کد مقاله | کد نشریه | سال انتشار | مقاله انگلیسی | نسخه تمام متن |
---|---|---|---|---|
495972 | 862845 | 2013 | 6 صفحه PDF | دانلود رایگان |
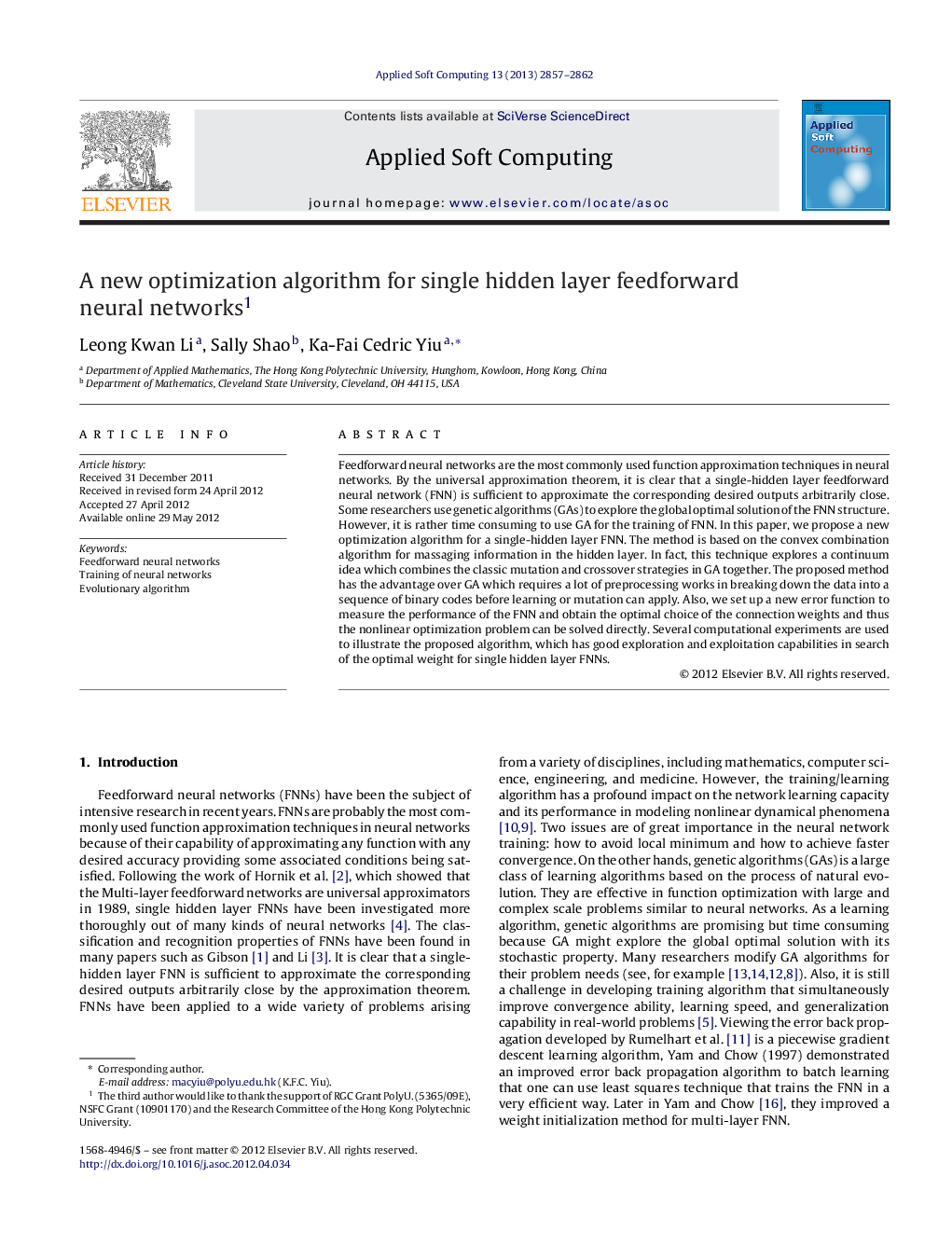
Feedforward neural networks are the most commonly used function approximation techniques in neural networks. By the universal approximation theorem, it is clear that a single-hidden layer feedforward neural network (FNN) is sufficient to approximate the corresponding desired outputs arbitrarily close. Some researchers use genetic algorithms (GAs) to explore the global optimal solution of the FNN structure. However, it is rather time consuming to use GA for the training of FNN. In this paper, we propose a new optimization algorithm for a single-hidden layer FNN. The method is based on the convex combination algorithm for massaging information in the hidden layer. In fact, this technique explores a continuum idea which combines the classic mutation and crossover strategies in GA together. The proposed method has the advantage over GA which requires a lot of preprocessing works in breaking down the data into a sequence of binary codes before learning or mutation can apply. Also, we set up a new error function to measure the performance of the FNN and obtain the optimal choice of the connection weights and thus the nonlinear optimization problem can be solved directly. Several computational experiments are used to illustrate the proposed algorithm, which has good exploration and exploitation capabilities in search of the optimal weight for single hidden layer FNNs.
Convergence of the proposed optimization algorithm for single hidden layer feedforward neural networks. Figure optionsDownload as PowerPoint slideHighlights
► We study the single hidden layer feedforward neural networks.
► We propose a new optimization algorithm to perform training instead of the genetic algorithm.
► We show the proposed algorithm achieves better and faster convergence.
Journal: Applied Soft Computing - Volume 13, Issue 5, May 2013, Pages 2857–2862