost showed the most consistent performance.
Figure optionsDownload as PowerPoint slide
Journal: Applied Soft Computing - Volume 13, Issue 11, November 2013, Pages 4383–4391