کد مقاله | کد نشریه | سال انتشار | مقاله انگلیسی | نسخه تمام متن |
---|---|---|---|---|
497959 | 862952 | 2014 | 23 صفحه PDF | دانلود رایگان |
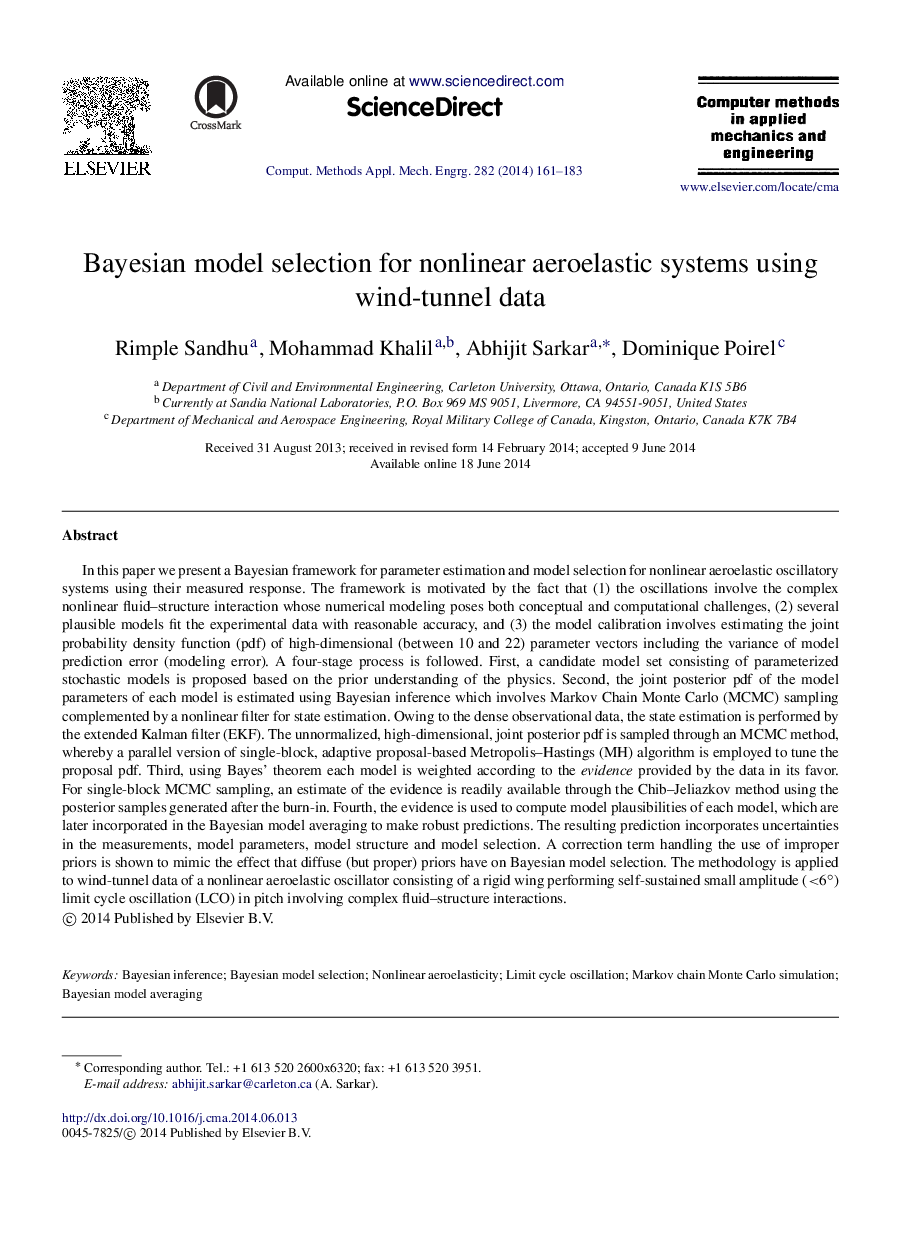
In this paper we present a Bayesian framework for parameter estimation and model selection for nonlinear aeroelastic oscillatory systems using their measured response. The framework is motivated by the fact that (1) the oscillations involve the complex nonlinear fluid–structure interaction whose numerical modeling poses both conceptual and computational challenges, (2) several plausible models fit the experimental data with reasonable accuracy, and (3) the model calibration involves estimating the joint probability density function (pdf) of high-dimensional (between 10 and 22) parameter vectors including the variance of model prediction error (modeling error). A four-stage process is followed. First, a candidate model set consisting of parameterized stochastic models is proposed based on the prior understanding of the physics. Second, the joint posterior pdf of the model parameters of each model is estimated using Bayesian inference which involves Markov Chain Monte Carlo (MCMC) sampling complemented by a nonlinear filter for state estimation. Owing to the dense observational data, the state estimation is performed by the extended Kalman filter (EKF). The unnormalized, high-dimensional, joint posterior pdf is sampled through an MCMC method, whereby a parallel version of single-block, adaptive proposal-based Metropolis–Hastings (MH) algorithm is employed to tune the proposal pdf. Third, using Bayes’ theorem each model is weighted according to the evidence provided by the data in its favor. For single-block MCMC sampling, an estimate of the evidence is readily available through the Chib–Jeliazkov method using the posterior samples generated after the burn-in. Fourth, the evidence is used to compute model plausibilities of each model, which are later incorporated in the Bayesian model averaging to make robust predictions. The resulting prediction incorporates uncertainties in the measurements, model parameters, model structure and model selection. A correction term handling the use of improper priors is shown to mimic the effect that diffuse (but proper) priors have on Bayesian model selection. The methodology is applied to wind-tunnel data of a nonlinear aeroelastic oscillator consisting of a rigid wing performing self-sustained small amplitude (<<6°) limit cycle oscillation (LCO) in pitch involving complex fluid–structure interactions.
Journal: Computer Methods in Applied Mechanics and Engineering - Volume 282, 1 December 2014, Pages 161–183