کد مقاله | کد نشریه | سال انتشار | مقاله انگلیسی | نسخه تمام متن |
---|---|---|---|---|
4969541 | 1449976 | 2017 | 15 صفحه PDF | دانلود رایگان |
عنوان انگلیسی مقاله ISI
Robust-learning fuzzy c-means clustering algorithm with unknown number of clusters
دانلود مقاله + سفارش ترجمه
دانلود مقاله ISI انگلیسی
رایگان برای ایرانیان
کلمات کلیدی
موضوعات مرتبط
مهندسی و علوم پایه
مهندسی کامپیوتر
چشم انداز کامپیوتر و تشخیص الگو
پیش نمایش صفحه اول مقاله
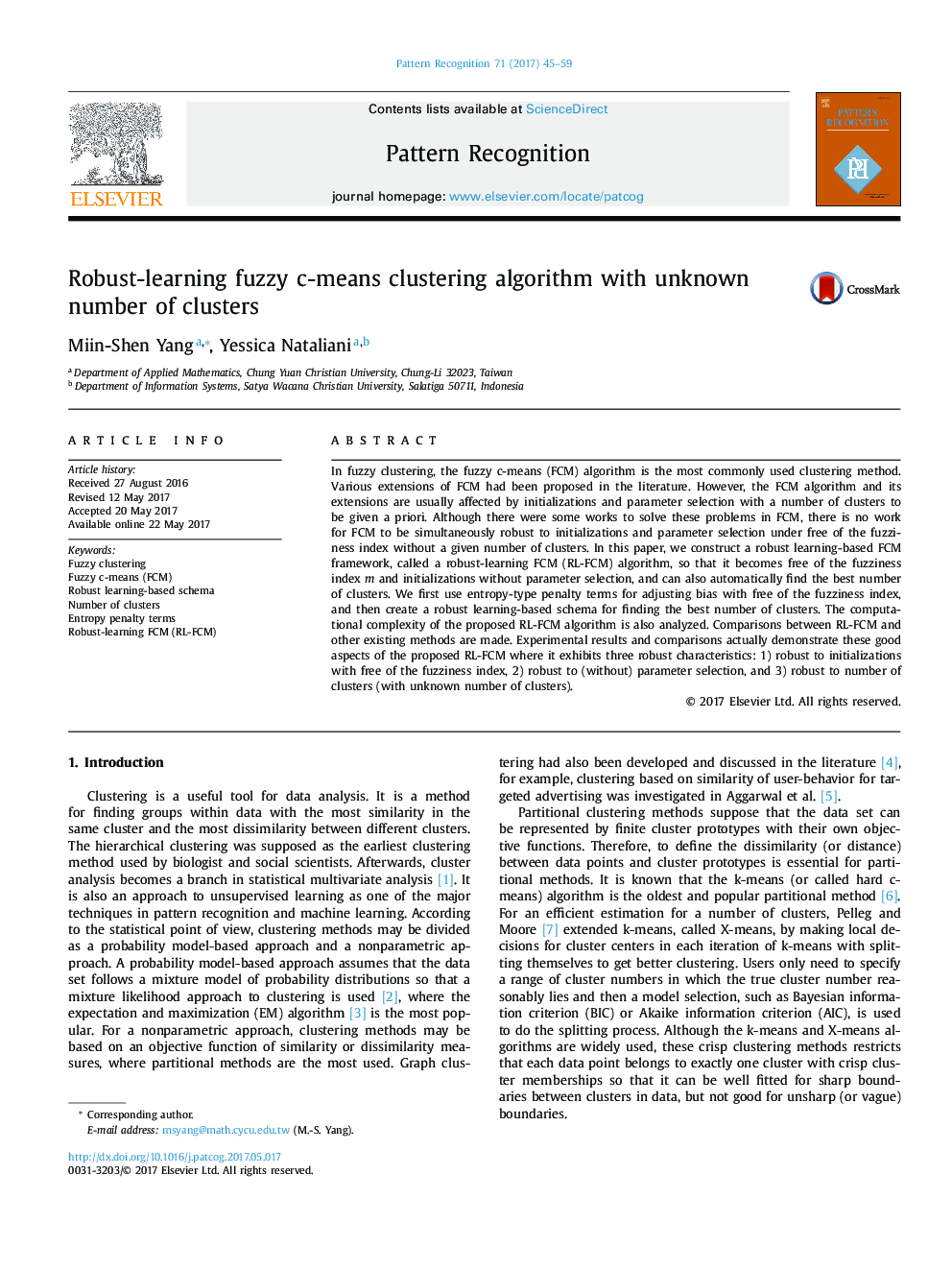
چکیده انگلیسی
In fuzzy clustering, the fuzzy c-means (FCM) algorithm is the most commonly used clustering method. Various extensions of FCM had been proposed in the literature. However, the FCM algorithm and its extensions are usually affected by initializations and parameter selection with a number of clusters to be given a priori. Although there were some works to solve these problems in FCM, there is no work for FCM to be simultaneously robust to initializations and parameter selection under free of the fuzziness index without a given number of clusters. In this paper, we construct a robust learning-based FCM framework, called a robust-learning FCM (RL-FCM) algorithm, so that it becomes free of the fuzziness index m and initializations without parameter selection, and can also automatically find the best number of clusters. We first use entropy-type penalty terms for adjusting bias with free of the fuzziness index, and then create a robust learning-based schema for finding the best number of clusters. The computational complexity of the proposed RL-FCM algorithm is also analyzed. Comparisons between RL-FCM and other existing methods are made. Experimental results and comparisons actually demonstrate these good aspects of the proposed RL-FCM where it exhibits three robust characteristics: 1) robust to initializations with free of the fuzziness index, 2) robust to (without) parameter selection, and 3) robust to number of clusters (with unknown number of clusters).
ناشر
Database: Elsevier - ScienceDirect (ساینس دایرکت)
Journal: Pattern Recognition - Volume 71, November 2017, Pages 45-59
Journal: Pattern Recognition - Volume 71, November 2017, Pages 45-59
نویسندگان
Yang Miin-Shen, Yessica Nataliani,