کد مقاله | کد نشریه | سال انتشار | مقاله انگلیسی | نسخه تمام متن |
---|---|---|---|---|
496026 | 862847 | 2013 | 20 صفحه PDF | دانلود رایگان |
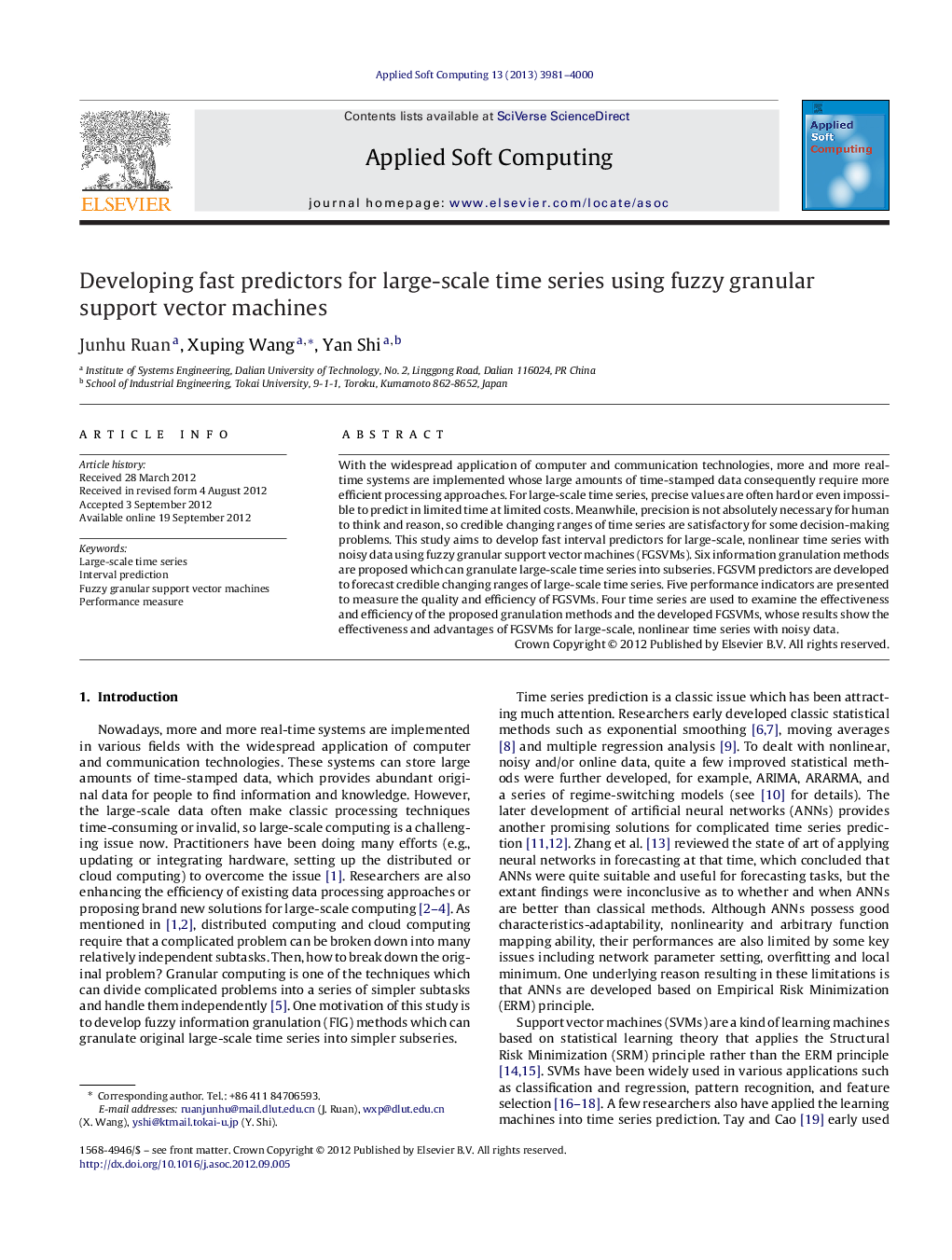
With the widespread application of computer and communication technologies, more and more real-time systems are implemented whose large amounts of time-stamped data consequently require more efficient processing approaches. For large-scale time series, precise values are often hard or even impossible to predict in limited time at limited costs. Meanwhile, precision is not absolutely necessary for human to think and reason, so credible changing ranges of time series are satisfactory for some decision-making problems. This study aims to develop fast interval predictors for large-scale, nonlinear time series with noisy data using fuzzy granular support vector machines (FGSVMs). Six information granulation methods are proposed which can granulate large-scale time series into subseries. FGSVM predictors are developed to forecast credible changing ranges of large-scale time series. Five performance indicators are presented to measure the quality and efficiency of FGSVMs. Four time series are used to examine the effectiveness and efficiency of the proposed granulation methods and the developed FGSVMs, whose results show the effectiveness and advantages of FGSVMs for large-scale, nonlinear time series with noisy data.
Figure optionsDownload as PowerPoint slideHighlights
► Six information granulation methods are proposed to granulate large-scale time series.
► FGSVMs are developed for large-scale, nonlinear time series with noisy data.
► Five performance indicators are presented to measure the quality and efficiency of FGSVM predictors.
► The length of granulation window has important impacts on the number of granulated windows and the granules in each window.
► The introduction of fuzzy information granulation is helpful for classic SVMs to build time-saving and robust predictors.
Journal: Applied Soft Computing - Volume 13, Issue 9, September 2013, Pages 3981–4000