کد مقاله | کد نشریه | سال انتشار | مقاله انگلیسی | نسخه تمام متن |
---|---|---|---|---|
4969549 | 1449976 | 2017 | 14 صفحه PDF | دانلود رایگان |
عنوان انگلیسی مقاله ISI
LG-CNN: From local parts to global discrimination for fine-grained recognition
دانلود مقاله + سفارش ترجمه
دانلود مقاله ISI انگلیسی
رایگان برای ایرانیان
کلمات کلیدی
موضوعات مرتبط
مهندسی و علوم پایه
مهندسی کامپیوتر
چشم انداز کامپیوتر و تشخیص الگو
پیش نمایش صفحه اول مقاله
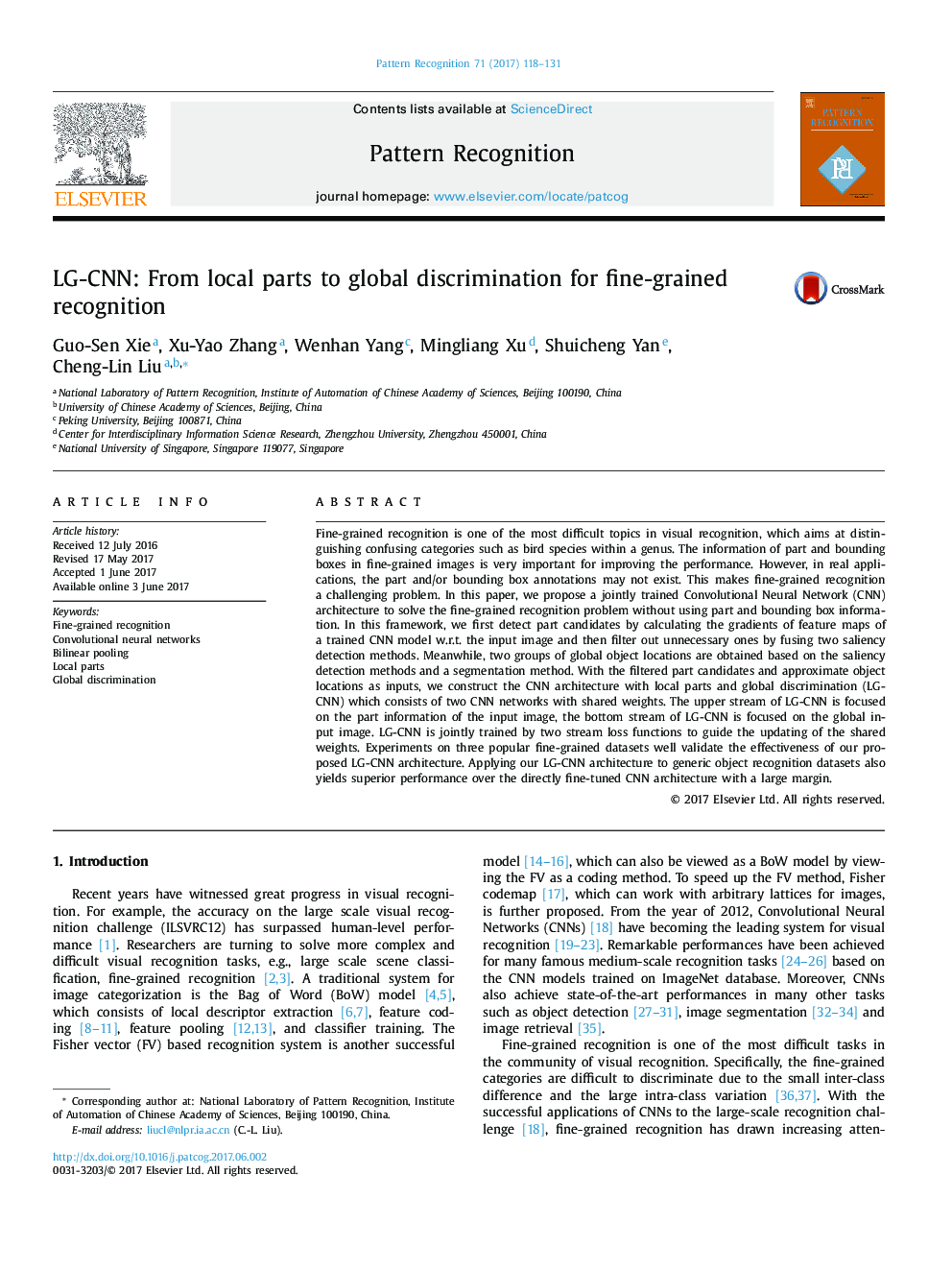
چکیده انگلیسی
Fine-grained recognition is one of the most difficult topics in visual recognition, which aims at distinguishing confusing categories such as bird species within a genus. The information of part and bounding boxes in fine-grained images is very important for improving the performance. However, in real applications, the part and/or bounding box annotations may not exist. This makes fine-grained recognition a challenging problem. In this paper, we propose a jointly trained Convolutional Neural Network (CNN) architecture to solve the fine-grained recognition problem without using part and bounding box information. In this framework, we first detect part candidates by calculating the gradients of feature maps of a trained CNN model w.r.t. the input image and then filter out unnecessary ones by fusing two saliency detection methods. Meanwhile, two groups of global object locations are obtained based on the saliency detection methods and a segmentation method. With the filtered part candidates and approximate object locations as inputs, we construct the CNN architecture with local parts and global discrimination (LG-CNN) which consists of two CNN networks with shared weights. The upper stream of LG-CNN is focused on the part information of the input image, the bottom stream of LG-CNN is focused on the global input image. LG-CNN is jointly trained by two stream loss functions to guide the updating of the shared weights. Experiments on three popular fine-grained datasets well validate the effectiveness of our proposed LG-CNN architecture. Applying our LG-CNN architecture to generic object recognition datasets also yields superior performance over the directly fine-tuned CNN architecture with a large margin.
ناشر
Database: Elsevier - ScienceDirect (ساینس دایرکت)
Journal: Pattern Recognition - Volume 71, November 2017, Pages 118-131
Journal: Pattern Recognition - Volume 71, November 2017, Pages 118-131
نویسندگان
Guo-Sen Xie, Xu-Yao Zhang, Wenhan Yang, Mingliang Xu, Shuicheng Yan, Cheng-Lin Liu,