کد مقاله | کد نشریه | سال انتشار | مقاله انگلیسی | نسخه تمام متن |
---|---|---|---|---|
4970001 | 1450021 | 2017 | 9 صفحه PDF | دانلود رایگان |
عنوان انگلیسی مقاله ISI
Adaptive spectrum transformation by topology preservation on indefinite proximity data
دانلود مقاله + سفارش ترجمه
دانلود مقاله ISI انگلیسی
رایگان برای ایرانیان
کلمات کلیدی
موضوعات مرتبط
مهندسی و علوم پایه
مهندسی کامپیوتر
چشم انداز کامپیوتر و تشخیص الگو
پیش نمایش صفحه اول مقاله
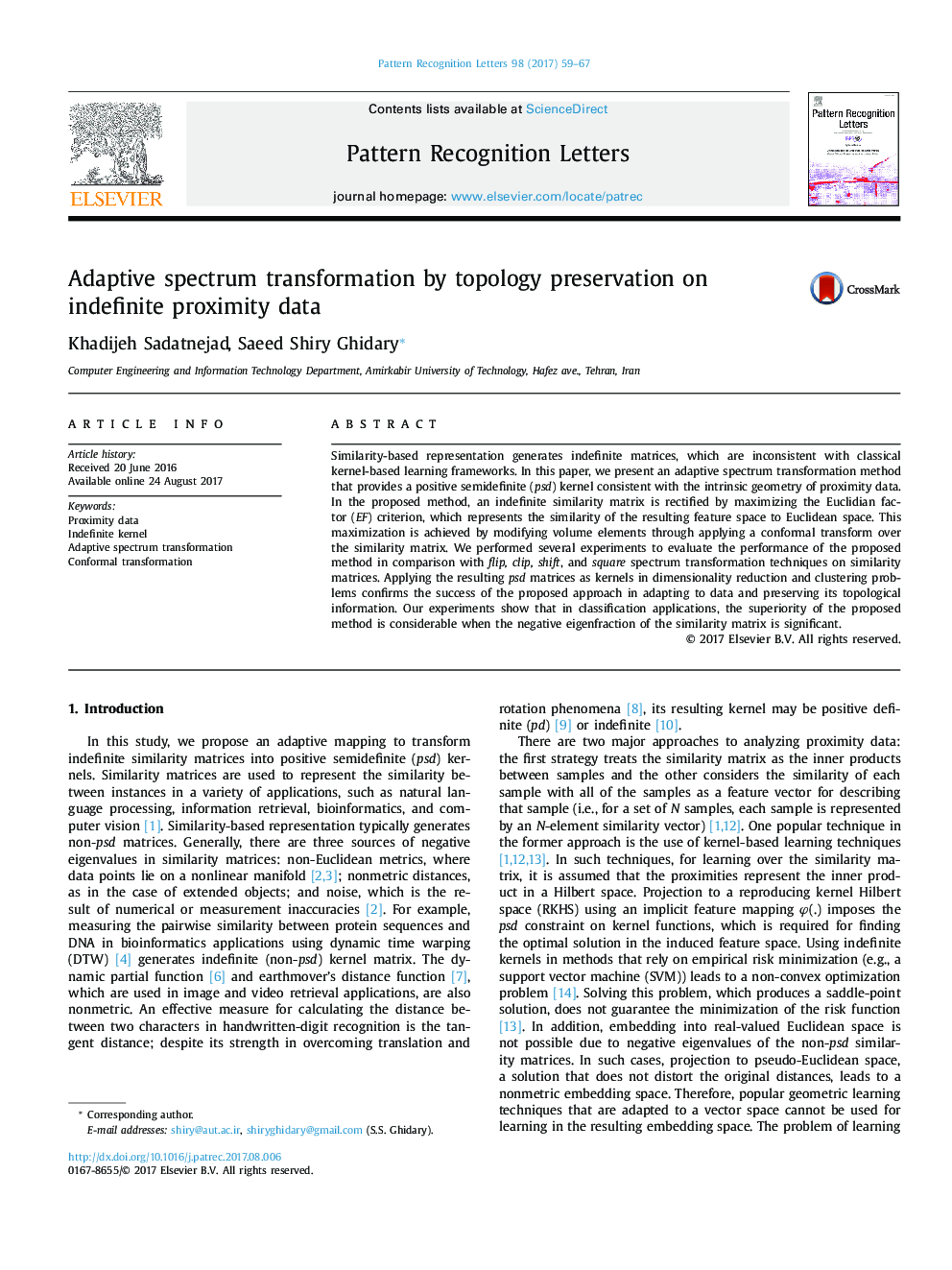
چکیده انگلیسی
Similarity-based representation generates indefinite matrices, which are inconsistent with classical kernel-based learning frameworks. In this paper, we present an adaptive spectrum transformation method that provides a positive semidefinite (psd) kernel consistent with the intrinsic geometry of proximity data. In the proposed method, an indefinite similarity matrix is rectified by maximizing the Euclidian factor (EF) criterion, which represents the similarity of the resulting feature space to Euclidean space. This maximization is achieved by modifying volume elements through applying a conformal transform over the similarity matrix. We performed several experiments to evaluate the performance of the proposed method in comparison with flip, clip, shift, and square spectrum transformation techniques on similarity matrices. Applying the resulting psd matrices as kernels in dimensionality reduction and clustering problems confirms the success of the proposed approach in adapting to data and preserving its topological information. Our experiments show that in classification applications, the superiority of the proposed method is considerable when the negative eigenfraction of the similarity matrix is significant.
ناشر
Database: Elsevier - ScienceDirect (ساینس دایرکت)
Journal: Pattern Recognition Letters - Volume 98, 15 October 2017, Pages 59-67
Journal: Pattern Recognition Letters - Volume 98, 15 October 2017, Pages 59-67
نویسندگان
Khadijeh Sadatnejad, Saeed Shiry Ghidary,