کد مقاله | کد نشریه | سال انتشار | مقاله انگلیسی | نسخه تمام متن |
---|---|---|---|---|
4969572 | 1449974 | 2018 | 14 صفحه PDF | دانلود رایگان |
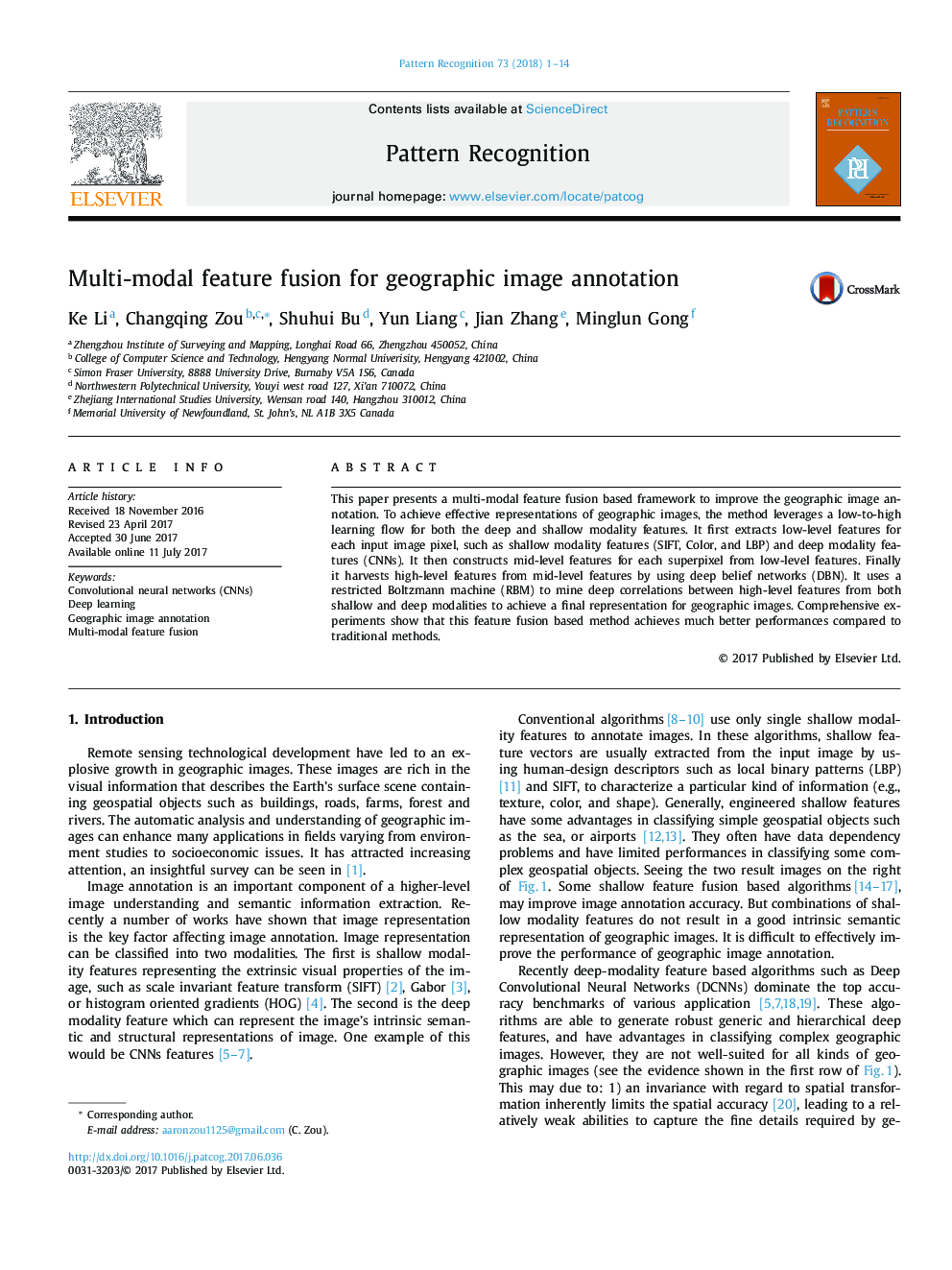
- Multi-modal feature construction: as for the shallow modality features, we propose a mixed shallow feature model which combines Color, LBP, and SIFT features to represent the extrinsic visual properties of geographic images; as for the deep modality features, we design a specialized DCNN to extract the intrinsic semantic information for geographic images.
- Multi-modal feature fusion: we propose a multi-modal feature fusion model based on DBNs and RBM to build a powerful joint representation for geographic images. The model has been shown to be effective to capture both the intrinsic and extrinsic semantic information.
- Open geographic image dataset: we have built a geographic image dataset which contains 300 images (600 â¯Ã⯠600) in six typical areas such as urban, rural, and mountain.
This paper presents a multi-modal feature fusion based framework to improve the geographic image annotation. To achieve effective representations of geographic images, the method leverages a low-to-high learning flow for both the deep and shallow modality features. It first extracts low-level features for each input image pixel, such as shallow modality features (SIFT, Color, and LBP) and deep modality features (CNNs). It then constructs mid-level features for each superpixel from low-level features. Finally it harvests high-level features from mid-level features by using deep belief networks (DBN). It uses a restricted Boltzmann machine (RBM) to mine deep correlations between high-level features from both shallow and deep modalities to achieve a final representation for geographic images. Comprehensive experiments show that this feature fusion based method achieves much better performances compared to traditional methods.
Journal: Pattern Recognition - Volume 73, January 2018, Pages 1-14