کد مقاله | کد نشریه | سال انتشار | مقاله انگلیسی | نسخه تمام متن |
---|---|---|---|---|
4965013 | 1447940 | 2017 | 10 صفحه PDF | دانلود رایگان |
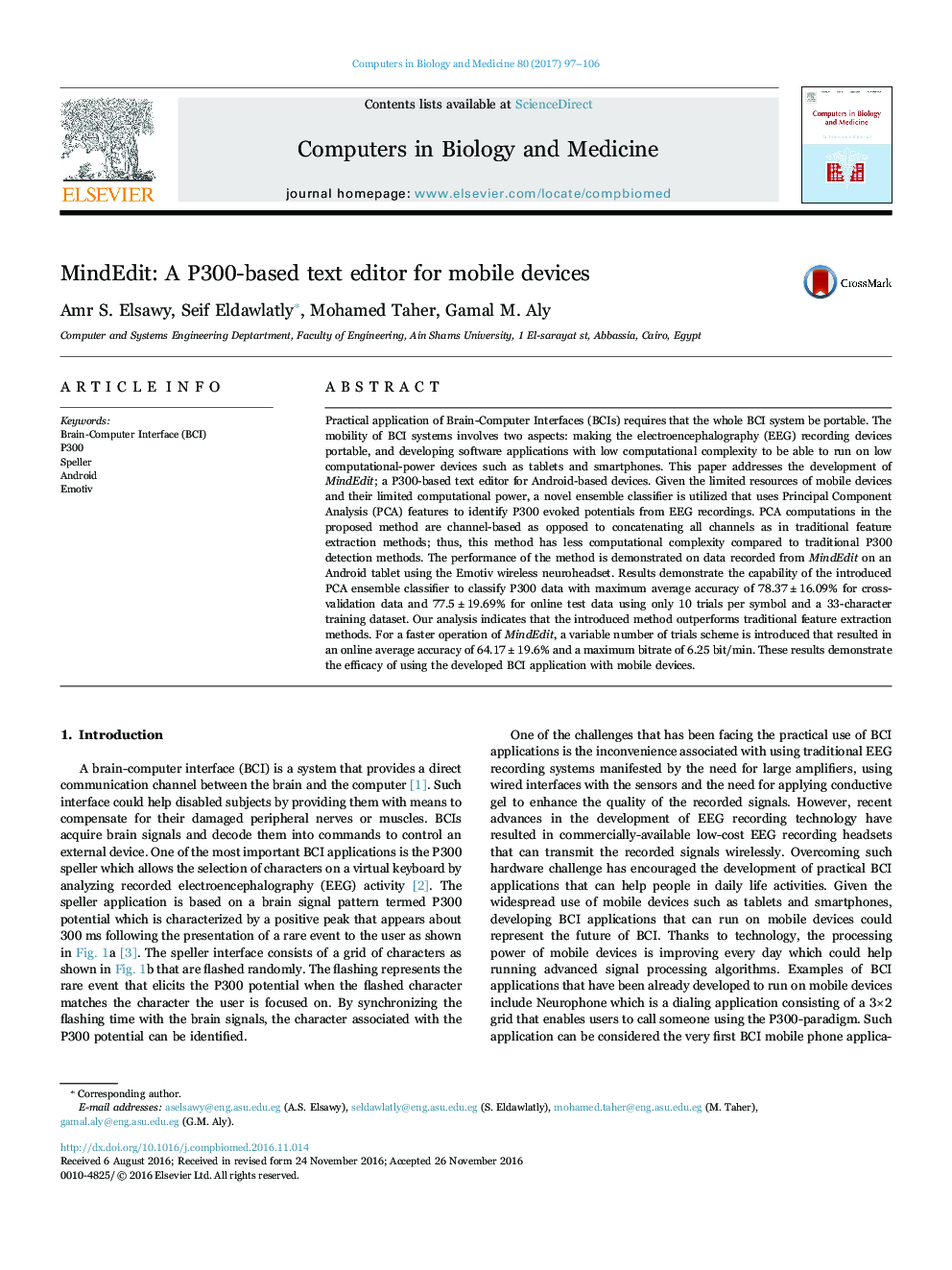
- A P300-based text editor for mobile devices is developed.
- A PCA ensemble classifier is employed to combine multiple channels features.
- A variable number of trials scheme is introduced to enhance the bitrate of MindEdit.
- Proposed ensemble classifier outperformed traditional feature vector approach.
Practical application of Brain-Computer Interfaces (BCIs) requires that the whole BCI system be portable. The mobility of BCI systems involves two aspects: making the electroencephalography (EEG) recording devices portable, and developing software applications with low computational complexity to be able to run on low computational-power devices such as tablets and smartphones. This paper addresses the development of MindEdit; a P300-based text editor for Android-based devices. Given the limited resources of mobile devices and their limited computational power, a novel ensemble classifier is utilized that uses Principal Component Analysis (PCA) features to identify P300 evoked potentials from EEG recordings. PCA computations in the proposed method are channel-based as opposed to concatenating all channels as in traditional feature extraction methods; thus, this method has less computational complexity compared to traditional P300 detection methods. The performance of the method is demonstrated on data recorded from MindEdit on an Android tablet using the Emotiv wireless neuroheadset. Results demonstrate the capability of the introduced PCA ensemble classifier to classify P300 data with maximum average accuracy of 78.37±16.09% for cross-validation data and 77.5±19.69% for online test data using only 10 trials per symbol and a 33-character training dataset. Our analysis indicates that the introduced method outperforms traditional feature extraction methods. For a faster operation of MindEdit, a variable number of trials scheme is introduced that resulted in an online average accuracy of 64.17±19.6% and a maximum bitrate of 6.25 bit/min. These results demonstrate the efficacy of using the developed BCI application with mobile devices.
Journal: Computers in Biology and Medicine - Volume 80, 1 January 2017, Pages 97-106