کد مقاله | کد نشریه | سال انتشار | مقاله انگلیسی | نسخه تمام متن |
---|---|---|---|---|
5081951 | 1477625 | 2009 | 9 صفحه PDF | دانلود رایگان |
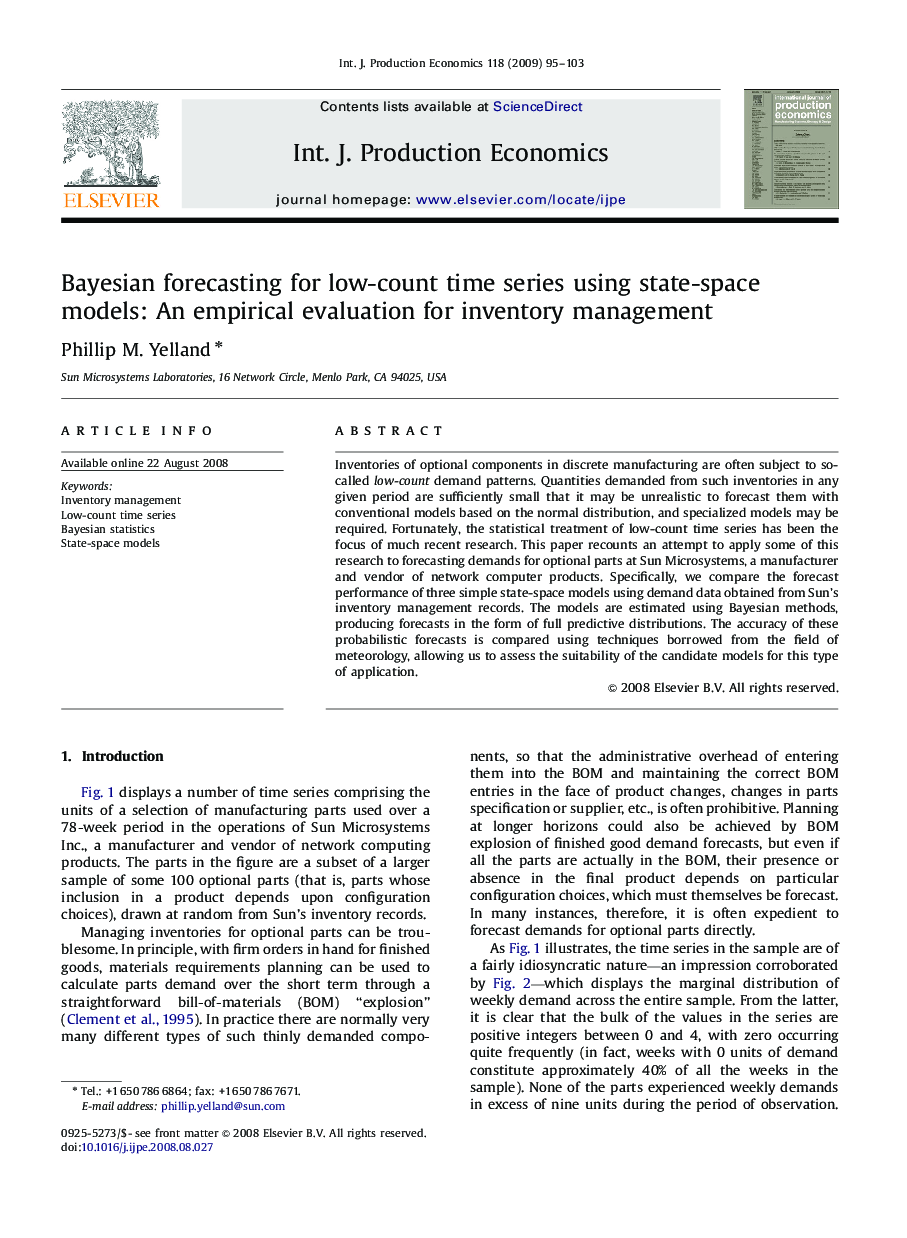
Inventories of optional components in discrete manufacturing are often subject to so-called low-count demand patterns. Quantities demanded from such inventories in any given period are sufficiently small that it may be unrealistic to forecast them with conventional models based on the normal distribution, and specialized models may be required. Fortunately, the statistical treatment of low-count time series has been the focus of much recent research. This paper recounts an attempt to apply some of this research to forecasting demands for optional parts at Sun Microsystems, a manufacturer and vendor of network computer products. Specifically, we compare the forecast performance of three simple state-space models using demand data obtained from Sun's inventory management records. The models are estimated using Bayesian methods, producing forecasts in the form of full predictive distributions. The accuracy of these probabilistic forecasts is compared using techniques borrowed from the field of meteorology, allowing us to assess the suitability of the candidate models for this type of application.
Journal: International Journal of Production Economics - Volume 118, Issue 1, March 2009, Pages 95-103