کد مقاله | کد نشریه | سال انتشار | مقاله انگلیسی | نسخه تمام متن |
---|---|---|---|---|
505381 | 864499 | 2014 | 10 صفحه PDF | دانلود رایگان |
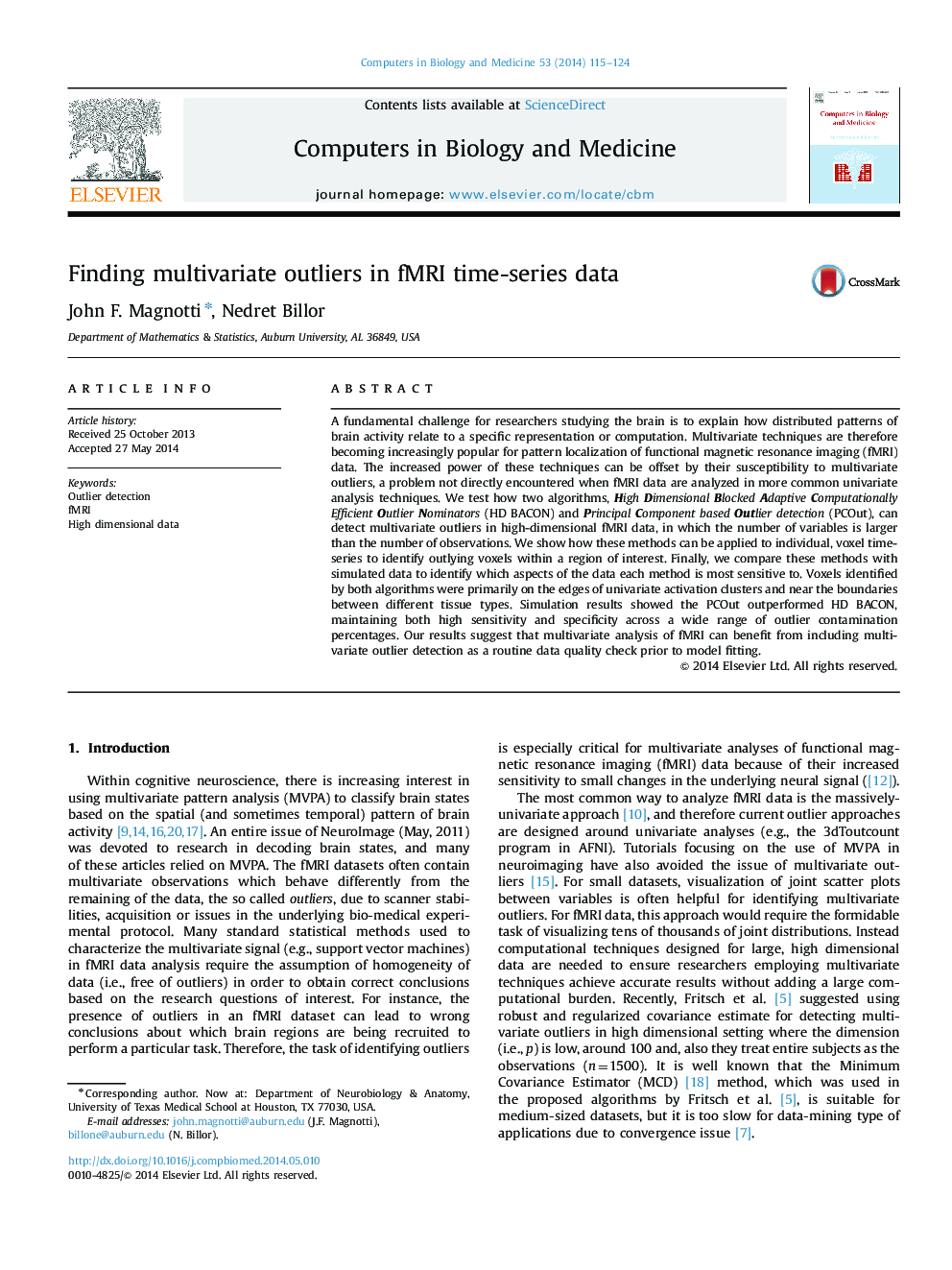
• Multivariate outlier detection methods are applicable to fMRI time-series data.
• Removing outliers increases spatial specificity without hurting classification.
• Simulation shows PCOut is more sensitivity to small outliers than HD BACON.
A fundamental challenge for researchers studying the brain is to explain how distributed patterns of brain activity relate to a specific representation or computation. Multivariate techniques are therefore becoming increasingly popular for pattern localization of functional magnetic resonance imaging (fMRI) data. The increased power of these techniques can be offset by their susceptibility to multivariate outliers, a problem not directly encountered when fMRI data are analyzed in more common univariate analysis techniques. We test how two algorithms, High Dimensional Blocked Adaptive Computationally Efficient Outlier Nominators (HD BACON) and Principal Component based Outlier detection (PCOut), can detect multivariate outliers in high-dimensional fMRI data, in which the number of variables is larger than the number of observations. We show how these methods can be applied to individual, voxel time-series to identify outlying voxels within a region of interest. Finally, we compare these methods with simulated data to identify which aspects of the data each method is most sensitive to. Voxels identified by both algorithms were primarily on the edges of univariate activation clusters and near the boundaries between different tissue types. Simulation results showed the PCOut outperformed HD BACON, maintaining both high sensitivity and specificity across a wide range of outlier contamination percentages. Our results suggest that multivariate analysis of fMRI can benefit from including multivariate outlier detection as a routine data quality check prior to model fitting.
Journal: Computers in Biology and Medicine - Volume 53, 1 October 2014, Pages 115–124