کد مقاله | کد نشریه | سال انتشار | مقاله انگلیسی | نسخه تمام متن |
---|---|---|---|---|
4969966 | 1450019 | 2017 | 7 صفحه PDF | دانلود رایگان |
عنوان انگلیسی مقاله ISI
Distance metric learning with the Universum
دانلود مقاله + سفارش ترجمه
دانلود مقاله ISI انگلیسی
رایگان برای ایرانیان
موضوعات مرتبط
مهندسی و علوم پایه
مهندسی کامپیوتر
چشم انداز کامپیوتر و تشخیص الگو
پیش نمایش صفحه اول مقاله
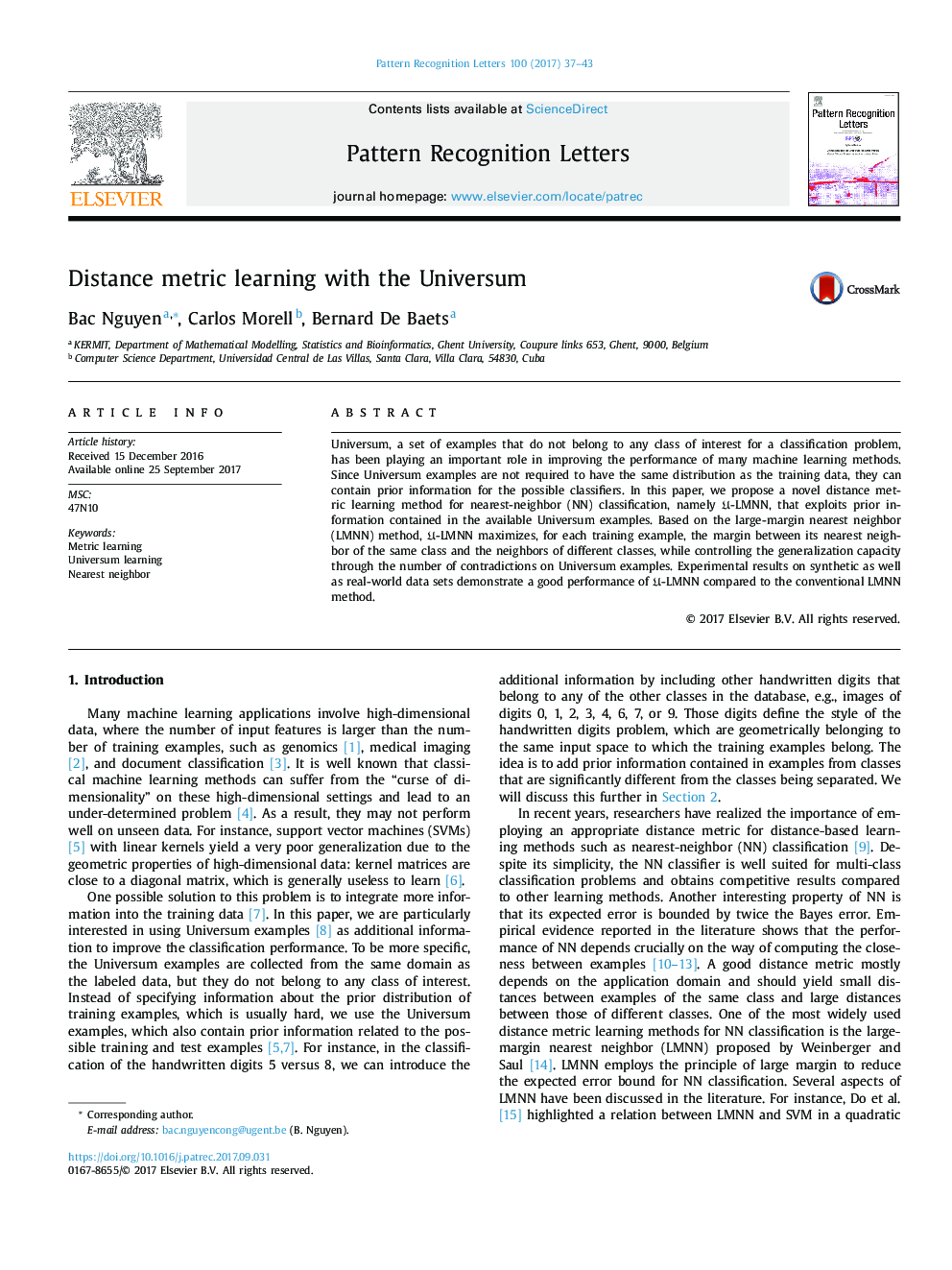
چکیده انگلیسی
Universum, a set of examples that do not belong to any class of interest for a classification problem, has been playing an important role in improving the performance of many machine learning methods. Since Universum examples are not required to have the same distribution as the training data, they can contain prior information for the possible classifiers. In this paper, we propose a novel distance metric learning method for nearest-neighbor (NN) classification, namely U-LMNN, that exploits prior information contained in the available Universum examples. Based on the large-margin nearest neighbor (LMNN) method, U-LMNN maximizes, for each training example, the margin between its nearest neighbor of the same class and the neighbors of different classes, while controlling the generalization capacity through the number of contradictions on Universum examples. Experimental results on synthetic as well as real-world data sets demonstrate a good performance of U-LMNN compared to the conventional LMNN method.
ناشر
Database: Elsevier - ScienceDirect (ساینس دایرکت)
Journal: Pattern Recognition Letters - Volume 100, 1 December 2017, Pages 37-43
Journal: Pattern Recognition Letters - Volume 100, 1 December 2017, Pages 37-43
نویسندگان
Bac Nguyen, Carlos Morell, Bernard De Baets,